FARM: hierarchical association rule mining and visualization method
BCB(2021)
摘要
ABSTRACTAssociations search is one of the methods of data analysis. Association Rule Mining (ARM) approach can construct association rules from observational data, but the most widely used algorithm Apriori typically produces large number of unstructured results without any ranking or statistical significance. We propose a novel method for association rules mining FARM (Fishbone Association Rule Mining) to address these challenges. First of all, it is necessary to solve the problem with huge number of unstructured rules. It is important because large number of rules results in time costs for their investigation and absence of rule structure gives no information which features are more important. FARM uses hierarchical structure for rules producing which is helpful because priority of features became clearly visible. At each step FARM is trying to increase hierarchical rule complexity by adding additional features in such way that optimization metric (e.g., conviction) would grow. During this procedure it's also being checked that information growth is achieving. Further significance filtering is used to focus on statistically significant results. FARM involves check for statistical significance using hold-out approach which begins with splitting dataset into two parts - first for rules construction and second one for validation. Constructed rules are firstly filtered by chi-squared test, then validated and finally checked using statistical testing with multiple comparisons correction. At this point FARM obtains statistically significant hierarchical rules and they need to be shown in human readable way for which Ishikawa diagram is used. This diagram is based on the idea of causal-like hierarchy structure visualization with the fishbone head target and ordered predicates in the ribs, so it perfectly corresponds to our needs. Final rules are included in result diagrams and interactive filters provide FARM users with ability to set filters to show most significant rules, or rules with minimal required characteristics. Analysis can be run using dedicated web service what improves convenience for everyone who wants to try FARM. We applied FARM to previously published public datasets achieving rules which included original papers results. After that we used FARM in our recent paper [1] where we found associations between changes in methylome and regulatory regions in the genome. FARM has shown itself convenient in use and promising due to abilities in detecting significant rules and their apparent visualization. We believe that FARM will accelerate discoveries by producing complete solution for analysis and visualization of data patterns.
更多查看译文
AI 理解论文
溯源树
样例
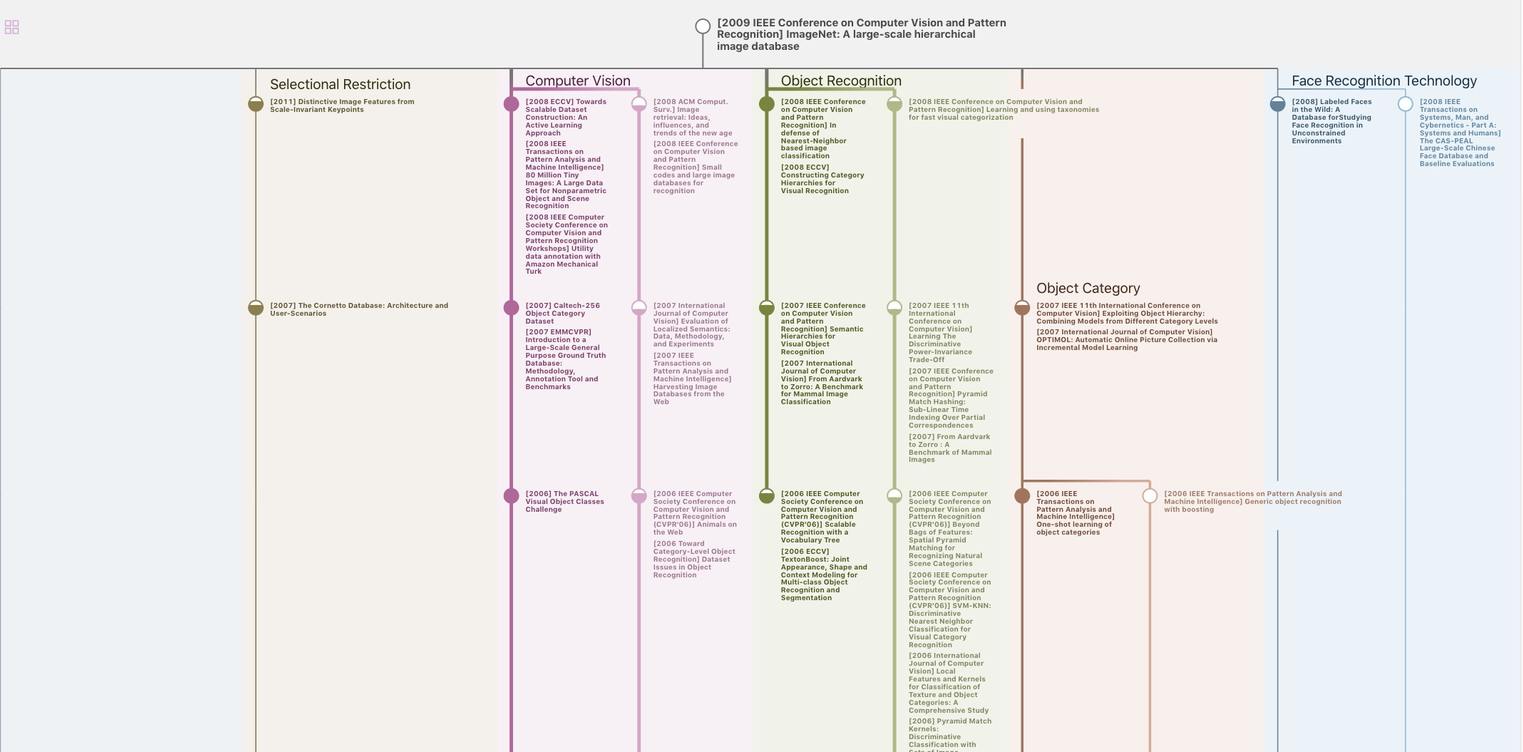
生成溯源树,研究论文发展脉络
Chat Paper
正在生成论文摘要