Detecting Extremely Small Lesions in Mouse Brain MRI with Point Annotations via Multi-task Learning.
MLMI@MICCAI(2021)
摘要
Detection of small lesions in magnetic resonance imaging (MRI) images is one of the most challenging tasks. Compared with detection in natural images, small lesion detection in MRI images faces two major problems: First, small lesions only occupy a small fraction of voxels within an image, yielding insufficient features and information for them to be distinguished from the surrounding tissues. Second, an accurate outline of these small lesions manually is time-consuming and inefficient even for medical experts in pathology. Hence, existing methods cannot accurately detect lesions with such a limited amount of information. To solve these problems, we propose a novel multi-task convolutional neural network (CNN), which simultaneously performs regression of lesion number and detection of lesion location. Both lesion number and location can be obtained through point annotations, which is much easier and efficient than a full segmentation of lesionmanually. We use an encoder-decoder structure that outputs a distance map of each pixel to the nearest lesion centers. Additionally, a regression branch is added after the encoder to learn the counting of lesion numbers, thus providing an extra regularization. Note that these two tasks share the same encoder weights. We demonstrate that our model enables the counting and locating of extremely small lesions within 3-5 voxels (300 x 300 voxels per image) with a recall of 72.66% on a large mouse brain MRI image dataset (more than 1000 images), and outperforms other methods.
更多查看译文
关键词
MRI,Mouse brain,Lesion detection,Point annotations,Multi-task learning
AI 理解论文
溯源树
样例
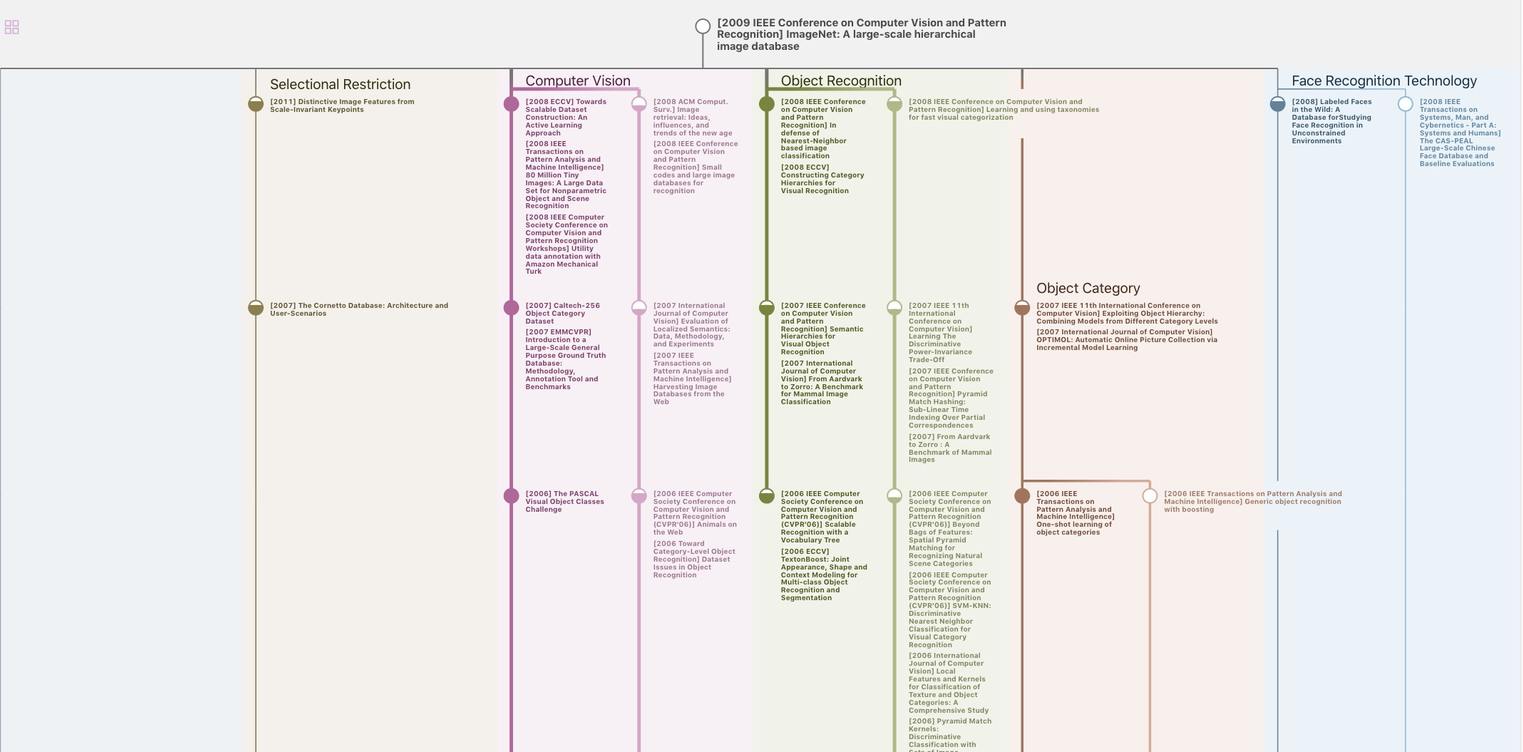
生成溯源树,研究论文发展脉络
Chat Paper
正在生成论文摘要