A seq2seq Neural Network based Conversational Agent for Gulf Arabic Dialect
2020 21st International Arab Conference on Information Technology (ACIT)(2020)
摘要
A Conversational Agent (CA), or dialogue system, is a computer system that has the ability to respond to humans automatically using natural language. CAs offer instant responses and can concurrently assist a potentially unlimited number of users. The modeling of CAs in Arabic has so far received less attention when compared with other languages due to the complexity of the Arabic language, the existence of several dialects, and a lack of data resources. The literature contends that modeling a CA in Arabic mostly done using pattern-matching and information retrieval, employing classification approaches with a closed-domain data source. There is extremely limited research so far on modeling an open-domain CA in the Arabic dialect. This research has utilized a deep-learning architecture, known as the Seq2Seq neural network, to build a CA in the Arabic Gulf dialect. We formulated the CA problem as a machine translation problem and, therefore, built our corpus from tweets, in the post-reply format, to train and evaluate the model. We investigated the effects of pretrained embeddings on the performance of the CA. For our evaluation, a Bilingual Evaluation Understudy (BLEU) score and human evaluators were used. The performance of the model was found to be comparable with existing deep learning models that have been trained on much larger corpora and in other languages. Our results present a promising first step towards building an open-domain CA in the Gulf Arabic dialect.
更多查看译文
关键词
Chatbots,Deep Learning,Conversational Agent,Neural Machine Translation,Transformer
AI 理解论文
溯源树
样例
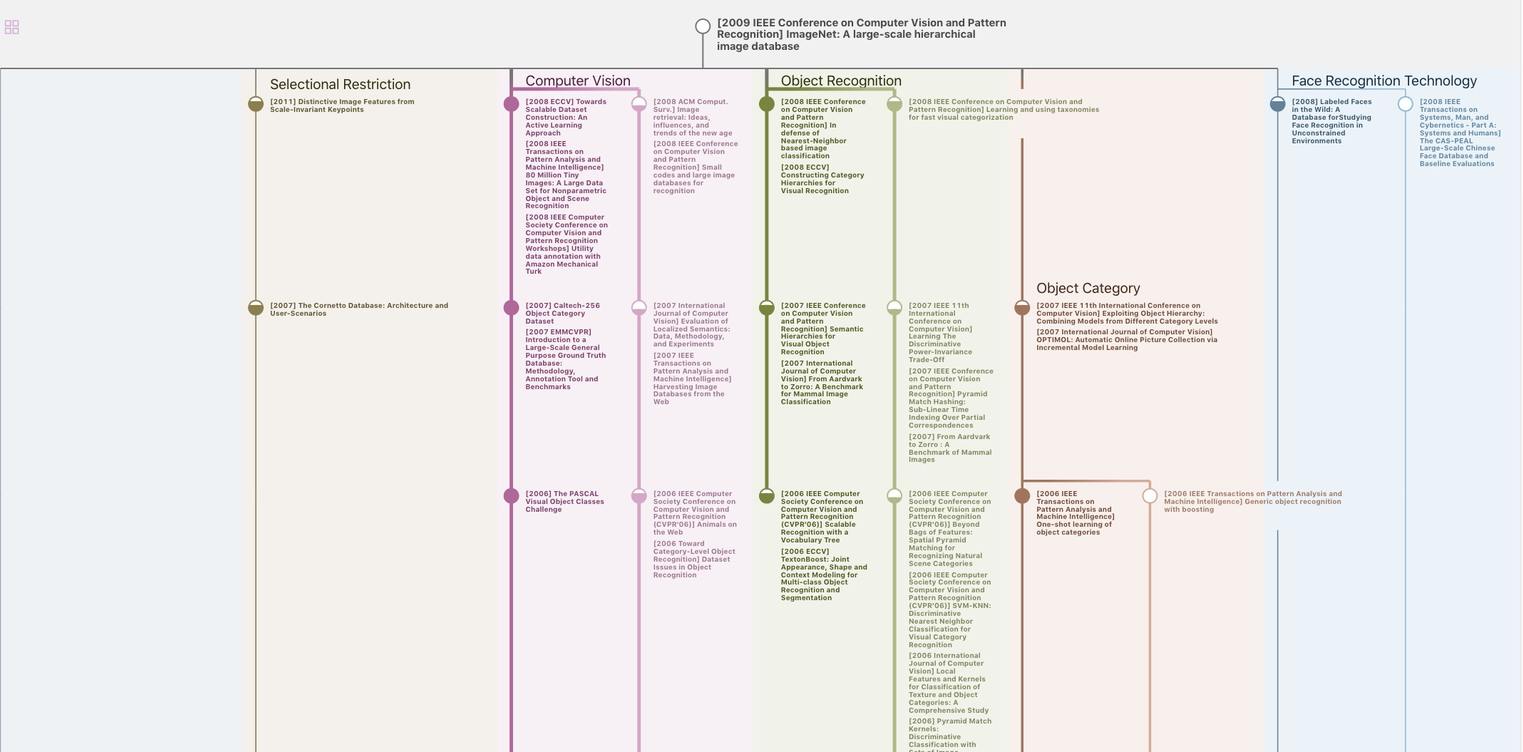
生成溯源树,研究论文发展脉络
Chat Paper
正在生成论文摘要