GCNIllustrator: Illustrating the Effect of Hyperparameters on Graph Convolutional Networks
International Multimedia Conference(2021)
摘要
ABSTRACTAn increasing number of real-world applications are using graph-structured datasets, imposing challenges to existing machine learning algorithms. Graph Convolutional Networks (GCNs) are deep learning models, specifically designed to operate on graphs. One of the most tedious steps in training GCNs is the choice of the hyperparameters, especially since they exhibit unique properties compared to other neural models. Not only machine learning beginners, but also experienced practitioners often have difficulties to properly tune their models. We hypothesize that having a tool that visualizes the effect of hyperparameters choice on the performance can accelerate the model development and improve the understanding of these black-box models. Additionally, observing clusters of certain nodes helps to empirically understand how a given prediction was made due to the feature propagation step of GCNs. Therefore, this demo introduces GCNIllustrator - a web-based visual analytics tool for illustrating the effect of hyperparameters on the predictions in a citations graph.
更多查看译文
AI 理解论文
溯源树
样例
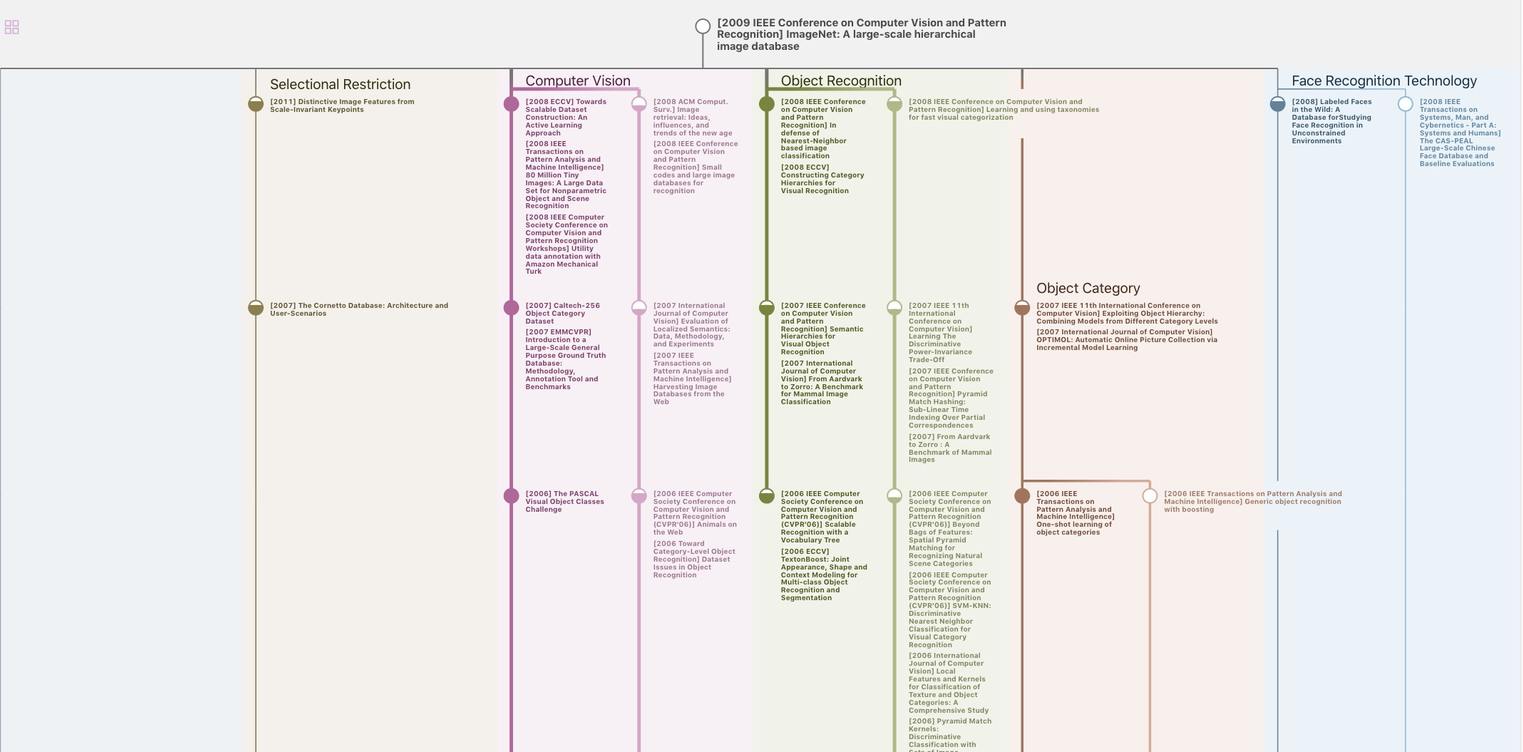
生成溯源树,研究论文发展脉络
Chat Paper
正在生成论文摘要