Contextualised Word Embeddings Based on Transfer Learning to Dialogue Response Generation - a Proposal and Comparisons.
ISEEIE(2021)
摘要
Contextualised word embeddings have recently become essential elements of Natural Language Processing (NLP) systems since these embedding models encode not only words but also their contexts to generate context-specific representations. Pre-trained models such as BERT, GPT, and derived architectures are increasingly present on NLP task benchmarks. Several comparative analyses of such models have been performed, but so far no one compares the most recent architectures in a dialogue generation dataset by considering multiple metrics relevant to the task. In this paper, we not only propose an encoder-decoder system that uses transfer learning with pre-trained word embeddings, but we also systematically compare various pretrained contextualised word embedding architectures on the DSTC-7 dataset, using metrics based on mutual information, dialogue length, and variety of answers. We use the word embeddings as a first layer of the encoder, making it possible to encode the texts in a latent space. As a decoder, we use an LSTM layer and a byte pair encoding tokenisation, aligned with state-of-the-art dialogue systems recently published. The networks are trained during the same amount of epochs, with the same optimisers and learning rates. Considering the quality of the dialogue, our results show that there is no superior technique on all metrics. However, there are relevant differences concerning the computational costs to encode the data.
更多查看译文
AI 理解论文
溯源树
样例
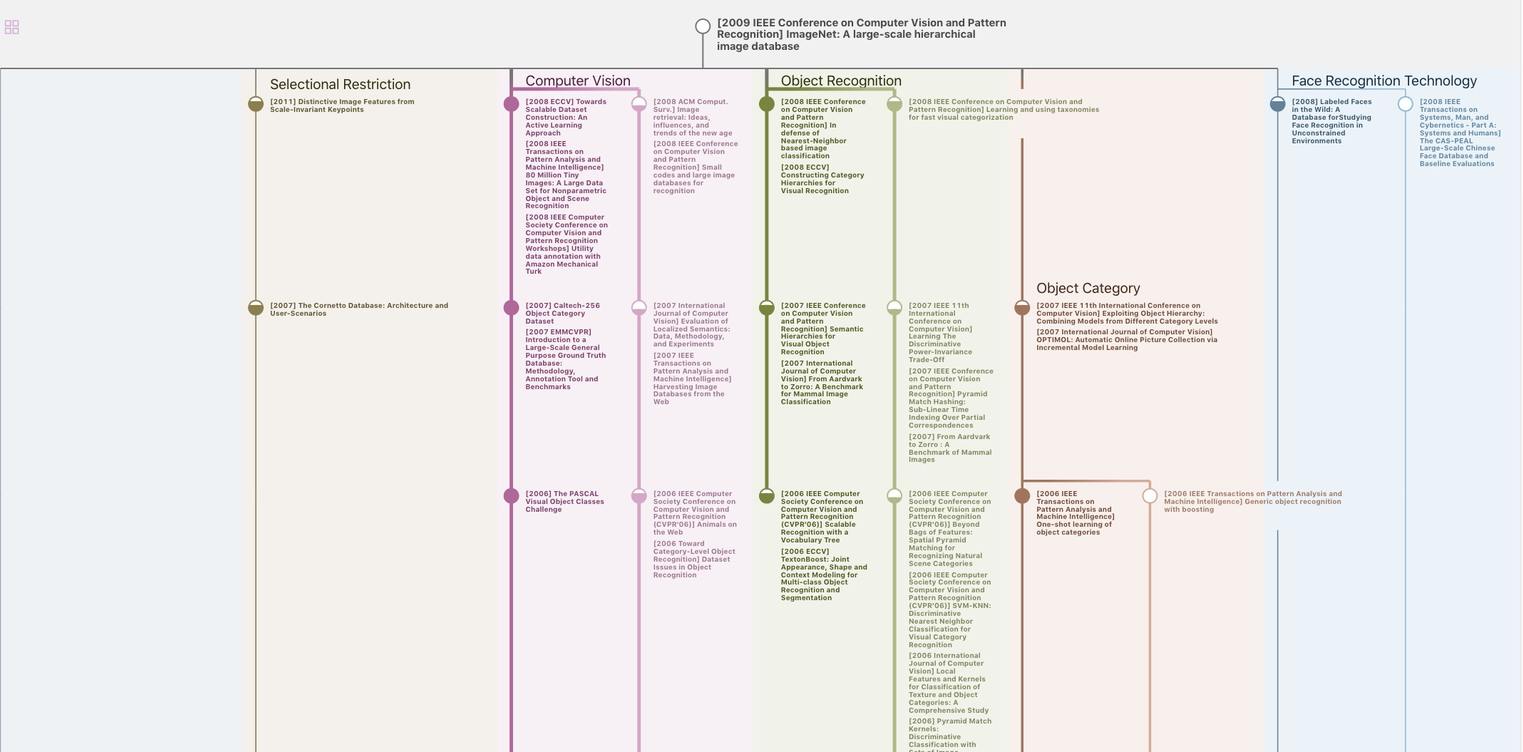
生成溯源树,研究论文发展脉络
Chat Paper
正在生成论文摘要