A Fused Context-Aware Neural Model for Item Recommendation
user-5f3206704c775e3a7964bd8b(2017)
摘要
The user reviews have been widely adopted for user rating prediction. However, the existing review-based methods mainly derive the latent features for the users and items in an independent and static manner. That is, a single static feature vector is used for a user to identify her preference on all candidate items without considering the particular characteristics of each item. In this paper, we propose a fused context-aware neural model for rating prediction by using reviews and rating scores simultaneously, named FCAN. FCAN consists of two learning components: context-based feature learning and interaction-based feature learning. In context-based feature learning, the dynamic user latent features and item latent features are extracted through the convolution operations and nonlinearities supercharged by the neural treatment. In this sense, the relevant features from both the user review and item review on the basis of the user-item pair are identified for rating prediction. Moreover, introducing context-based features increases interpretability in rating prediction. However, the features learnt in context-based learning component is only review-driven and may not be comprehensive. We further extract complementary latent features by using the user-item rating scores in interaction-based feature learning component. With a dynamic linear fusion mechanism, FCAN can better capture the user preference for rating prediction using both the reviews and the rating scores. Experiments on five real-world datasets show that FCAN achieves significantly better predication accuracy than state-of-the-art review-based alternatives.
更多查看译文
关键词
Feature learning,Feature vector,Context (language use),Interpretability,Machine learning,Component (UML),Computer science,Fusion mechanism,Basis (linear algebra),Preference,Artificial intelligence
AI 理解论文
溯源树
样例
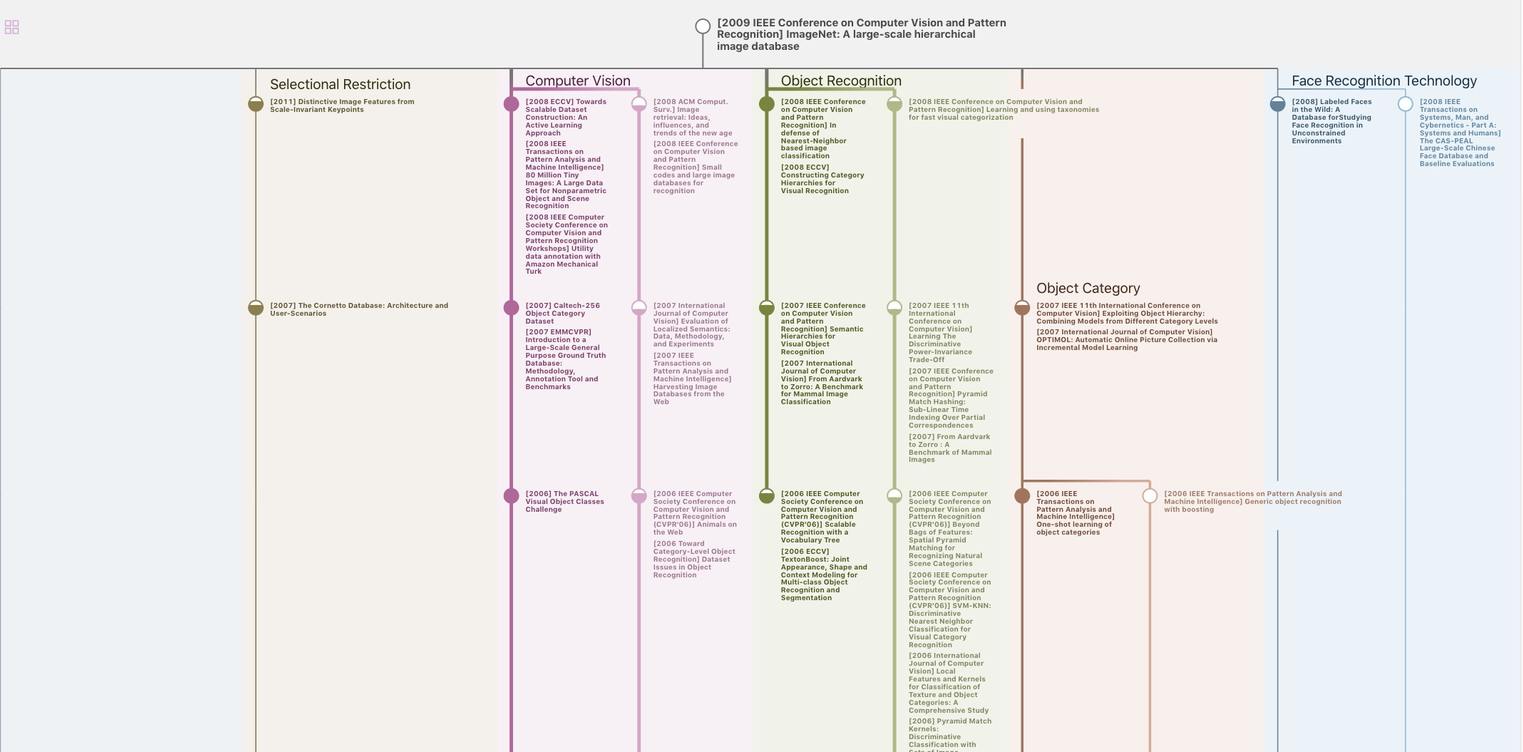
生成溯源树,研究论文发展脉络
Chat Paper
正在生成论文摘要