Learning Control Sets for Lattice Planners from User Preferences.
WAFR(2021)
摘要
This work investigates the design of a motion planner that can capture user preferences. In detail, we generate a sparse control set for a lattice planner which closely follows the preferences of a user. Given a set of demonstrated trajectories from a single user, we estimate user preferences based on a weighted sum of trajectory features. We then optimize a set of connections in the lattice of given size for the user cost function. The restricted number of connections limits the branching factor, ensuring strong performance during subsequent motion planning. Further, every trajectory in the control set reflects the learned user preference while the sub-optimality due to the size restriction is minimized. We show that this problem is optimally solved by applying a separation principle: First, we find the best estimate of the user cost function given the data, then an optimal control set is computed given that estimate. We evaluate our work in a simulation for an autonomous robot in a four-dimensional spatiotemporal lattice and show that the proposed approach is suitable to replicate the demonstrated behaviour while enjoying substantially increased performance.
更多查看译文
关键词
lattice planners,control sets,user preferences
AI 理解论文
溯源树
样例
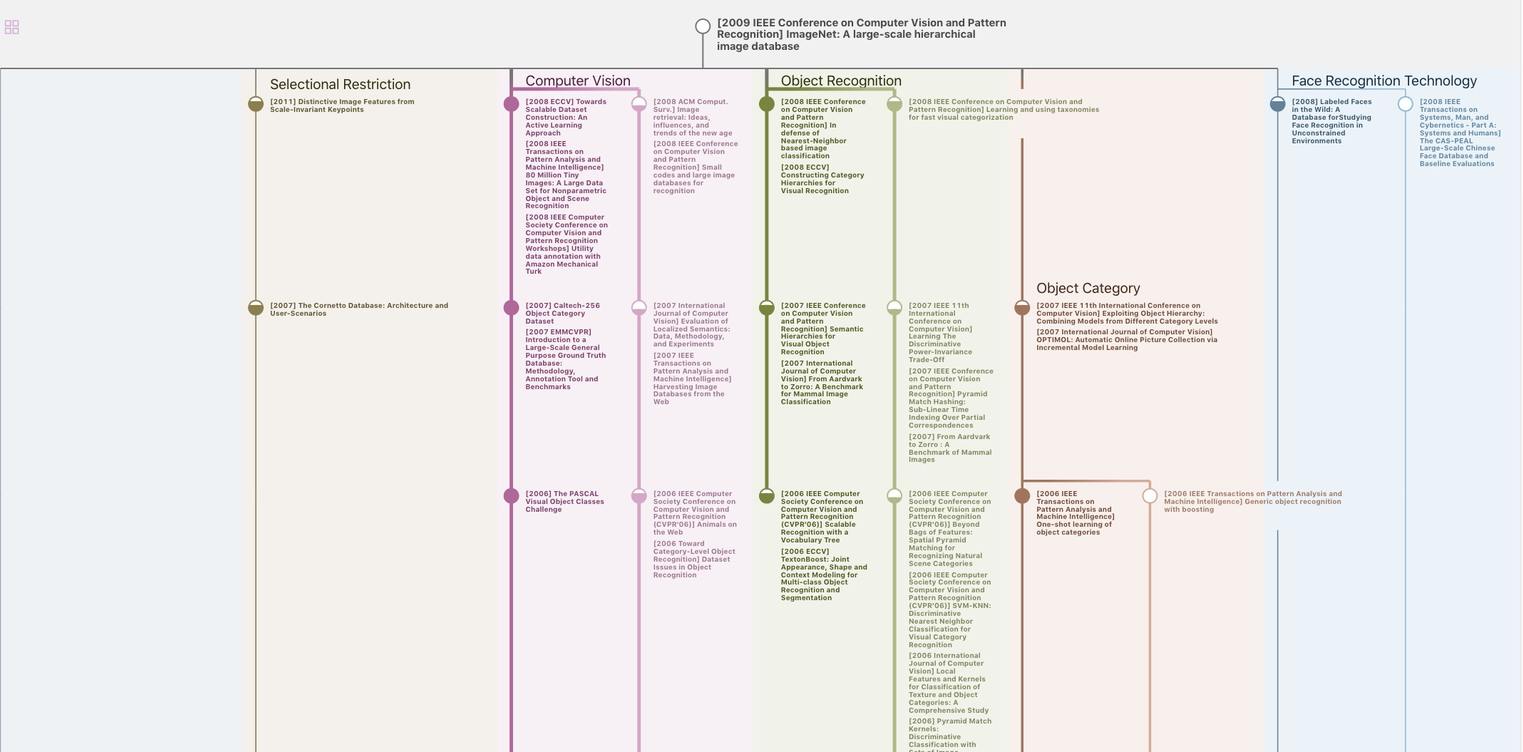
生成溯源树,研究论文发展脉络
Chat Paper
正在生成论文摘要