Locating Visual Explanations for Video Question Answering.
MMM (1)(2021)
摘要
Although promising performance has been reported for Video Question Answering (VideoQA) in recent years, there is still a large gap for human to truly understand the model decisions. Besides, beyond a short answer, complementary visual information is desirable to enhance and elucidate the content of QA pairs. To this end, we introduce a new task called Video Question Answering with Visual Explanations (VQA-VE), which requires to generate answers and provide visual explanations (i.e., locating relevant moments within the whole video) simultaneously. This task bridges video question answering and temporal localization. They are two separate and typical visual tasks and come with our challenge. For training and evaluation, we build a new dataset on top of ActivityNet Captions by annotating QA pairs with temporal ground-truth. We also adopt a large-scale benchmark TVQA. Towards VQA-VE, we develop a new model that is able to generate complete natural language sentences as answers while locating relevant moments with various time spans in a multi-task framework. We also introduce two metrics to fairly measure the performance on VQA-VE. Experimental results not only show the effectiveness of our model, but also demonstrate that additional supervision from visual explanations can improve the performance of models on traditional VideoQA task.
更多查看译文
关键词
Question answering,Task (project management),Natural language,Benchmark (computing),Natural language processing,Computer science,Measure (data warehouse),Artificial intelligence,Short answer
AI 理解论文
溯源树
样例
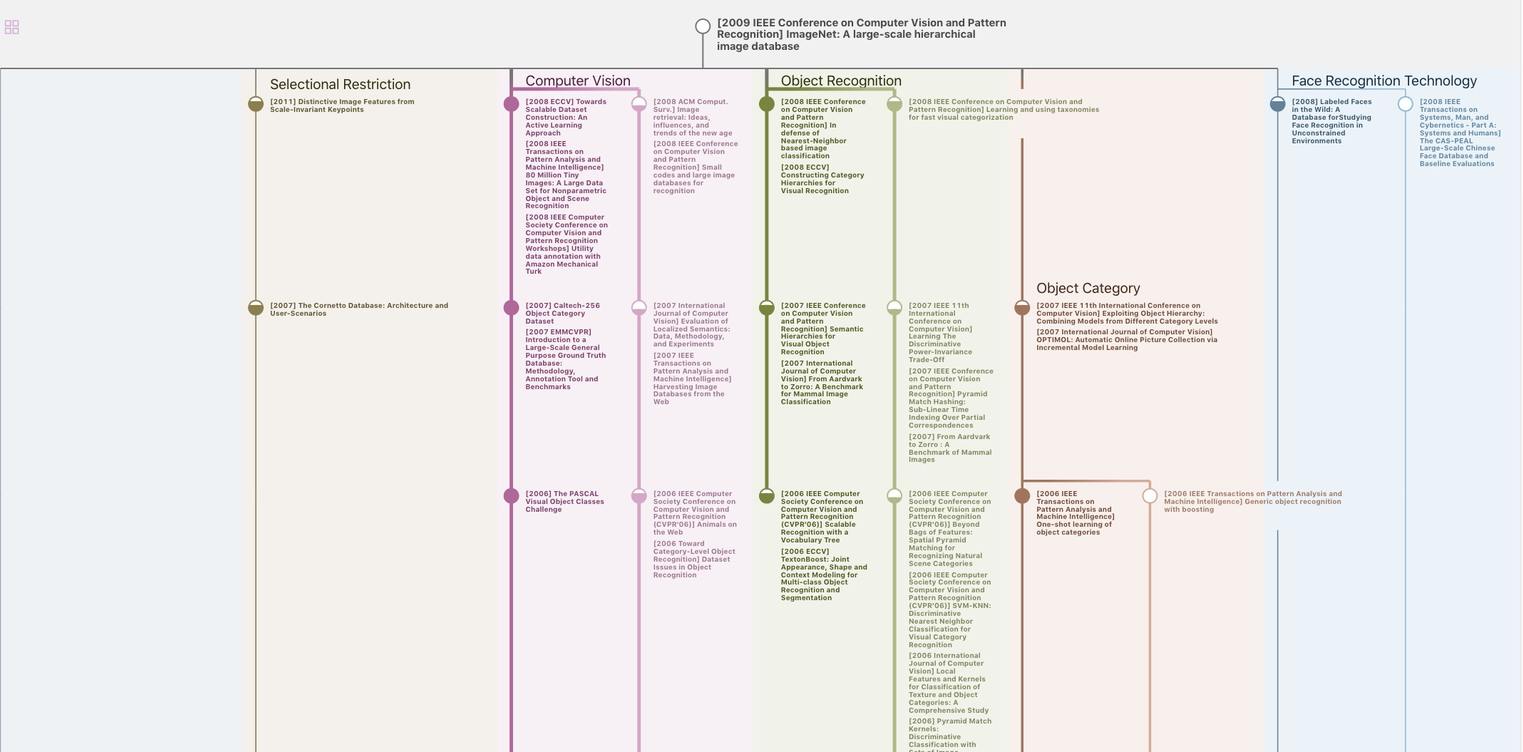
生成溯源树,研究论文发展脉络
Chat Paper
正在生成论文摘要