D-REX: Dialogue Relation Extraction with Explanations
PROCEEDINGS OF THE 4TH WORKSHOP ON NLP FOR CONVERSATIONAL AI(2022)
Abstract
Existing research studies on cross-sentence relation extraction in long-form multi-party conversations aim to improve relation extraction without considering the explainability of such methods. This work addresses that gap by focusing on extracting explanations that indicate that a relation exists while using only partially labeled explanations. We propose our model-agnostic framework, D-REX, a policy-guided semi-supervised algorithm that optimizes for explanation quality and relation extraction simultaneously. We frame relation extraction as a re-ranking task and include relation- and entity-specific explanations as an intermediate step of the inference process. We find that human annotators are 4.2 times more likely to prefer D-REX's explanations over a joint relation extraction and explanation model. Finally, our evaluations show that D-REX is simple yet effective and improves relation extraction performance of strong baseline models by 1.2-4.7%.(1)
MoreTranslated text
Key words
dialogue relation extraction,explanations,d-rex
AI Read Science
Must-Reading Tree
Example
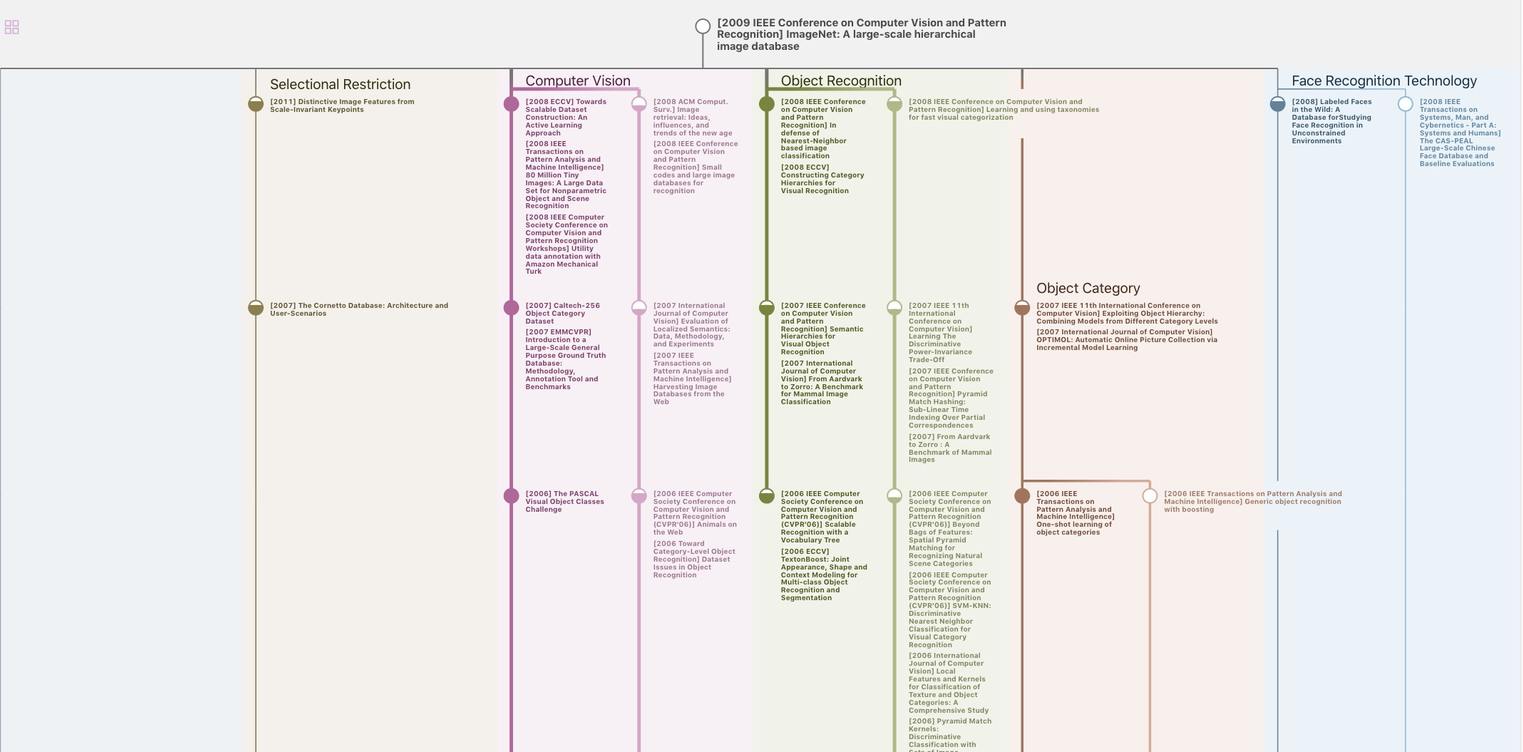
Generate MRT to find the research sequence of this paper
Chat Paper
Summary is being generated by the instructions you defined