Exploring Heterogeneous Characteristics of Layers in ASR Models for More Efficient Training.
IEEE International Conference on Acoustics, Speech, and Signal Processing (ICASSP)(2022)
摘要
Transformer-based architectures have been the subject of research aimed at understanding their overparameterization and the non-uniform importance of their layers. Applying these approaches to Automatic Speech Recognition, we demonstrate that the state-of-the-art Conformer models generally have multiple ambient layers. We study the stability of these layers across runs and model sizes, propose that group normalization may be used without disrupting their formation, and examine their correlation with model weight updates in each layer. Finally, we apply these findings to Federated Learning in order to improve the training procedure, by targeting Federated Dropout to layers by importance. This allows us to reduce the model size optimized by clients without quality degradation, and shows potential for future exploration.
更多查看译文
关键词
speech recognition,federated learning,ambient layers,deep learning
AI 理解论文
溯源树
样例
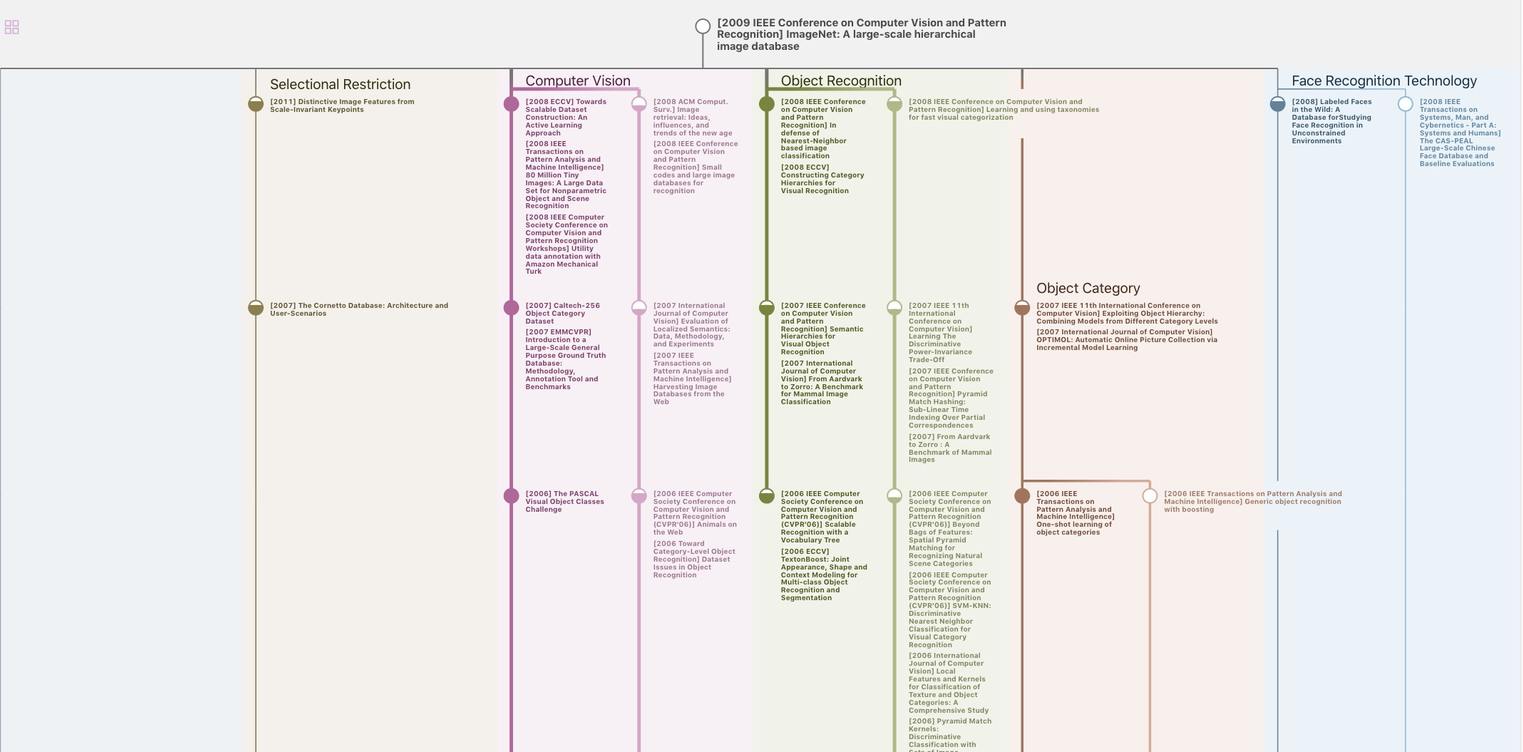
生成溯源树,研究论文发展脉络
Chat Paper
正在生成论文摘要