Automatic ICD-10 Coding and Training System: Deep Neural Network Based on Supervised Learning.
JMIR medical informatics(2021)
摘要
BACKGROUND:The International Classification of Diseases (ICD) code is widely used as the reference in medical system and billing purposes. However, classifying diseases into ICD codes still mainly relies on humans reading a large amount of written material as the basis for coding. Coding is both laborious and time-consuming. Since the conversion of ICD-9 to ICD-10, the coding task became much more complicated, and deep learning- and natural language processing-related approaches have been studied to assist disease coders.
OBJECTIVE:This paper aims at constructing a deep learning model for ICD-10 coding, where the model is meant to automatically determine the corresponding diagnosis and procedure codes based solely on free-text medical notes to improve accuracy and reduce human effort.
METHODS:We used diagnosis records of the National Taiwan University Hospital as resources and apply natural language processing techniques, including global vectors, word to vectors, embeddings from language models, bidirectional encoder representations from transformers, and single head attention recurrent neural network, on the deep neural network architecture to implement ICD-10 auto-coding. Besides, we introduced the attention mechanism into the classification model to extract the keywords from diagnoses and visualize the coding reference for training freshmen in ICD-10. Sixty discharge notes were randomly selected to examine the change in the F
1RESULTS:In experiments on the medical data set of National Taiwan University Hospital, our prediction results revealed F
11CONCLUSIONS:The proposed model significantly improved the F
1
更多查看译文
关键词
International Classification of Diseases,Recurrent Neural Network,deep learning,natural language processing,text classification
AI 理解论文
溯源树
样例
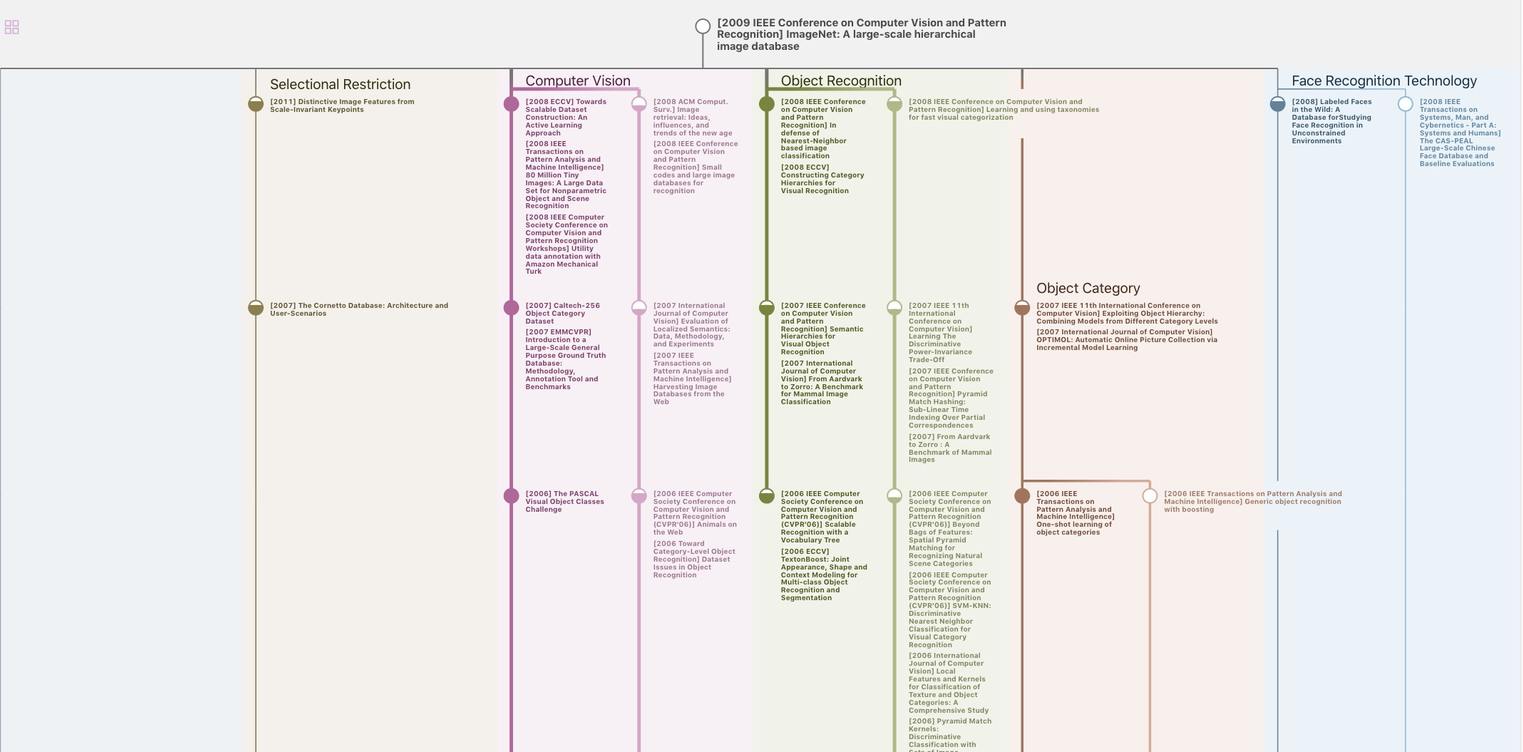
生成溯源树,研究论文发展脉络
Chat Paper
正在生成论文摘要