Domain Generalization via Domain-based Covariance Minimization
arXiv (Cornell University)(2021)
Abstract
Researchers have been facing a difficult problem that data generation mechanisms could be influenced by internal or external factors leading to the training and test data with quite different distributions, consequently traditional classification or regression from the training set is unable to achieve satisfying results on test data. In this paper, we address this nontrivial domain generalization problem by finding a central subspace in which domain-based covariance is minimized while the functional relationship is simultaneously maximally preserved. We propose a novel variance measurement for multiple domains so as to minimize the difference between conditional distributions across domains with solid theoretical demonstration and supports, meanwhile, the algorithm preserves the functional relationship via maximizing the variance of conditional expectations given output. Furthermore, we also provide a fast implementation that requires much less computation and smaller memory for large-scale matrix operations, suitable for not only domain generalization but also other kernel-based eigenvalue decompositions. To show the practicality of the proposed method, we compare our methods against some well-known dimension reduction and domain generalization techniques on both synthetic data and real-world applications. We show that for small-scale datasets, we are able to achieve better quantitative results indicating better generalization performance over unseen test datasets. For large-scale problems, the proposed fast implementation maintains the quantitative performance but at a substantially lower computational cost.
MoreTranslated text
Key words
covariance minimization,generalization,domain-based
AI Read Science
Must-Reading Tree
Example
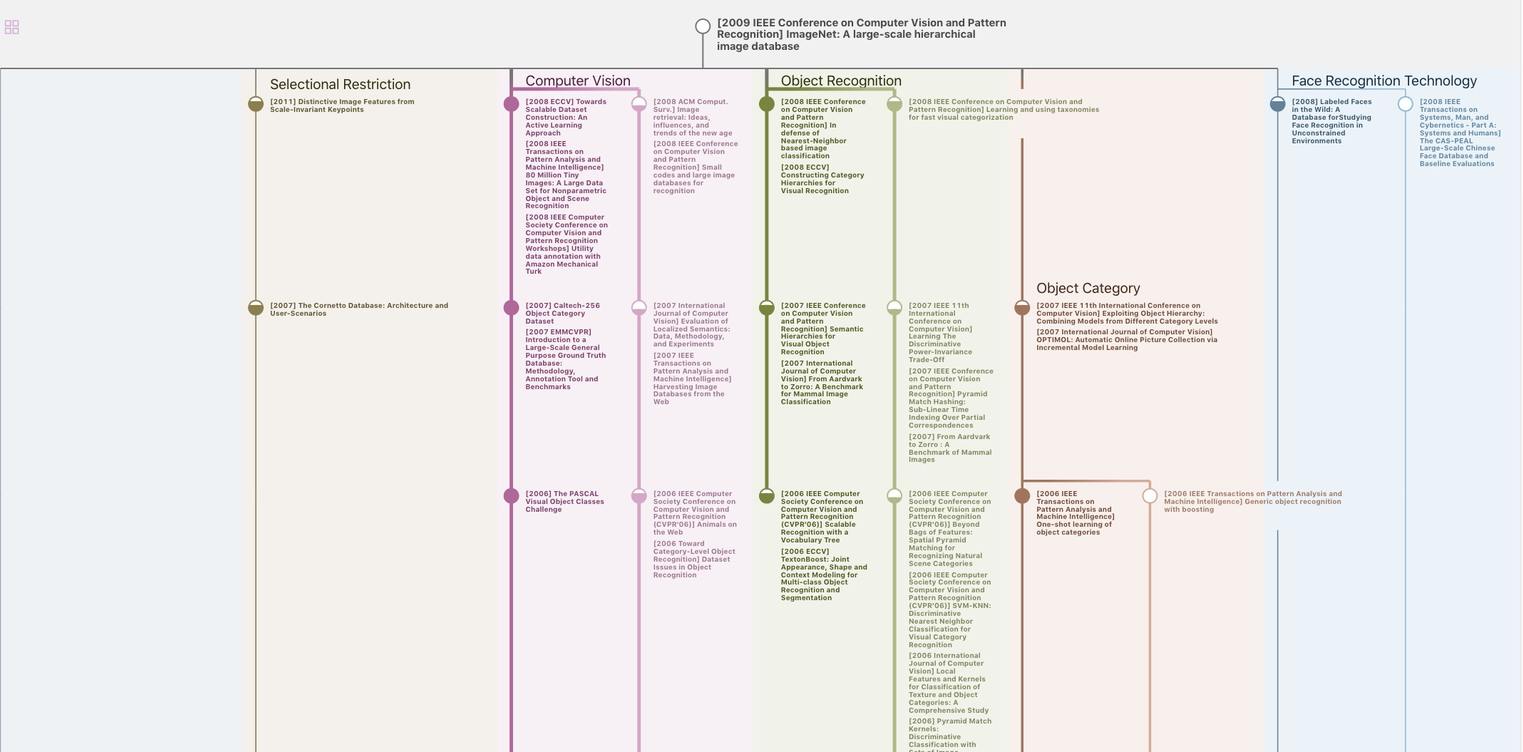
Generate MRT to find the research sequence of this paper
Chat Paper
Summary is being generated by the instructions you defined