Improving the Robustness to Variations of Objects and Instructions with a Neuro-Symbolic Approach for Interactive Instruction Following
arxiv(2022)
摘要
An interactive instruction following task has been proposed as a benchmark for learning to map natural language instructions and first-person vision into sequences of actions to interact with objects in 3D environments. We found that an existing end-to-end neural model for this task tends to fail to interact with objects of unseen attributes and follow various instructions. We assume that this problem is caused by the high sensitivity of neural feature extraction to small changes in vision and language inputs. To mitigate this problem, we propose a neuro-symbolic approach that utilizes high-level symbolic features, which are robust to small changes in raw inputs, as intermediate representations. We verify the effectiveness of our model with the subtask evaluation on the ALFRED benchmark. Our experiments show that our approach significantly outperforms the end-to-end neural model by 9, 46, and 74 points in the success rate on the ToggleObject, PickupObject, and SliceObject subtasks in unseen environments respectively.
更多查看译文
关键词
interactive instructions,variations,neuro-symbolic
AI 理解论文
溯源树
样例
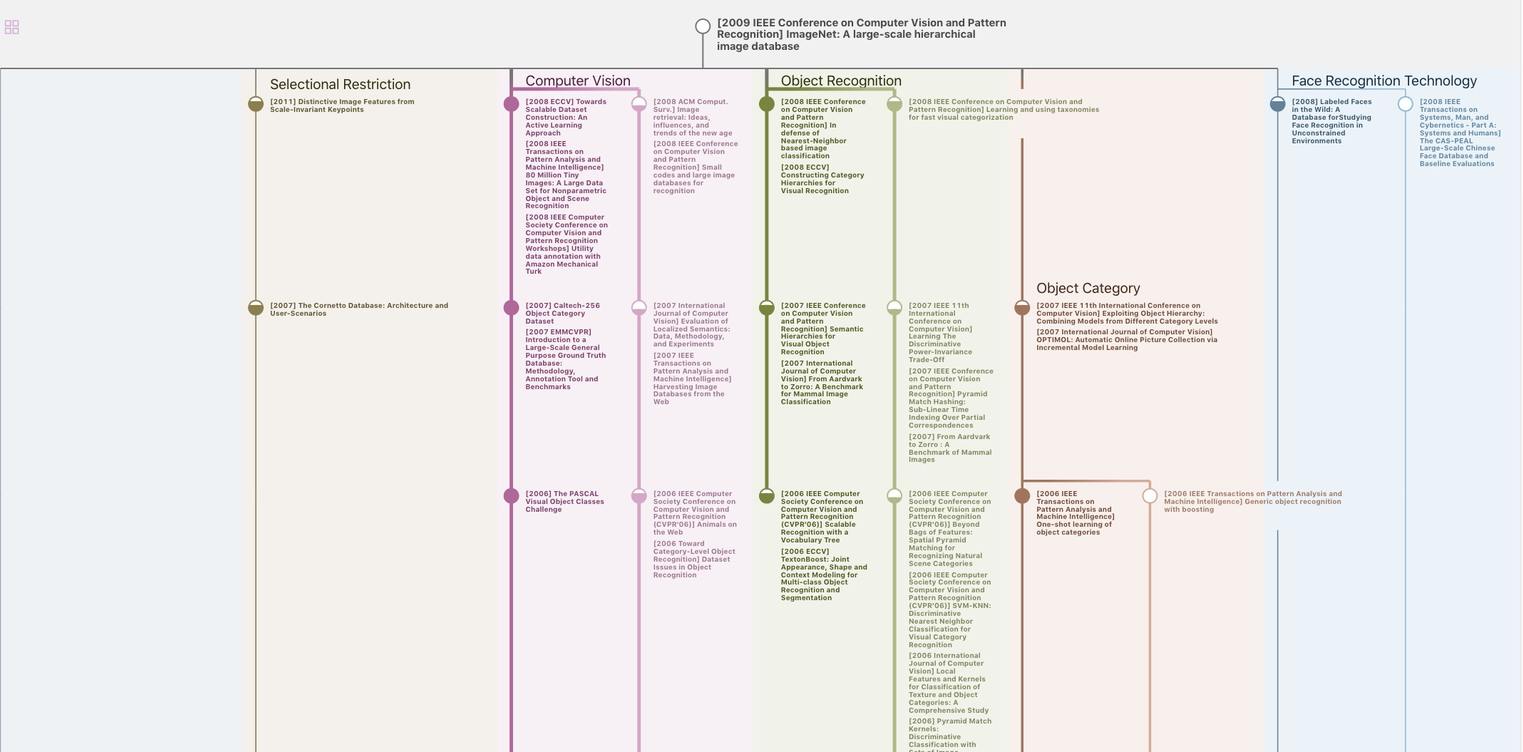
生成溯源树,研究论文发展脉络
Chat Paper
正在生成论文摘要