Differentiation of multiple system atrophy subtypes by gray matter atrophy
JOURNAL OF NEUROIMAGING(2022)
摘要
Background and Purpose Multiple system atrophy(MSA) is a rare adult-onset synucleinopathy that can be divided in two subtypes depending on whether the prevalence of its symptoms is more parkinsonian or cerebellar (MSA-P and MSA-C, respectively). The aim of this work is to investigate the structural MRI changes able to discriminate MSA phenotypes. Methods The sample includes 31 MSA patients (15 MSA-C and 16 MSA-P) and 39 healthy controls. Participants underwent a comprehensive motor and neuropsychological battery. MRI data were acquired with a 3T scanner (MAGNETOM Trio, Siemens, Germany). FreeSurfer was used to obtain volumetric and cortical thickness measures. A Support Vector Machine (SVM) algorithm was used to assess the classification between patients' group using cortical and subcortical structural data. Results After correction for multiple comparisons, MSA-C patients had greater atrophy than MSA-P in the left cerebellum, whereas MSA-P showed reduced volume bilaterally in the pallidum and putamen. Using deep gray matter volume ratios and mean cortical thickness as features, the SVM algorithm provided a consistent classification between MSA-C and MSA-P patients (balanced accuracy 74.2%, specificity 75.0%, and sensitivity 73.3%). The cerebellum, putamen, thalamus, ventral diencephalon, pallidum, and caudate were the most contributing features to the classification decision (z > 3.28; p < .05 [false discovery rate]). Conclusions MSA-C and MSA-P with similar disease severity and duration have a differential distribution of gray matter atrophy. Although cerebellar atrophy is a clear differentiator between groups, thalamic and basal ganglia structures are also relevant contributors to distinguishing MSA subtypes.
更多查看译文
关键词
cognition, cortical thickness, multiple system atrophy, machine learning, neuroimaging
AI 理解论文
溯源树
样例
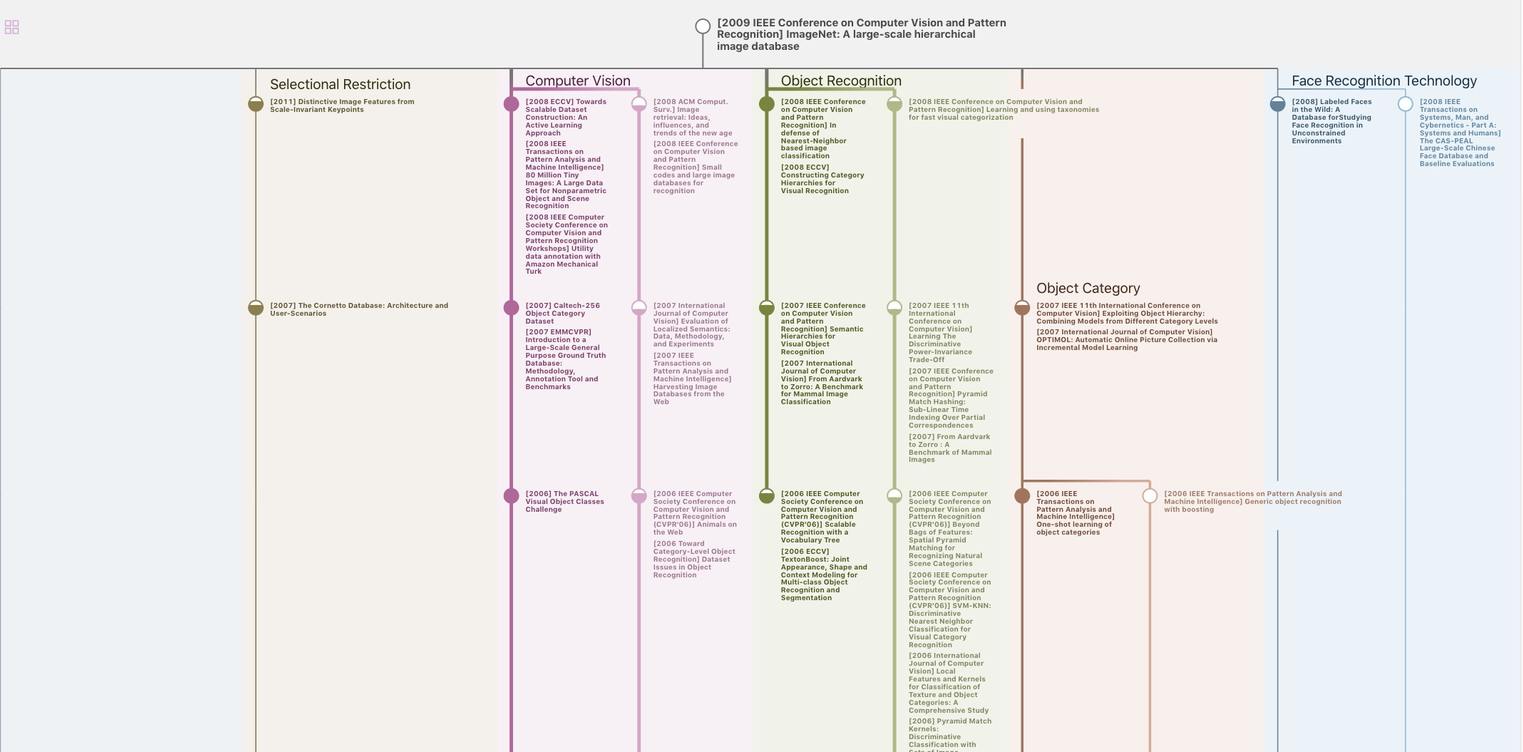
生成溯源树,研究论文发展脉络
Chat Paper
正在生成论文摘要