Generative image inpainting with salient prior and relative total variation
Journal of Visual Communication and Image Representation(2021)
摘要
Image inpainting is an important research direction of image processing. The generative adversarial network (GAN), which can reconstruct new reasonable content in the corrupted region, is the most interesting tool in current inpainting technologies. However, the previous deep methods generally need to be pre-added the binary mask representing the corruption location as the extra input. A novel inpainting algorithm which does not require additional external labels is proposed in this paper. The algorithm consists of two parts: corruption recognition module and content inpainting module, which can recognize and fill random corruption. In the recognizer, the salient object from the uncorrupted region is used as the prior for distinguishing corruption. In the inpainting module, a two-stage network is applied to reconstruct the image from coarse content to texture details. To avoid the misdetection in recognition which has a negative impact on the restoration in inpainting, we perform relative total variational filtering on the corrupted image, and use the salient map as the supervision of detail reconstruction. Qualitative and quantitative experiments on multiple datasets verify the effectiveness of our recognition module, the competitive advantage of our inpainting module, and the enlightening significance of our total algorithm in image inpainting.
更多查看译文
关键词
Image inpainting,GAN,Corruption recognition,Salient prior,Relative total variation
AI 理解论文
溯源树
样例
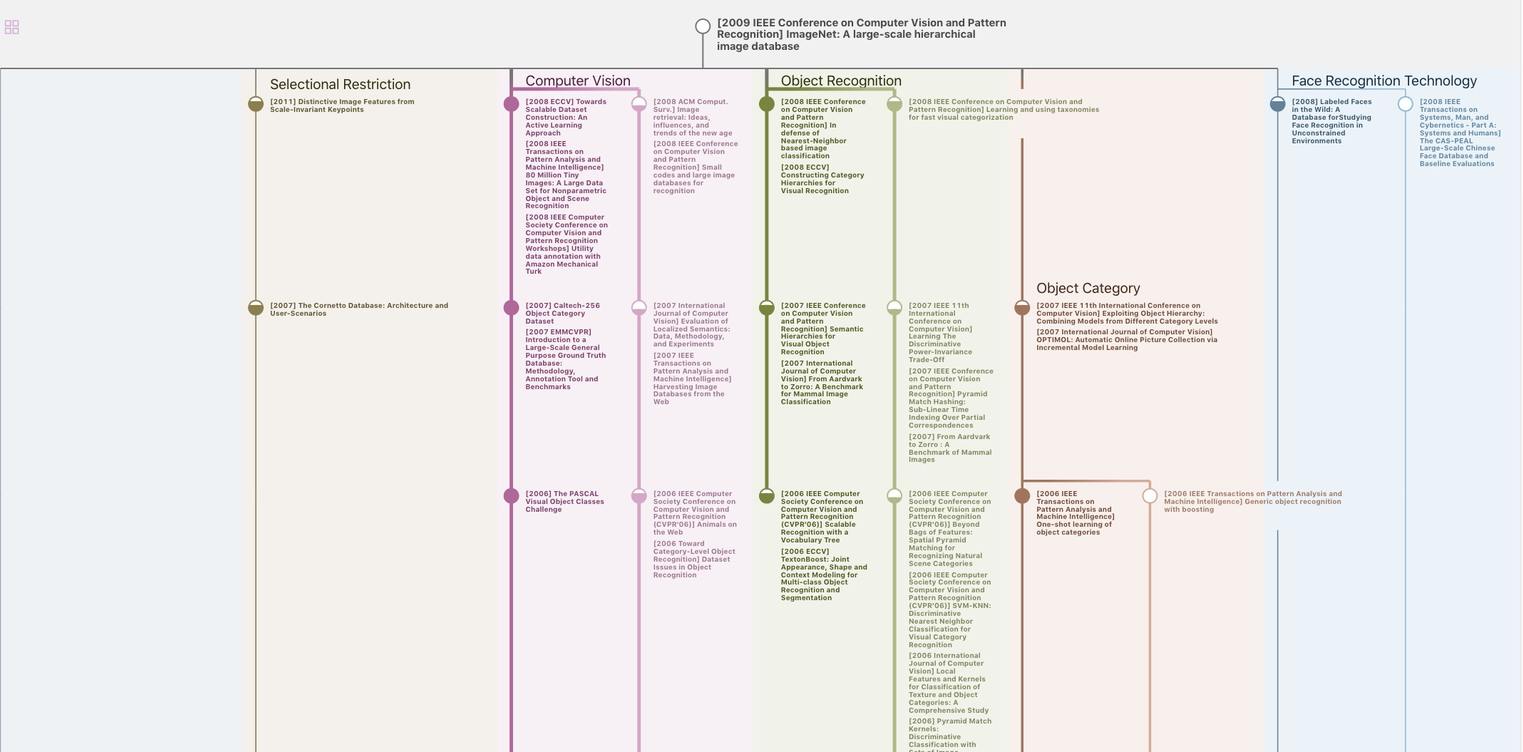
生成溯源树,研究论文发展脉络
Chat Paper
正在生成论文摘要