AW-Net: automatic muscle structure analysis on B-mode ultrasound images for injury prevention
BCB(2021)
摘要
ABSTRACTMuscle injuries have deep physical and mental impacts on athletic individuals. Not only are injuries a heavy setback for sportspersons as it reduces their practice time, but they also have significant after-math on their personal life [7, 17]. Knowing the individual's muscle state to adapt his practice to his needs and to prevent potential injuries is nowadays a high stake issue. Muscle geometry, referred to as muscle architecture is a crucial determinant of performance (i.e. muscle strength, power, shortening velocity). For a decade, it is proposed that hamstring muscle architecture (e.g. muscle fascicle length) may influence an individual's exposure to muscle injury [2]. B-mode ultrasound imaging is a non-invasive technique widely used to extract muscle properties by identifying the pennation angle and fascicle length in the images but requires time-consuming and prone to approximations manual post-processing of the images. This paper presents a fully automated method to analyze the muscle structure and properties from B-mode ultrasound images, more specifically by estimating the aponeurosis and fascicle architecture. In this study, we focus on segmenting aponeuroses and estimating a vector field modeling of the fascicle structure with a novel multi-task model, Attention W-Net (AW-Net), based on the U-Net architecture with attention gate. We enriched two public ultrasound datasets on lower leg muscles with aponeurosis annotation and made these modifications publicly available. Trained on these datasets, our model outperformed state-of-the-art methods and demonstrates precise estimation of the muscle structure and properties in a fully automated fashion. Additionally, by augmenting the training set with 288 proprietary ultrasound images of the semimembranous and biceps femoris muscles collected on athletic individuals and annotated with medical and sport science experts, our method generalizes to other muscles with further improved performance.
更多查看译文
关键词
ultrasound, neural networks, physical health, injury prevention, automatic medical image analysis
AI 理解论文
溯源树
样例
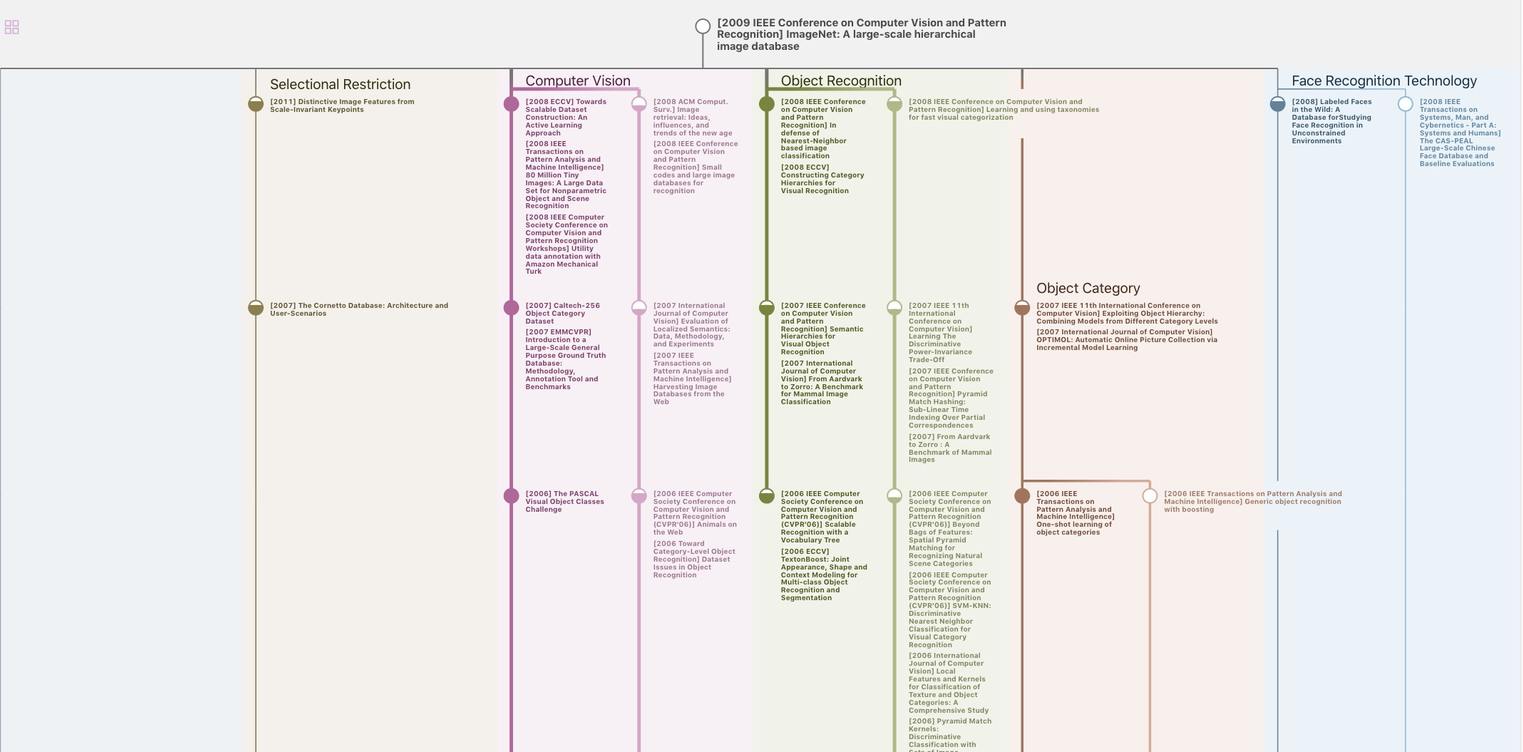
生成溯源树,研究论文发展脉络
Chat Paper
正在生成论文摘要