Computation of low-rank tensor approximation under existence constraint via a forward-backward algorithm
Periodicals(2021)
摘要
AbstractHighlights •Performance analysis of iterative algorithms for the calculation of the CP decomposition.•A novel problem formulation for the CP tensor decomposition via logarithmic regularized barriers.•Forward-backward algorithm to compute the CP tensor decomposition under coherence constraints.•Accelerated proximal gradient remains slightly faster than forward-backward for difficult cases.•Better performance of forward-backward compared to other algorithms for less difficult cases. AbstractThe Canonical Polyadic (CP) tensor decomposition has become an attractive mathematical tool in several fields during the last ten years. This decomposition is very powerful for representing and analyzing multidimensional data. The most attractive feature of the CP decomposition is its uniqueness, contrary to rank-revealing matrix decompositions, where the problem of rotational invariance remains. This paper presents the performance analysis of iterative descent algorithms for calculating the CP decomposition of tensors when columns of factor matrices are almost collinear – i.e. swamp problems arise. We propose in this paper a new and efficient proximal algorithm based on the Forward Backward splitting method. More precisely, the existence of the best low-rank tensor approximation is ensured thanks to a coherence constraint implemented via a logarithmic regularized barrier. Computer experiments demonstrate the efficiency and stability of the proposed algorithm in comparison to other iterative algorithms in the literature for the normal case, and also producing significant results even in difficult situations.
更多查看译文
关键词
CP decomposition, Tensor, Coherence constraint, Swamp, Forward backward splitting, Low-rank approximation
AI 理解论文
溯源树
样例
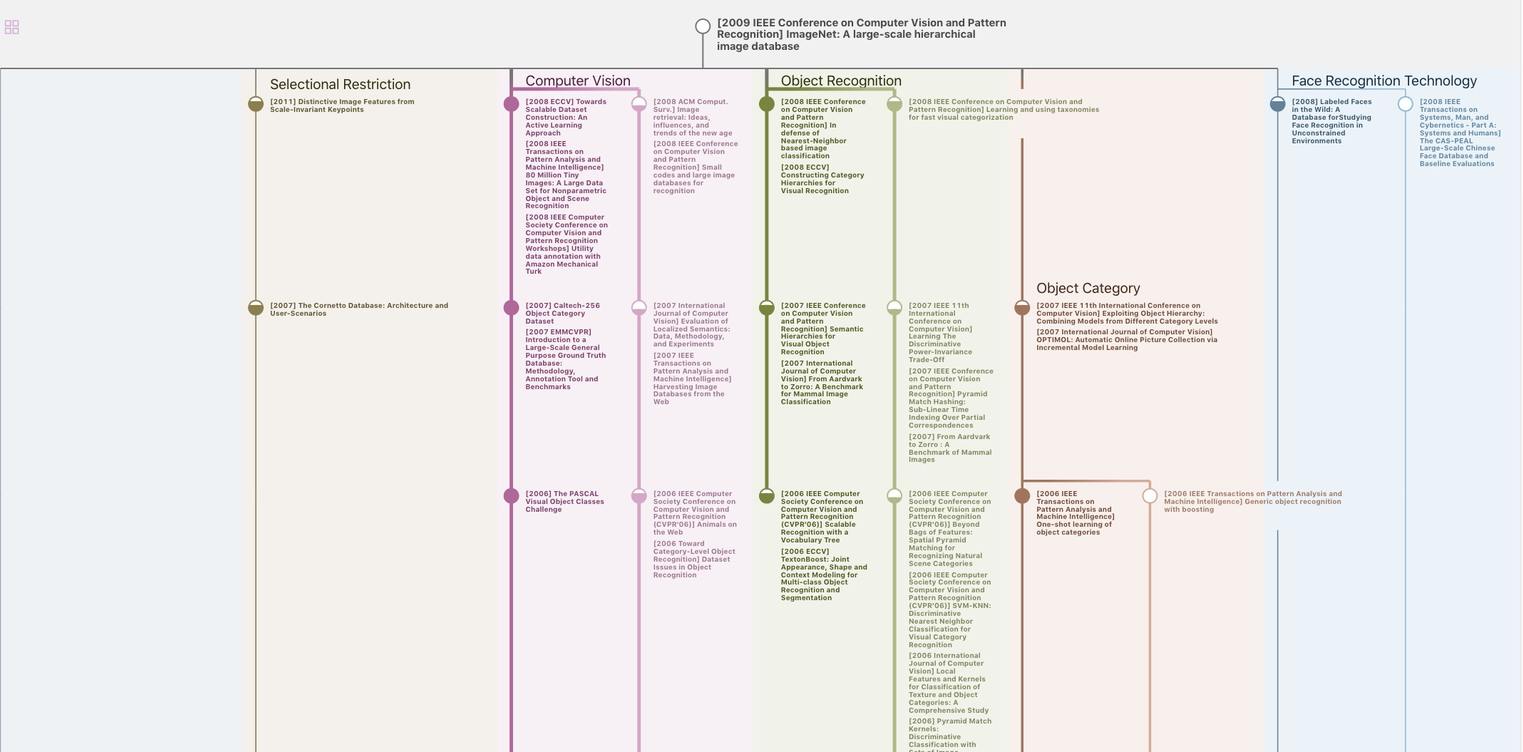
生成溯源树,研究论文发展脉络
Chat Paper
正在生成论文摘要