The CAT SET on the MAT: Cross Attention for Set Matching in Bipartite Hypergraphs
arxiv(2021)
摘要
Usual relations between entities could be captured using graphs; but those of a higher-order -- more so between two different types of entities (which we term "left" and "right") -- calls for a "bipartite hypergraph". For example, given a left set of symptoms and right set of diseases, the relation between a set subset of symptoms (that a patient experiences at a given point of time) and a subset of diseases (that he/she might be diagnosed with) could be well-represented using a bipartite hyperedge. The state-of-the-art in embedding nodes of a hypergraph is based on learning the self-attention structure between node-pairs from a hyperedge. In the present work, given a bipartite hypergraph, we aim at capturing relations between node pairs from the cross-product between the left and right hyperedges, and term it a "cross-attention" (CAT) based model. More precisely, we pose "bipartite hyperedge link prediction" as a set-matching (SETMAT) problem and propose a novel neural network architecture called CATSETMAT for the same. We perform extensive experiments on multiple bipartite hypergraph datasets to show the superior performance of CATSETMAT, which we compare with multiple techniques from the state-of-the-art. Our results also elucidate information flow in self- and cross-attention scenarios.
更多查看译文
关键词
bipartite hypergraphs,set matching,cross attention,cat set
AI 理解论文
溯源树
样例
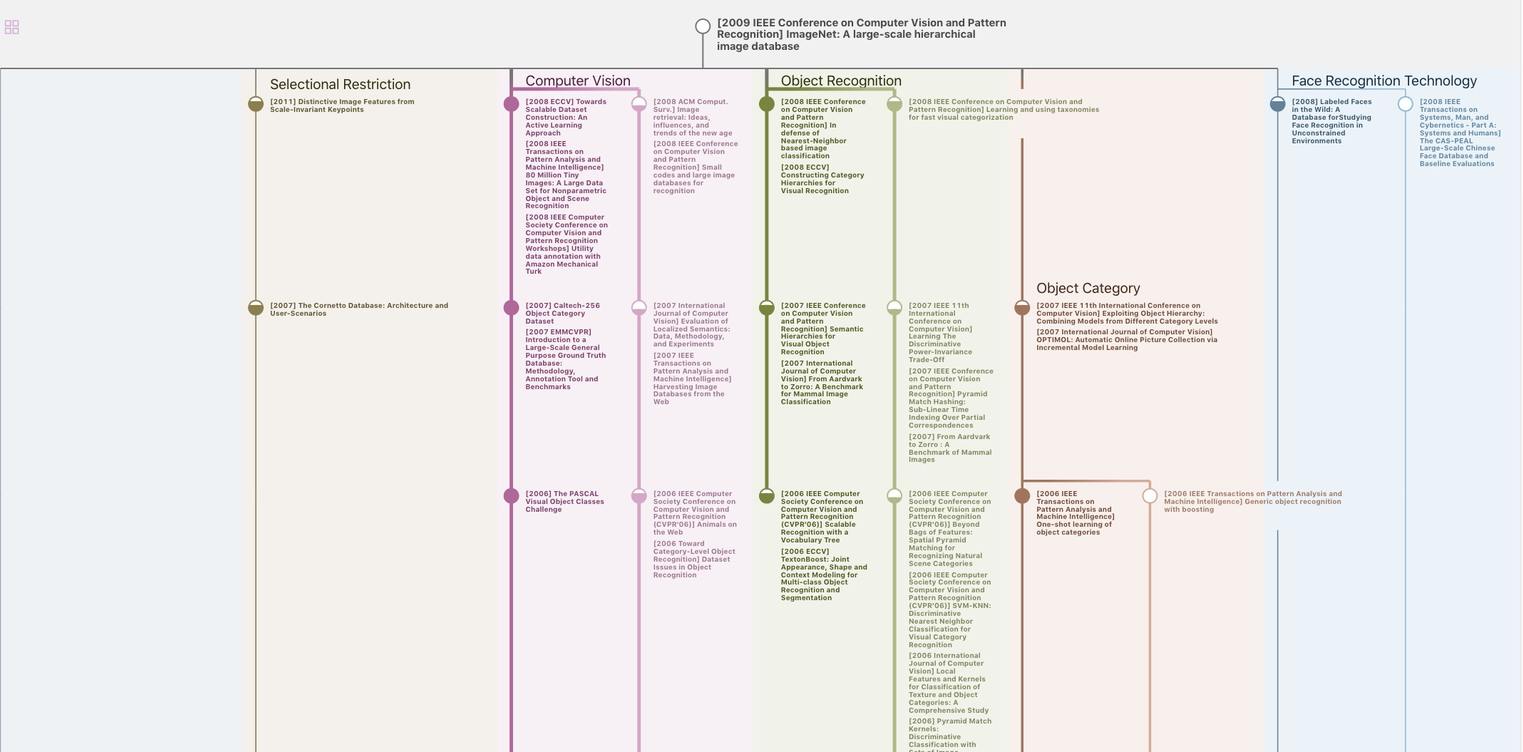
生成溯源树,研究论文发展脉络
Chat Paper
正在生成论文摘要