CLIP-Adapter: Better Vision-Language Models with Feature Adapters
International Journal of Computer Vision(2024)
摘要
Large-scale contrastive vision-language pretraining has shown significant progress in visual representation learning. Unlike traditional visual systems trained by a fixed set of discrete labels, a new paradigm was introduced in Radford et al. (International conference on machine learning, PMLR, 2021) to directly learn to align images with raw texts in an open-vocabulary setting. On downstream tasks, a carefully chosen text prompt is employed to make zero-shot predictions. To avoid non-trivial prompt engineering, context optimization (Zhou et al. in Int J Comput Vis 130(9):2337–2348, 2022) has been proposed to learn continuous vectors as task-specific prompts with few-shot training examples. In this paper, we show that there is an alternative path to achieve better vision-language models other than prompt tuning. While prompt tuning is for the textual inputs, we propose CLIP-Adapter to conduct fine-tuning with feature adapters on either visual or language branch. Specifically, CLIP-Adapter adopts an additional bottleneck layer to learn new features and performs residual-style feature blending with the original pretrained features. As a consequence, CLIP-Adapter is able to outperform context optimization while maintaining a simple design. Experiments and extensive ablation studies on various visual classification tasks demonstrate the effectiveness of our approach.
更多查看译文
关键词
Feature adapter,Vision-language model,Few-shot learning,Open-vocabulary
AI 理解论文
溯源树
样例
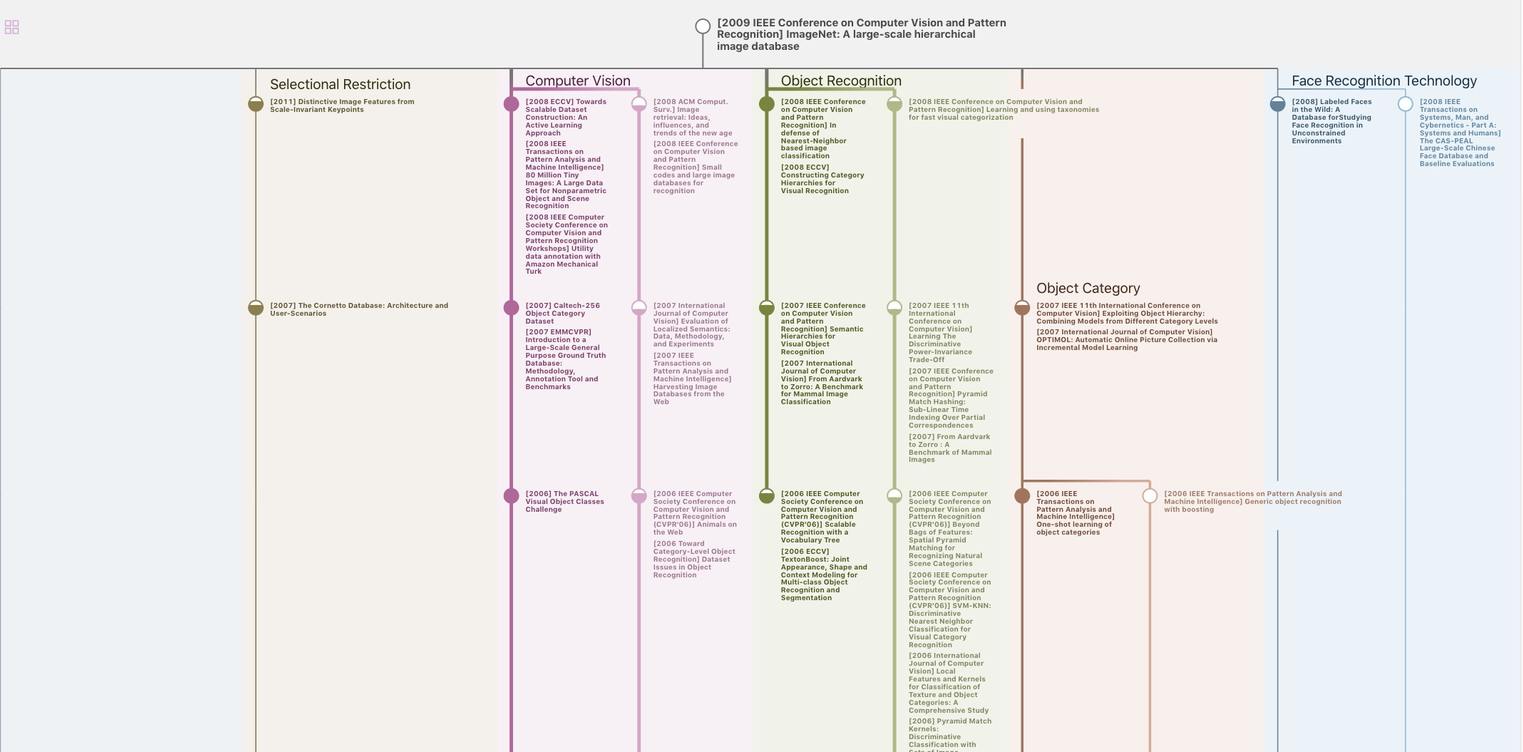
生成溯源树,研究论文发展脉络
Chat Paper
正在生成论文摘要