Simple Attention Module Based Speaker Verification with Iterative Noisy Label Detection.
IEEE International Conference on Acoustics, Speech, and Signal Processing (ICASSP)(2022)
摘要
Recently, the attention mechanism such as squeeze-and-excitation module (SE) and convolutional block attention module (CBAM) has achieved great success in deep learning-based speaker verification system. This paper introduces an alternative effective yet simple one, i.e., simple attention module (SimAM), for speaker verification. The SimAM module is a plug-and-play module without extra modal parameters. In addition, we propose a noisy label detection method to iteratively filter out the data samples with a noisy label from the training data, considering that a large-scale dataset labeled with human annotation or other automated processes may contain noisy labels. Data with the noisy label may over parameterize a deep neural network (DNN) and result in a performance drop due to the memorization effect of the DNN. Experiments are conducted on VoxCeleb dataset. The speaker verification model with SimAM achieves the 0.675% equal error rate (EER) on VoxCeleb1 original test trials. Our proposed iterative noisy label detection method further reduces the EER to 0.643%.
更多查看译文
关键词
Speaker verification,attention module,noisy label
AI 理解论文
溯源树
样例
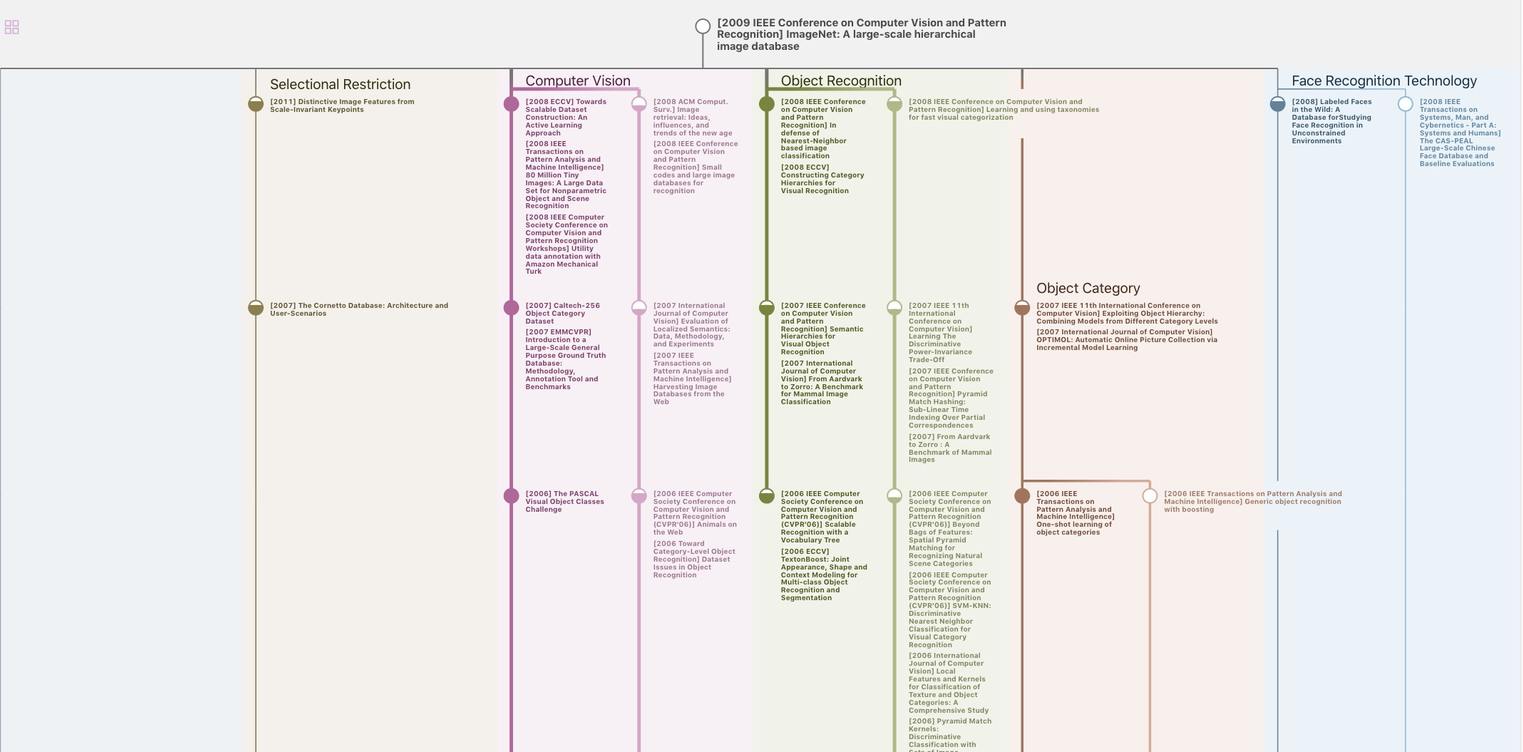
生成溯源树,研究论文发展脉络
Chat Paper
正在生成论文摘要