Lévy State-Space Models for Tracking and Intent Prediction of Highly Maneuverable Objects
IEEE Transactions on Aerospace and Electronic Systems(2021)
摘要
In this article, we present a Bayesian framework for maneuvering object tracking and intent prediction using novel α-stable Lévy state-space models, expressed in continuous time as Lévy processes. In contrast to conventional (fully) Gaussian formulations, the proposed models are driven by heavy-tailed α-stable noise and are, thus, much more able to capture extreme values/behaviors. This can better characterize sharp changes in the state, which may be induced by sudden and frequent maneuvers such as swift turns or abrupt accelerations. In particular, they are represented in a conditionally Gaussian series form, which ensures the tractability of the applied inference algorithms. A corresponding estimation strategy with the Rao-Blackwellized particle filter is then proposed, and an efficient intent inference procedure is introduced. Here, the underlying intent, driving the target's long-term behavior (e.g., reaching its final destination), is modeled as a latent variable. Real vessel data from maritime surveillance and human computer interactions (e.g., cursor data from motor-impaired interface users) are utilized to demonstrate the effectiveness of the proposed approach. It is shown to deliver noticeable improvements in the tracking and intent prediction performance (whenever relevant) compared with a more conventional Gaussian dynamic model.
更多查看译文
关键词
$\alpha$ -stable laws,intent inference,Lévy process,maneuver tracking,Poisson series representation,Rao–Blackwellized particle filtering
AI 理解论文
溯源树
样例
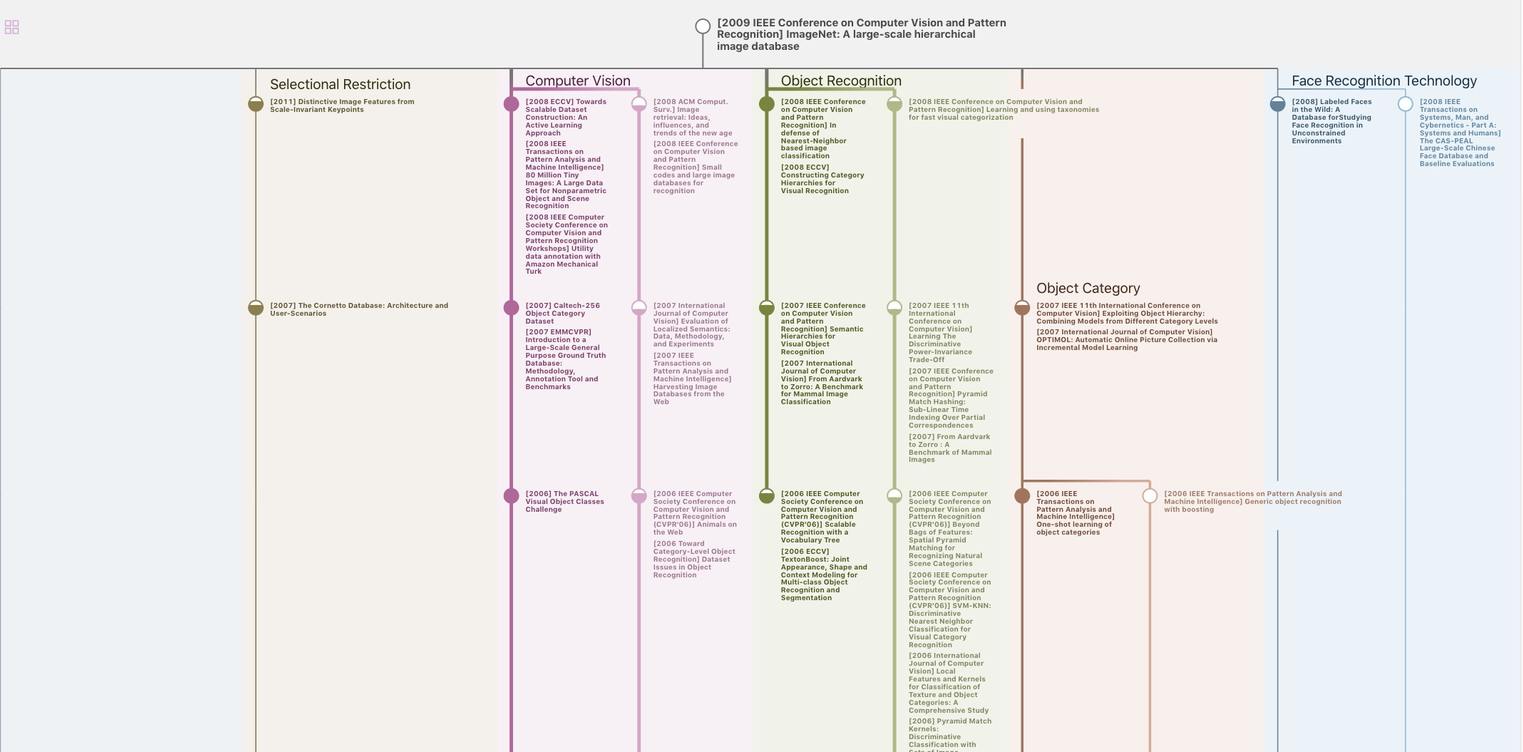
生成溯源树,研究论文发展脉络
Chat Paper
正在生成论文摘要