Multi-timescale and multi-centrality layered node selection for efficient traffic monitoring in SDNs
Computer Networks(2021)
摘要
With the assistance of global view in software-defined networks (SDNs), some switch nodes can be selected for traffic monitoring, which ensures the security of SDNs with a minimum cost. However, identifying the flow-based smallest subset of switches with the maximum coverage for node selection is always an NP-hard problem. To address this challenge, a two-stage parameter-rectifying-based selection (TSPRS) scheme is presented for efficient traffic monitoring in SDNs. In this novel scheme, a multi-timescale and multi-centrality layered virtual network (MMLVN) model is first built according to the different network structures over various time periods. By virtue of the MMLVN model, a tensor-based eigenvector multi-centrality computing (TEMC) method is employed at the first-stage selection to identify the smallest subset of switches with the maximum coverage for obtaining the subset of primary core nodes. To obtain more accurate parameters of real-time flows, a modified MMLVN model is further built by combining the results of a bandwidth cost-effective polling (BCeP) algorithm and the first-stage selection. Then, based on the modified MMLVN model, the TEMC method is reused to obtain the final optimization subset of the core nodes for efficient traffic monitoring at the second-stage selection. Extensive simulation experiments demonstrate that the proposed scheme can achieve a desirable tradeoff between the monitoring overhead and accuracy, and also reduce the communication bandwidth cost, which is superior to the existing state-of-the-art schemes.
更多查看译文
关键词
Software-defined networks,Eigenvector multi-centrality,Traffic monitoring,Node-selection,Cost-effectiveness
AI 理解论文
溯源树
样例
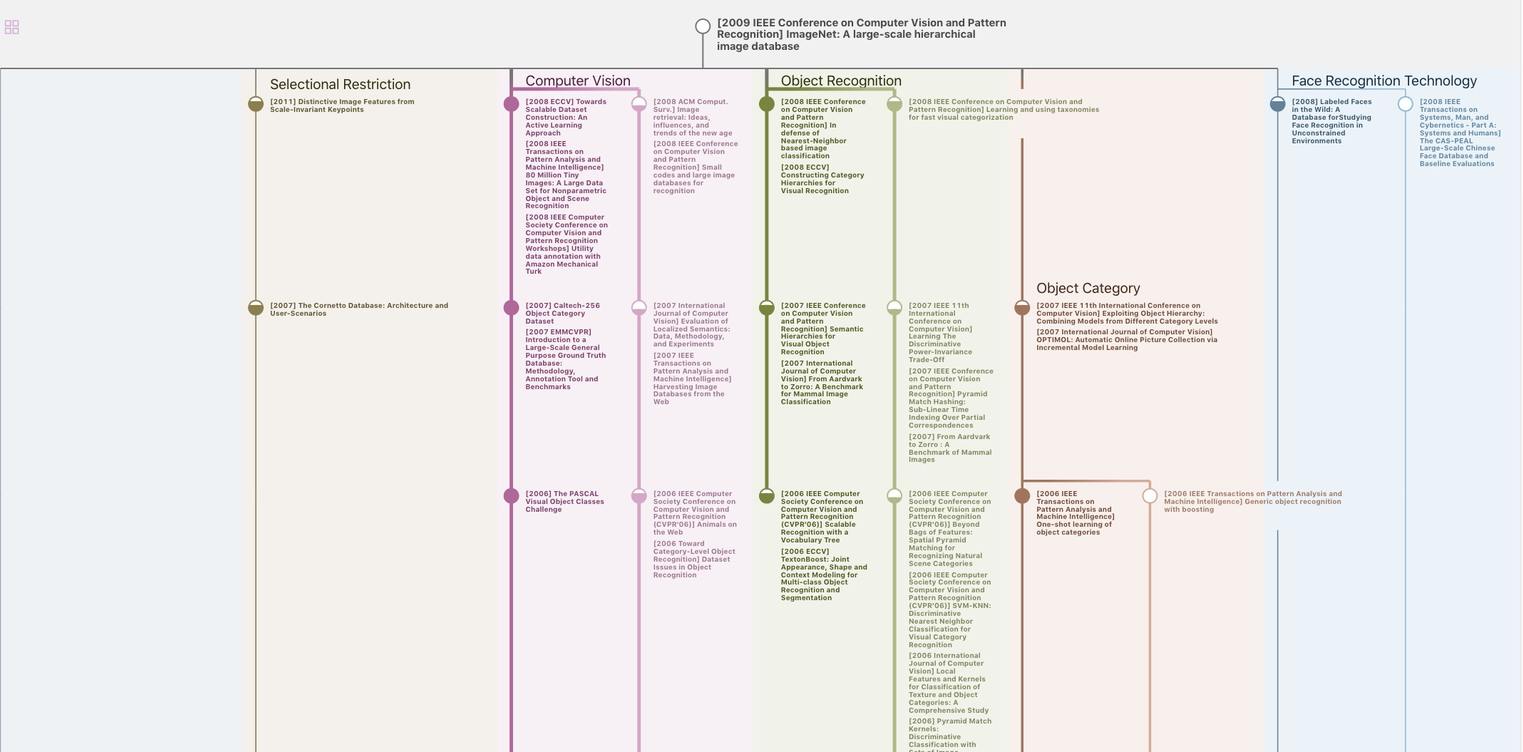
生成溯源树,研究论文发展脉络
Chat Paper
正在生成论文摘要