SimVLM: Simple Visual Language Model Pretraining with Weak Supervision
International Conference on Learning Representations (ICLR)(2022)
摘要
With recent progress in joint modeling of visual and textual representations, Vision-Language Pretraining (VLP) has achieved impressive performance on many multimodal downstream tasks. However, the requirement for expensive annotations including clean image captions and regional labels limits the scalability of existing approaches, and complicates the pretraining procedure with the introduction of multiple dataset-specific objectives. In this work, we relax these constraints and present a minimalist pretraining framework, named Simple Visual Language Model (SimVLM). Unlike prior work, SimVLM reduces the training complexity by exploiting large-scale weak supervision, and is trained end-to-end with a single prefix language modeling objective. Without utilizing extra data or task-specific customization, the resulting model significantly outperforms previous pretraining methods and achieves new state-of-the-art results on a wide range of discriminative and generative vision-language benchmarks, including VQA (+3.74% vqa-score), NLVR2 (+1.17% accuracy), SNLI-VE (+1.37% accuracy) and image captioning tasks (+10.1% average CIDEr score). Furthermore, we demonstrate that SimVLM acquires strong generalization and transfer ability, enabling zero-shot behavior including open-ended visual question answering and cross-modality transfer.
更多查看译文
关键词
Vision-Language Pretraining,Multimodal Language Model,Weak Supervision
AI 理解论文
溯源树
样例
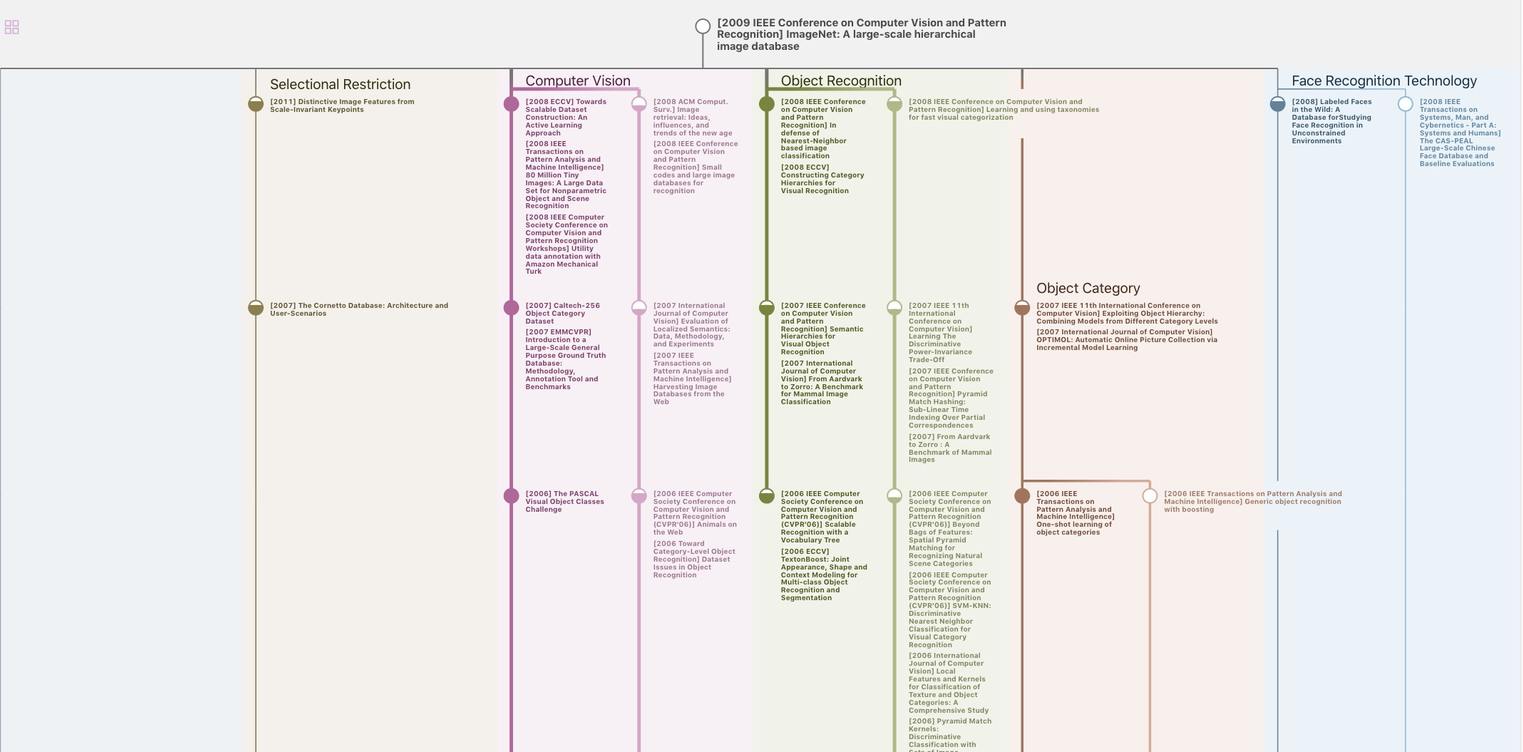
生成溯源树,研究论文发展脉络
Chat Paper
正在生成论文摘要