Partial Discharge Detection Based on Long Short-Term Memory Neural Network Classifier with Efficient Feature Extraction Methods
user-61442502e55422cecdaf6898(2021)
摘要
Faults occur in electric transmission and distribution lines may accompany with a destructive phenomenon named partial discharge. If left untreated for an extended period of time, the insulation would be damaged. Technical University of Ostrava and ENET Centre utilize a cost effective, simple meter to measure the stray electric field voltage signals for covered conductors of medium voltage overhead power lines. The data could be utilized to detect faults through continuously, and economically monitoring power lines. In recent years, various unique, and complicated methods have been proposed to detect the partial discharge in the aforementioned signals. Most of them are in need of huge amount of computing and equipment support. This article is dedicated to building an effective long short-term memory neural network classifier and reducing its requirements on hardware conditions, such as storage memory, CPU, and GPU, through efficient data processing and feature extraction. The proposed method is tested on the ENET Centre’s public voltage signal data. And it is compared to various classifiers. It demonstrates light calculation, superior performance, and great practicality. Such an automated monitoring method would reduce the maintenance costs of power systems. And it would efficiently prevents power outages.
更多查看译文
关键词
partial discharge detection,long short-term memory neural network,feature extraction method
AI 理解论文
溯源树
样例
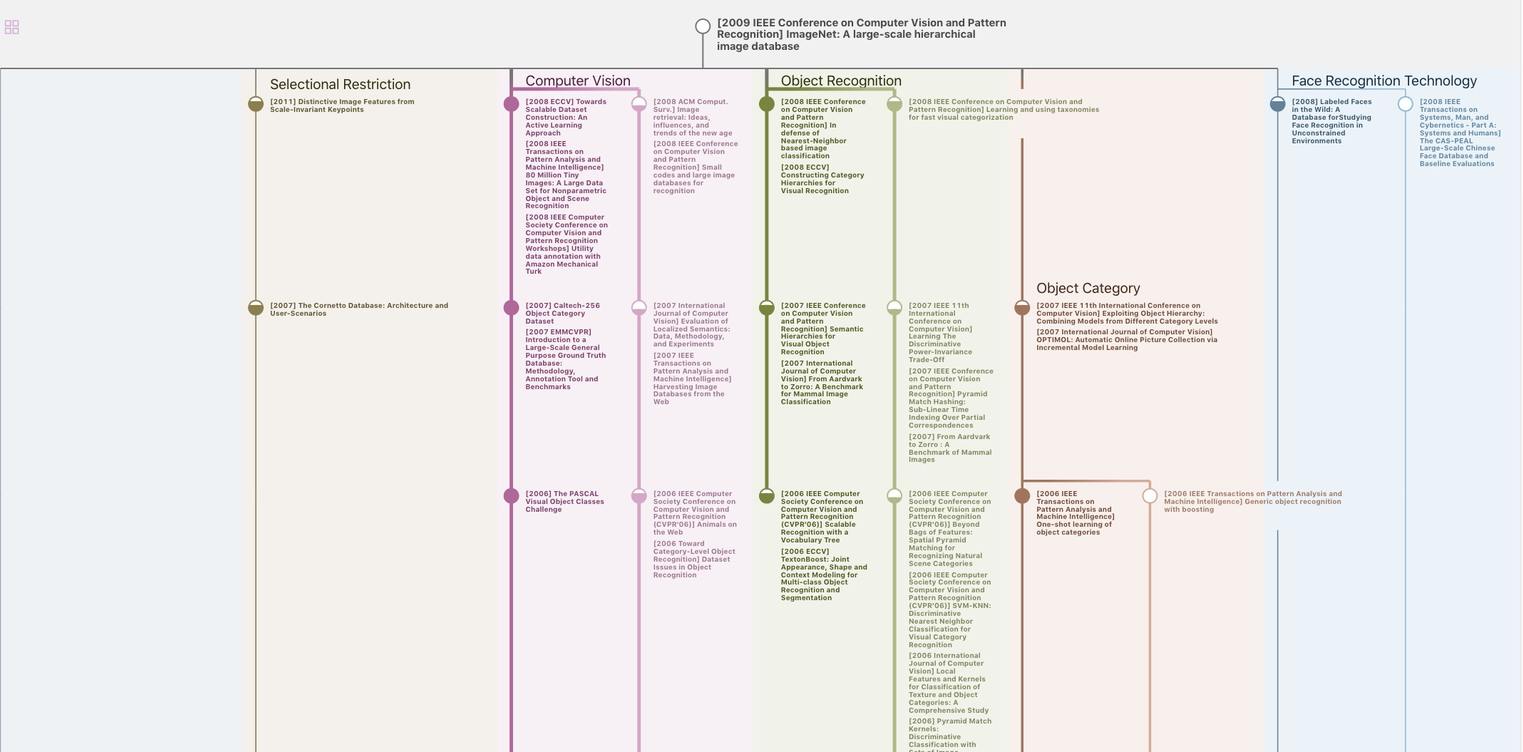
生成溯源树,研究论文发展脉络
Chat Paper
正在生成论文摘要