Fine-Grained Adversarial Semi-Supervised Learning
ACM Transactions on Multimedia Computing, Communications, and Applications(2022)
摘要
Editorial NotesThe authors have requested minor, non-substantive changes to the VoR and, in accordance with ACM policies, a Corrected Version of Record was published on March 18, 2022. For reference purposes, the VoR may still be accessed via the Supplemental Material section on this citation page.AbstractIn this article, we exploit Semi-Supervised Learning (SSL) to increase the amount of training data to improve the performance of Fine-Grained Visual Categorization (FGVC). This problem has not been investigated in the past in spite of prohibitive annotation costs that FGVC requires. Our approach leverages unlabeled data with an adversarial optimization strategy in which the internal features representation is obtained with a second-order pooling model. This combination allows one to back-propagate the information of the parts, represented by second-order pooling, onto unlabeled data in an adversarial training setting. We demonstrate the effectiveness of the combined use by conducting experiments on six state-of-the-art fine-grained datasets, which include Aircrafts, Stanford Cars, CUB-200-2011, Oxford Flowers, Stanford Dogs, and the recent Semi-Supervised iNaturalist-Aves. Experimental results clearly show that our proposed method has better performance than the only previous approach that examined this problem; it also obtained higher classification accuracy with respect to the supervised learning methods with which we compared.
更多查看译文
关键词
Fine-grained visual categorization, deep neural networks, semi-supervised learning, adversarial learning
AI 理解论文
溯源树
样例
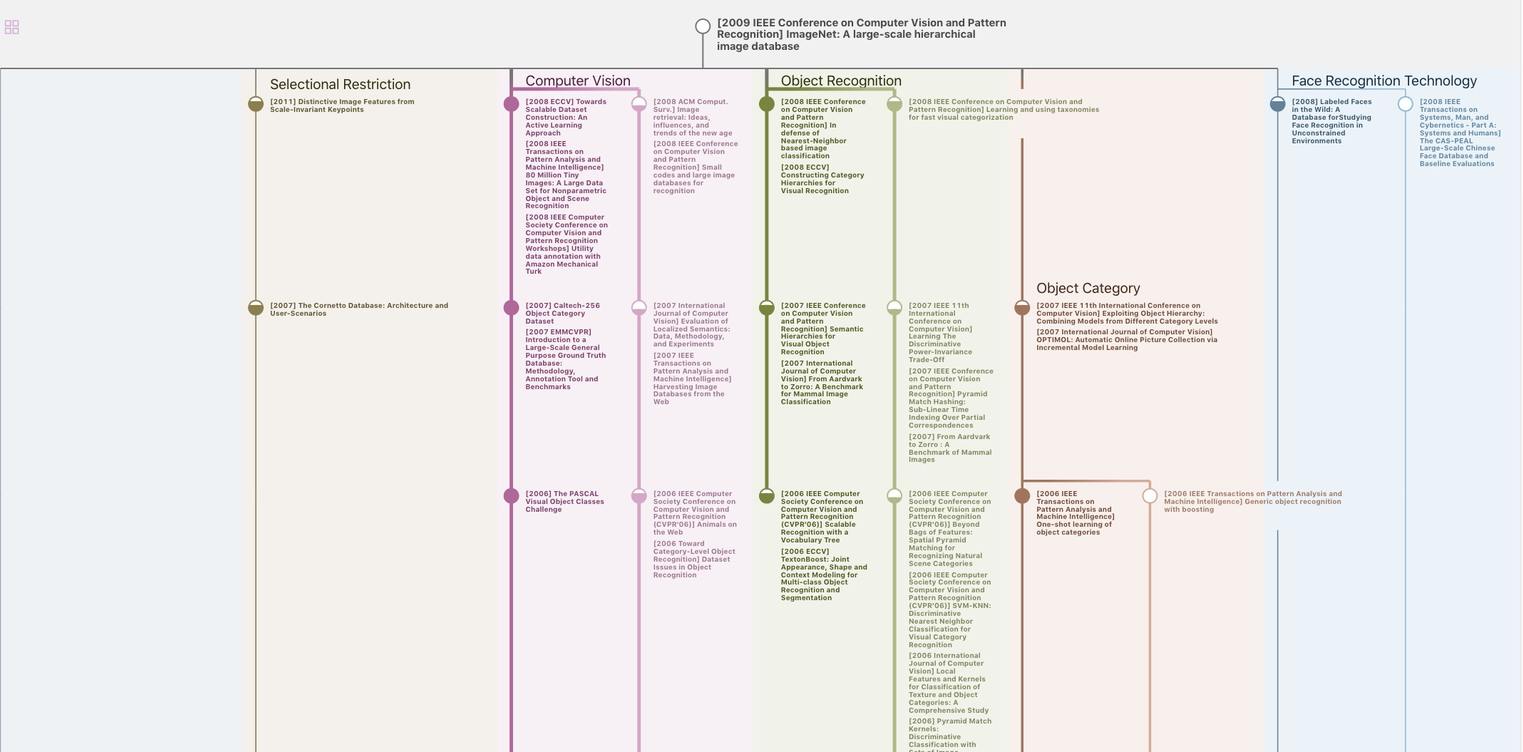
生成溯源树,研究论文发展脉络
Chat Paper
正在生成论文摘要