Passage Similarity and Diversification in Non-factoid Question Answering.
ICTIR(2021)
摘要
The rise in popularity of mobile and voice search has led to a shift in focus from document retrieval to short answer passage retrieval for non-factoid questions. Some of the questions have multiple answers, and the aim is to retrieve a set of relevant answer passages, which covers all these alternatives. Compared to documents, answers are more specific and typically form more defined types or groups. Grouping answer passages based on strong similarity measures may provide a means of identifying these types. Typically, kNN clustering in combination with term-based representations have been used in Information Retrieval (IR) scenarios. An alternate method is to use pre-trained distributional representations such as GloVe and BERT, which capture additional semantic relationships. The recent success of trained neural models for various tasks provides the motivation for generating more task-specific representations. However, due to the absence of large datasets for incorporating passage level similarity information, a more feasible alternative is to use weak supervision based training. This information can then be used to generate a final ranked list of diversified answers using standard diversification algorithms. In this paper, we introduce a new dataset NFPassageQA_Sim, with human annotated similarity labels for pairs of answer passages corresponding to each question. These similarity labels are then processed to generate another dataset NFPassageQA_Div, which consists of answer types for these questions. Using the similarity labels, we demonstrate the effectiveness of using weak supervision signals derived from GloVe, fine-tuned and trained using a BERT model for the task of answer passage clustering. Finally, we introduce a model which incorporates these clusters into a MMR (Maximal Marginal Relevance) model, which significantly beats other diversification baselines using both diversity and relevance metrics.
更多查看译文
AI 理解论文
溯源树
样例
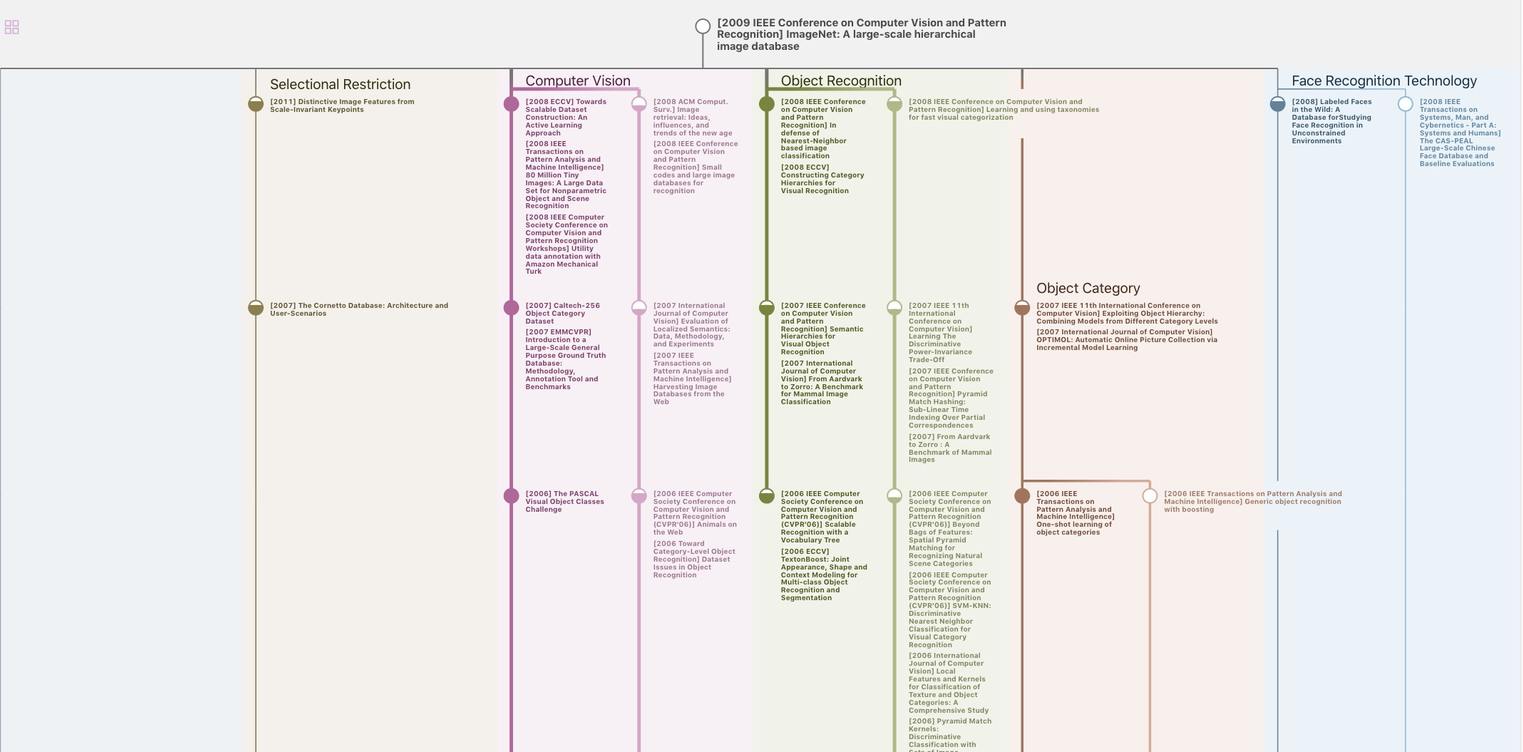
生成溯源树,研究论文发展脉络
Chat Paper
正在生成论文摘要