Joint modelling of longitudinal measurements and survival times via a multivariate copula approach
arxiv(2023)
摘要
Joint modelling of longitudinal and time-to-event data is usually described by a joint model which uses shared or correlated latent effects to capture associations between the two processes. Under this framework, the joint distribution of the two processes can be derived straightforwardly by assuming conditional independence given the random effects. Alternative approaches to induce interdependency into sub-models have also been considered in the literature and one such approach is using copulas to introduce non-linear correlation between the marginal distributions of the longitudinal and time-to-event processes. The multivariate Gaussian copula joint model has been proposed in the literature to fit joint data by applying a Monte Carlo expectation-maximisation algorithm. In this paper, we propose an exact likelihood estimation approach to replace the more computationally expensive Monte Carlo expectation-maximisation algorithm and we consider results based on using both the multivariate Gaussian and t copula functions. We also provide a straightforward way to compute dynamic predictions of survival probabilities, showing that our proposed model is comparable in prediction performance to the shared random effects joint model.
更多查看译文
关键词
Longitudinal data,time-to-event data,joint modelling,multivariate copula,dynamic prediction,likelihood approach
AI 理解论文
溯源树
样例
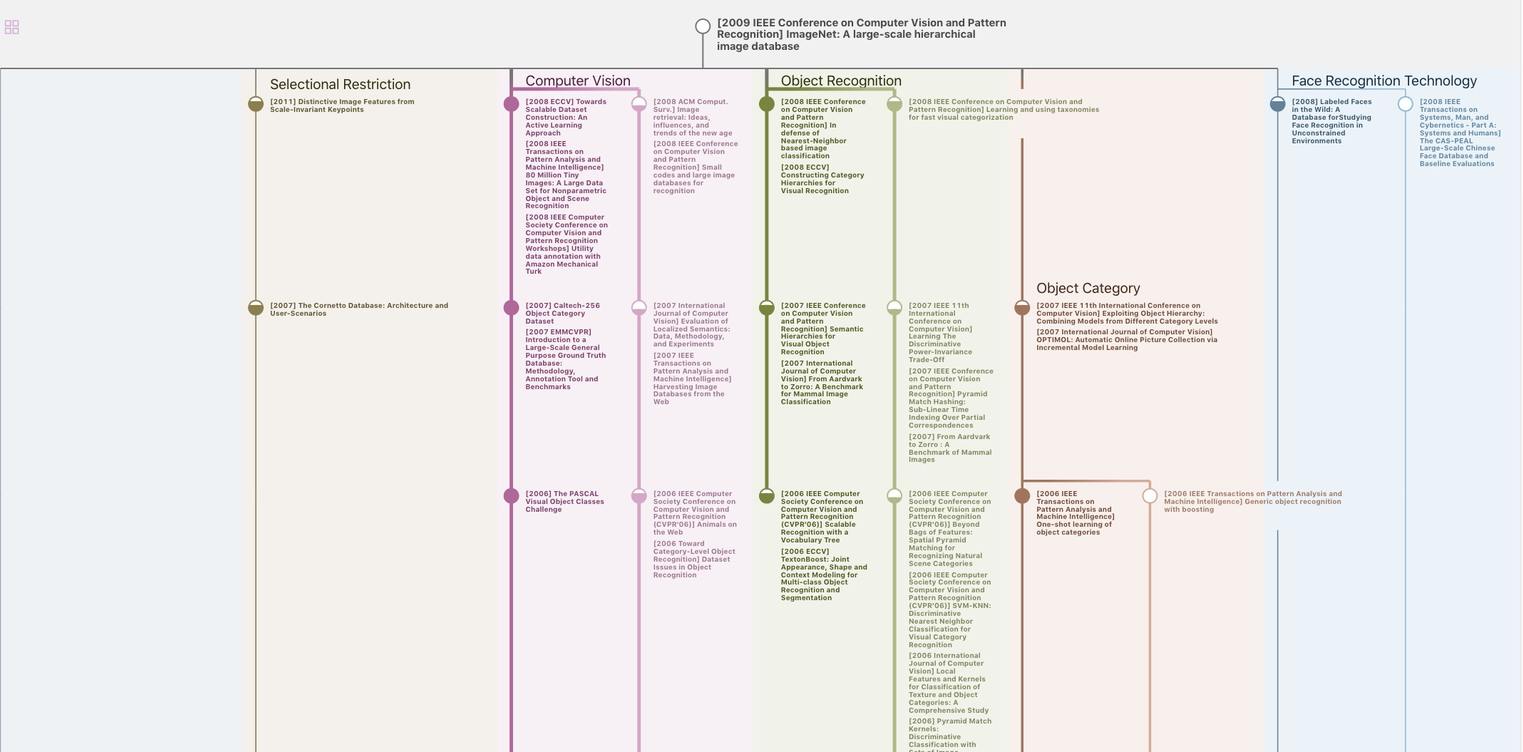
生成溯源树,研究论文发展脉络
Chat Paper
正在生成论文摘要