Rare Events via Cross-Entropy Population Monte Carlo
IEEE Signal Processing Letters(2022)
摘要
Rare events are events that happen with very low frequency. Estimating rare event probabilities using Monte Carlo techniques is computationally expensive, often to the point of intractability, and special methods are required. Importance sampling (IS) is a well known technique that uses a proposal distribution in place of a target distribution to lower the variance of the estimator. Key to the success of IS methods is the choice of a proposal distribution, or the parameters governing the distribution. Adaptive importance sampling improves the parameters of a family or population of proposal distributions iteratively through trials. We present a novel cross-entropy population Monte Carlo algorithm, which adapts the parameters of proposals through the cross-entropy method. The proposed method stands apart from previous work in that we are not optimizing a mixture distribution. Instead, we leverage deterministic mixture weights and optimize the distributions individually through a reinterpretation of the typical derivation of the cross-entropy method. Demonstrations on rare event examples show that the algorithm can outperform existing resampling based population Monte Carlo methods, especially for higher-dimensional problems. We also demonstrate efficacy on a conjunction analysis problem.
更多查看译文
关键词
Adaptive importance sampling,cross-entropy,rare events
AI 理解论文
溯源树
样例
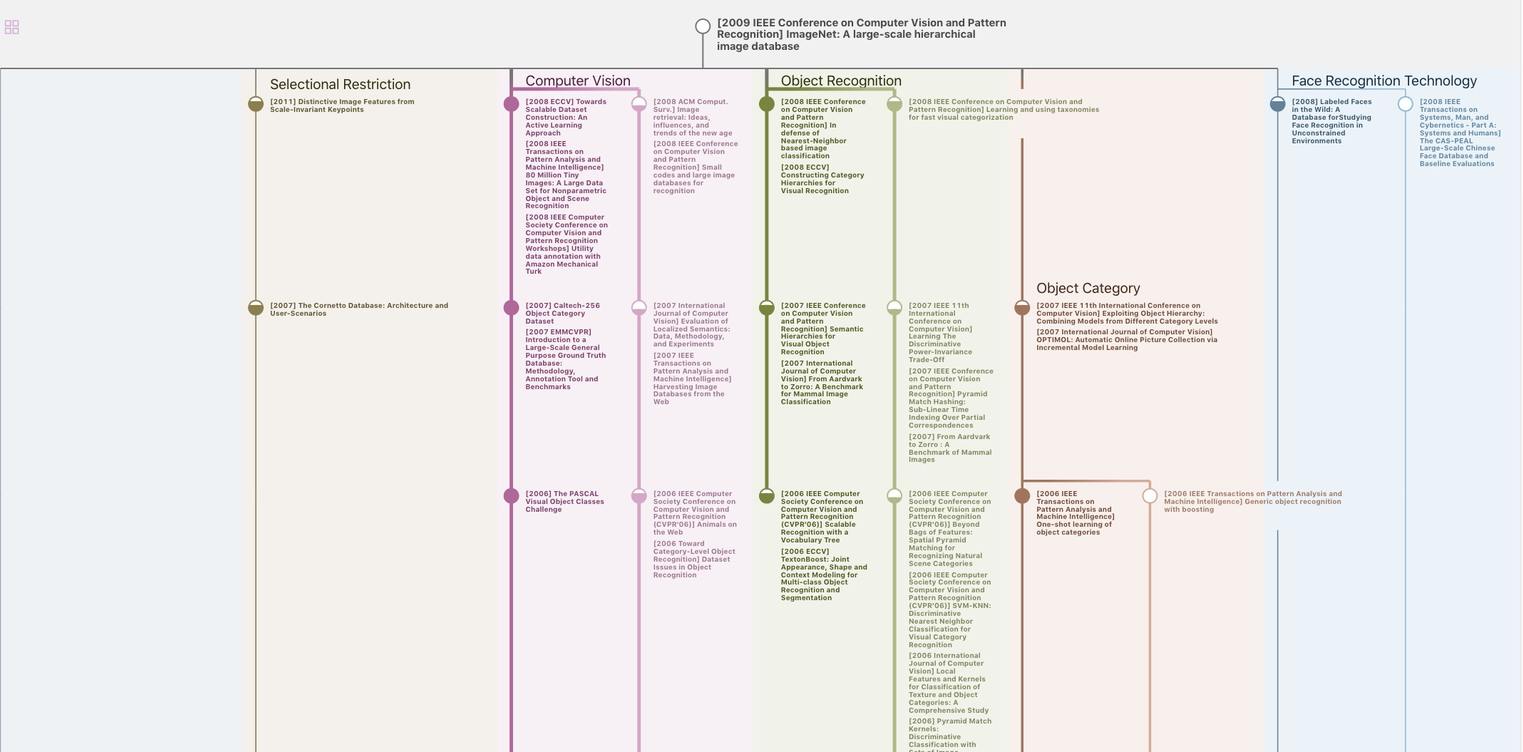
生成溯源树,研究论文发展脉络
Chat Paper
正在生成论文摘要