Self-supervised Learning is More Robust to Dataset Imbalance
International Conference on Learning Representations (ICLR)(2021)
摘要
Self-supervised learning (SSL) is a scalable way to learn general visual representations since it learns without labels. However, large-scale unlabeled datasets in the wild often have long-tailed label distributions, where we know little about the behavior of SSL. In this work, we systematically investigate self-supervised learning under dataset imbalance. First, we find out via extensive experiments that off-the-shelf self-supervised representations are already more robust to class imbalance than supervised representations. The performance gap between balanced and imbalanced pre-training with SSL is significantly smaller than the gap with supervised learning, across sample sizes, for both in-domain and, especially, out-of-domain evaluation. Second, towards understanding the robustness of SSL, we hypothesize that SSL learns richer features from frequent data: it may learn label-irrelevant-but-transferable features that help classify the rare classes and downstream tasks. In contrast, supervised learning has no incentive to learn features irrelevant to the labels from frequent examples. We validate this hypothesis with semi-synthetic experiments and theoretical analyses on a simplified setting. Third, inspired by the theoretical insights, we devise a re-weighted regularization technique that consistently improves the SSL representation quality on imbalanced datasets with several evaluation criteria, closing the small gap between balanced and imbalanced datasets with the same number of examples.
更多查看译文
关键词
self-supervised learning,dataset imbalance,representation learning,long-tailed recognition
AI 理解论文
溯源树
样例
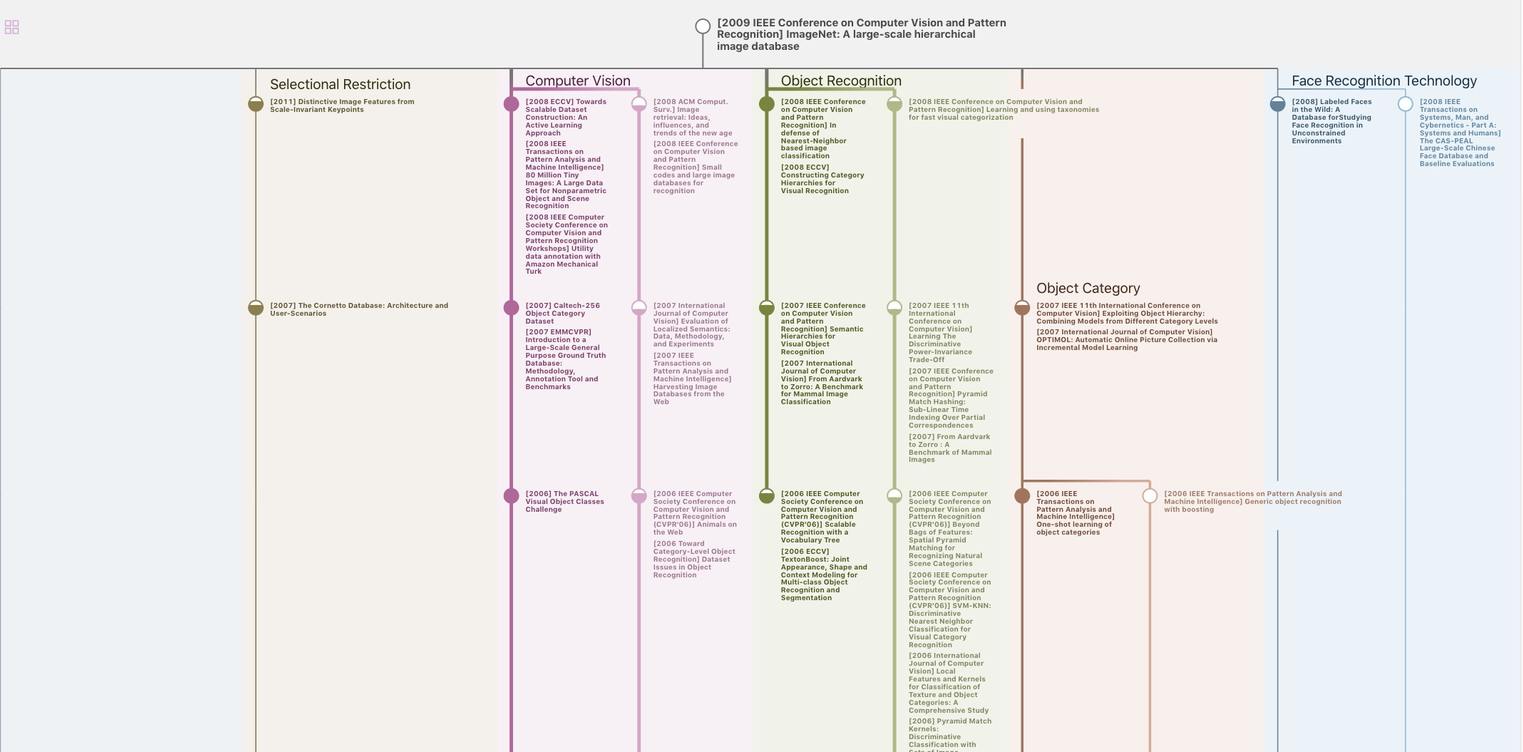
生成溯源树,研究论文发展脉络
Chat Paper
正在生成论文摘要