Optimised Code-Switched Language Model Data Augmentation in Four Under-Resourced South African Languages.
SPECOM(2021)
摘要
Code-switching in South African languages is common but data for language modelling remains extremely scarce. We present techniques that allow recurrent neural networks (LSTMs) to be better applied as generative models to the task of producing artificial code-switched text that can be used to augment the small training sets. We propose the application of prompting to favour the generation of sentences with intra-sentential language switches, and introduce an extensive LSTM hyperparameter search that specifically optimises the utility of the artificially generated code-switched text. We use these strategies to generate artificial code-switched text for four under-resourced South African languages and evaluate the utility of this additional data for language modelling. We find that the optimised models are able to generate text that leads to consistent perplexity and word error rate improvements for all four language pairs, especially at language switches. This is an improvement on previous work using the same speech data in which text generated without such optimisation did not provide improved performance. We conclude that prompting and targeted hyperparameter optimisation are an effective means of improving language model data augmentation for code-switched speech recognition.
更多查看译文
关键词
Code-switching, Language model data augmentation, LSTM, Speech recognition, Under-resourced languages, African languages, Bantu languages
AI 理解论文
溯源树
样例
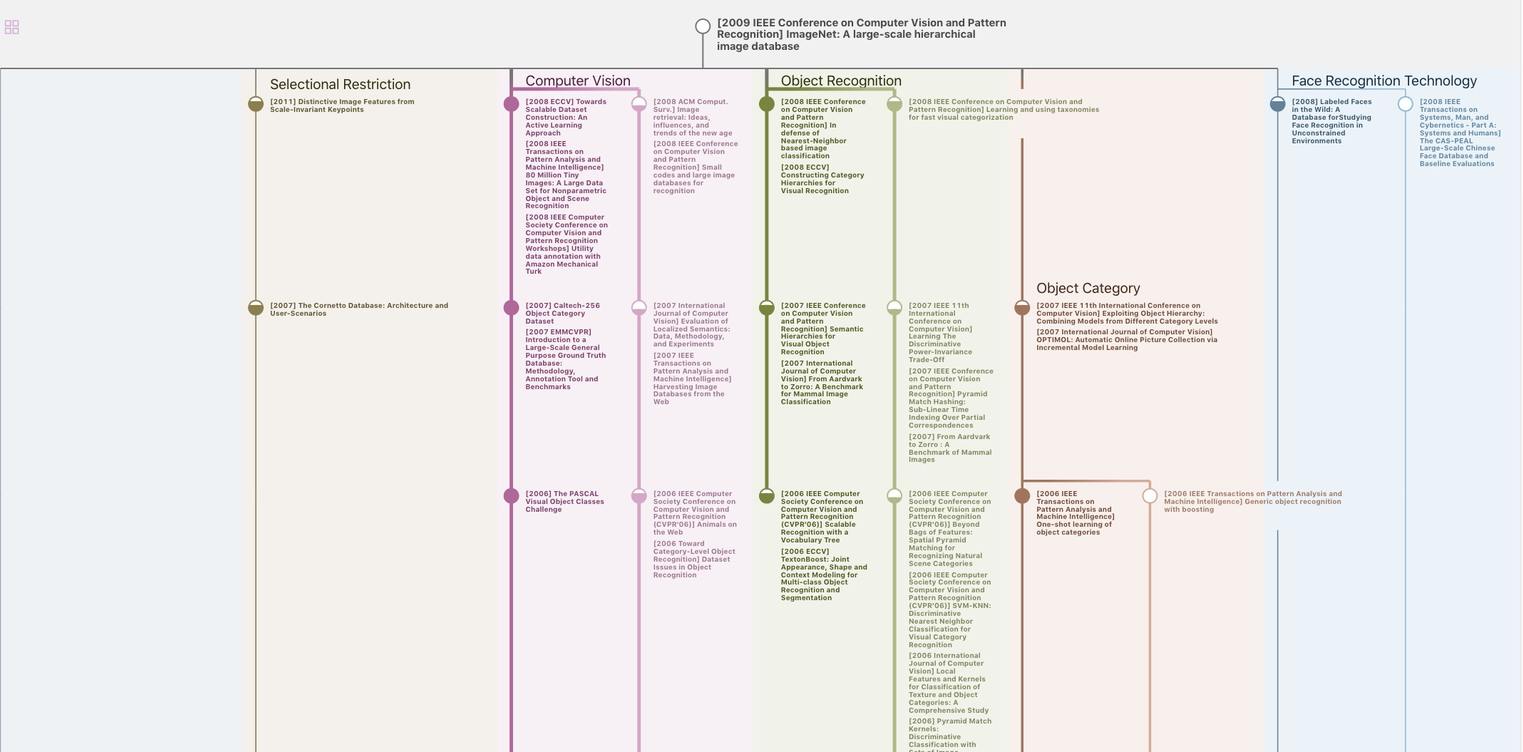
生成溯源树,研究论文发展脉络
Chat Paper
正在生成论文摘要