Learning Spherical Convolution for $360^{\circ }$ 360 ∘ Recognition
IEEE Transactions on Pattern Analysis and Machine Intelligence(2022)
摘要
While
$360^{\circ }$
cameras offer tremendous new possibilities in vision, graphics, and augmented reality, the spherical images they produce make visual recognition non-trivial. Ideally,
$360^{\circ }$
imagery could inherit the deep convolutional neural networks (CNNs) already trained with great success on perspective projection images. However, spherical images cannot be projected to a single plane without significant distortion, and existing methods to transfer CNNs from perspective to spherical images introduce significant computational costs and/or degradations in accuracy. We propose to
learn
a Spherical Convolution Network (SphConv) that translates a planar CNN to the equirectangular projection of
$360^{\circ }$
images. Given a source CNN for perspective images as input, SphConv learns to reproduce the flat filter outputs on
$360^{\circ }$
data, sensitive to the varying distortion effects across the viewing sphere. The key benefits are 1) efficient and accurate recognition for
$360^{\circ }$
images, and 2) the ability to leverage powerful pre-trained networks for perspective images. We further proposes two instantiation of SphConv—Spherical Kernel learns location dependent kernels on the sphere for SphConv, and Kernel Transformer Network learns a functional transformation that generates SphConv kernels from the source CNN. Among the two variants, Kernel Transformer Network has a much lower memory footprint at the cost of higher computational overhead. Validating our approach with multiple source CNNs and datasets, we show that SphConv using KTN successfully preserves the source CNN’s accuracy, while offering efficiency, transferability, and scalability to typical image resolutions. We further introduce a spherical Faster R-CNN model based on SphConv and show that we can learn a spherical object detector without any object annotation in
$360^{\circ }$
images.
更多查看译文
关键词
$360^{\circ }$ 360 ∘ video analysis,omnidirectional video,object detection
AI 理解论文
溯源树
样例
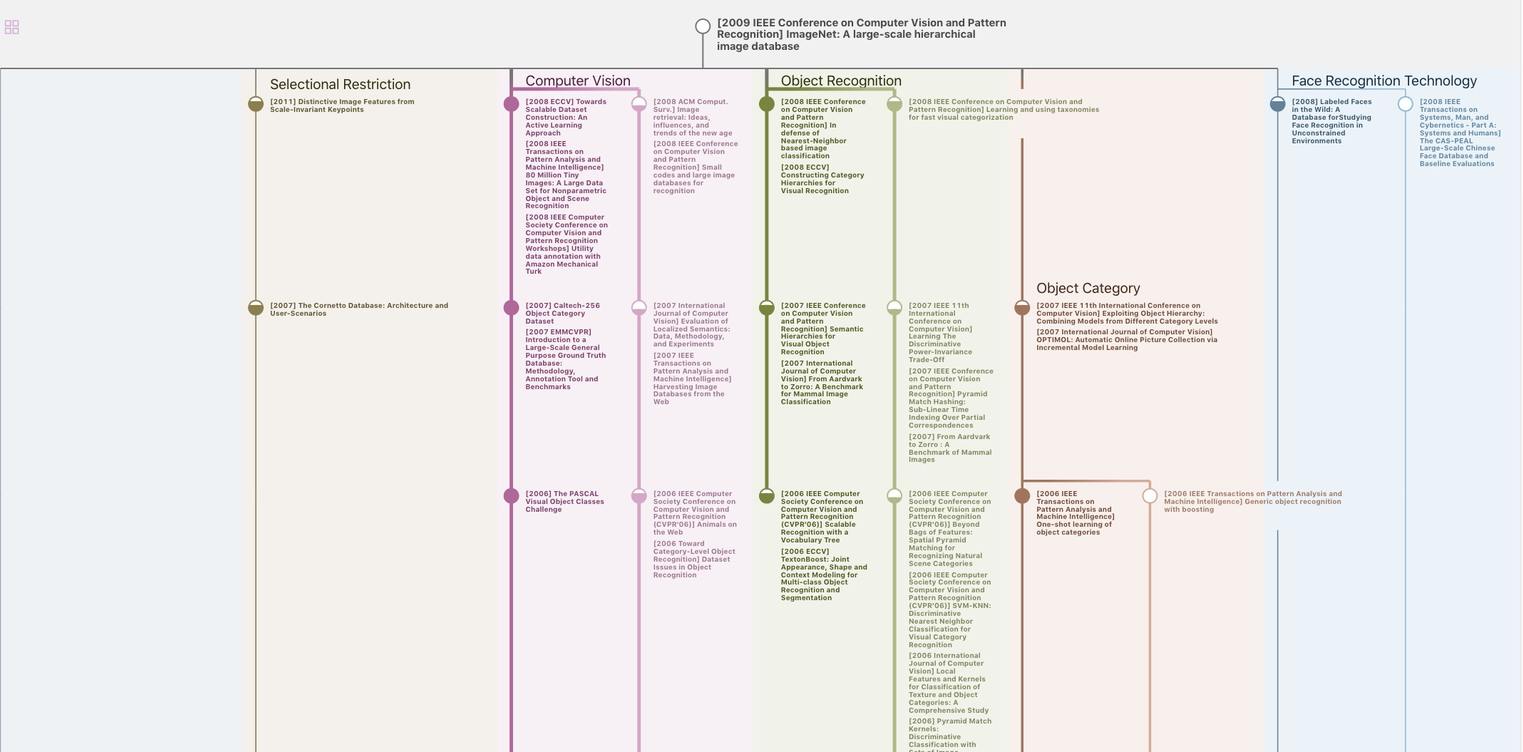
生成溯源树,研究论文发展脉络
Chat Paper
正在生成论文摘要