Boosting Local Recommendations With Partially Trained Global Model
15TH ACM CONFERENCE ON RECOMMENDER SYSTEMS (RECSYS 2021)(2021)
Abstract
Building recommendation systems for enterprise software has many unique challenges that are different from consumer-facing systems. When applied to different organizations, the data used to power those recommendation systems vary substantially in both quality and quantity due to differences in their operational practices, marketing strategies, and targeted audiences. At Salesforce, as a cloud provider of such a system with data across many different organizations, naturally, it makes sense to pool data from different organizations to build a model that combines all values from different brands. However, multiple issues like how do we make sure a model trained with pooled data can still capture customer specific characteristics, how do we design the system to handle those data responsibly and ethically, i.e., respecting contractual agreements with our clients, legal and compliance requirements, and the privacy of all the consumers. In this proposal, We present a framework that not only utilizes enriched user-level data across organizations, but also boosts business-specific characteristics in generating personal recommendations. We will also walk through key privacy considerations when designing such a system.
MoreTranslated text
Key words
global model,privacy,recommendation system
AI Read Science
Must-Reading Tree
Example
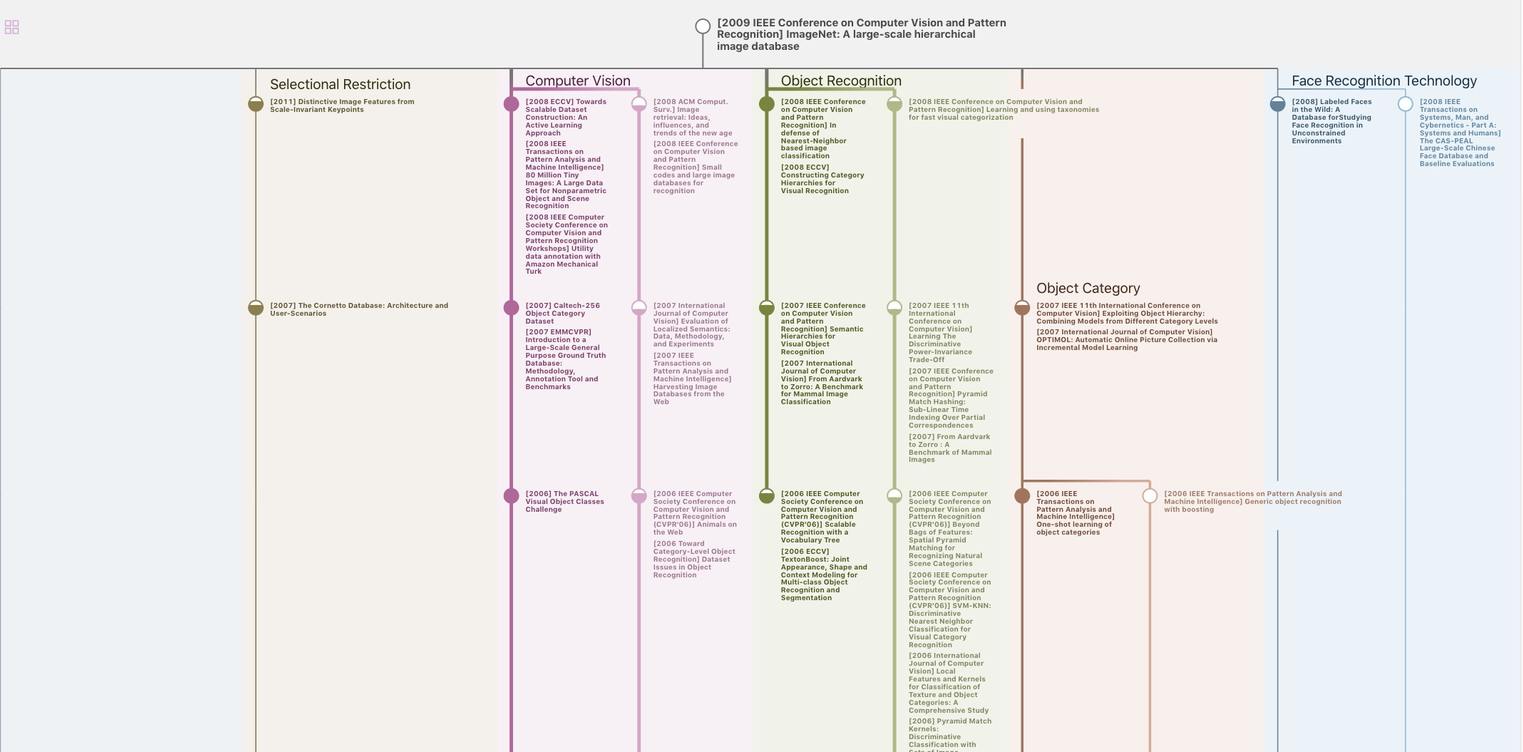
Generate MRT to find the research sequence of this paper
Chat Paper
Summary is being generated by the instructions you defined