UNO: Virtualizing and Unifying Nonlinear Operations for Emerging Neural Networks
2021 IEEE/ACM International Symposium on Low Power Electronics and Design (ISLPED)(2021)
摘要
Linear multiply-accumulate (MAC) operations have been the main focus of prior efforts in improving the energy efficiency of neural network inference due to their dominant contribution to energy consumption in traditional models. On the other hand, nonlinear operations, such as division, exponentiation, and logarithm, that are becoming increasingly significant in emerging neural network models, have been largely underexplored. In this paper, we propose UNO, a low-area, low-energy processing element that virtualizes the Taylor approximation of nonlinear operations on top of off-the-shelf linear MAC units already present in inference hardware. Such virtualization approximates multiple nonlinear operations in a unified, MAC-compatible manner to achieve dynamic run-time accuracy-energy scaling. Compared to the baseline, our scheme reduces the energy consumption by up to 38.4% for individual operations and increases the energy efficiency by up to 274.5% for emerging neural network models with negligible inference loss
更多查看译文
关键词
dynamic run-time accuracy-energy scaling,virtualization,inference hardware,off-the-shelf linear MAC units,Taylor approximation,neural network models,energy consumption,linear multiply-accumulate operations,unifying nonlinear operations,UNO
AI 理解论文
溯源树
样例
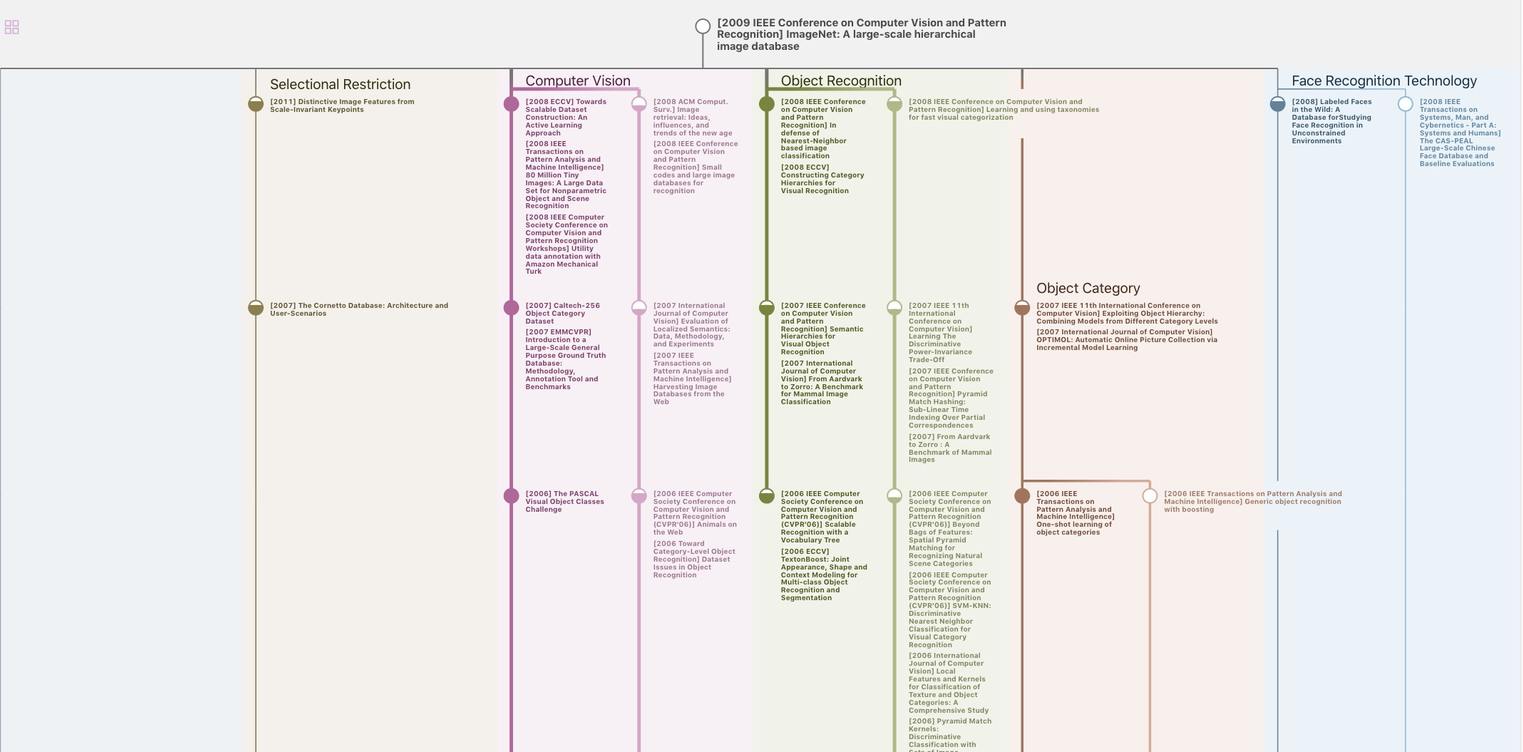
生成溯源树,研究论文发展脉络
Chat Paper
正在生成论文摘要