Segmenting thoracic cavities with neoplastic lesions: a head-to-head benchmark with fully convolutional neural networks
BCB(2021)
摘要
ABSTRACTAutomatic segmentation of thoracic cavity structures in computer tomography (CT) is a key step for applications ranging from radiotherapy planning to imaging biomarker discovery with radiomics approaches. State-of-the-art segmentation can be provided by fully convolutional neural networks such as the U-Net or V-Net. However, there is a very limited body of work on a comparative analysis of the performance of these architectures for chest CTs with significant neoplastic disease. In this work, we compared four different types of fully convolutional architectures using the same pre-processing and post-processing pipelines. These methods were evaluated using a dataset of CT images and thoracic cavity segmentations from 402 cancer patients. We found that these methods achieved very high segmentation performance by benchmarks of three evaluation criteria, i.e. Dice coefficient, average symmetric surface distance and 95% Hausdorff distance. Overall, the two-stage 3D U-Net model performed slightly better than other models, with Dice coefficients for left and right lung reaching 0.947 and 0.952, respectively. However, 3D U-Net model achieved the best performance under the evaluation of HD95 for right lung and ASSD for both left and right lung. These results demonstrate that the current state-of-art deep learning models can work very well for segmenting not only healthy lungs but also the lung containing different stages of cancerous lesions. The comprehensive types of lung masks from these evaluated methods enabled the creation of imaging-based biomarkers representing both healthy lung parenchyma and neoplastic lesions, allowing us to utilize these segmented areas for the downstream analysis, e.g. treatment planning, prognosis and survival prediction.
更多查看译文
关键词
Lungs with Cancer, segmentation, Fully Convolutional Neural Network
AI 理解论文
溯源树
样例
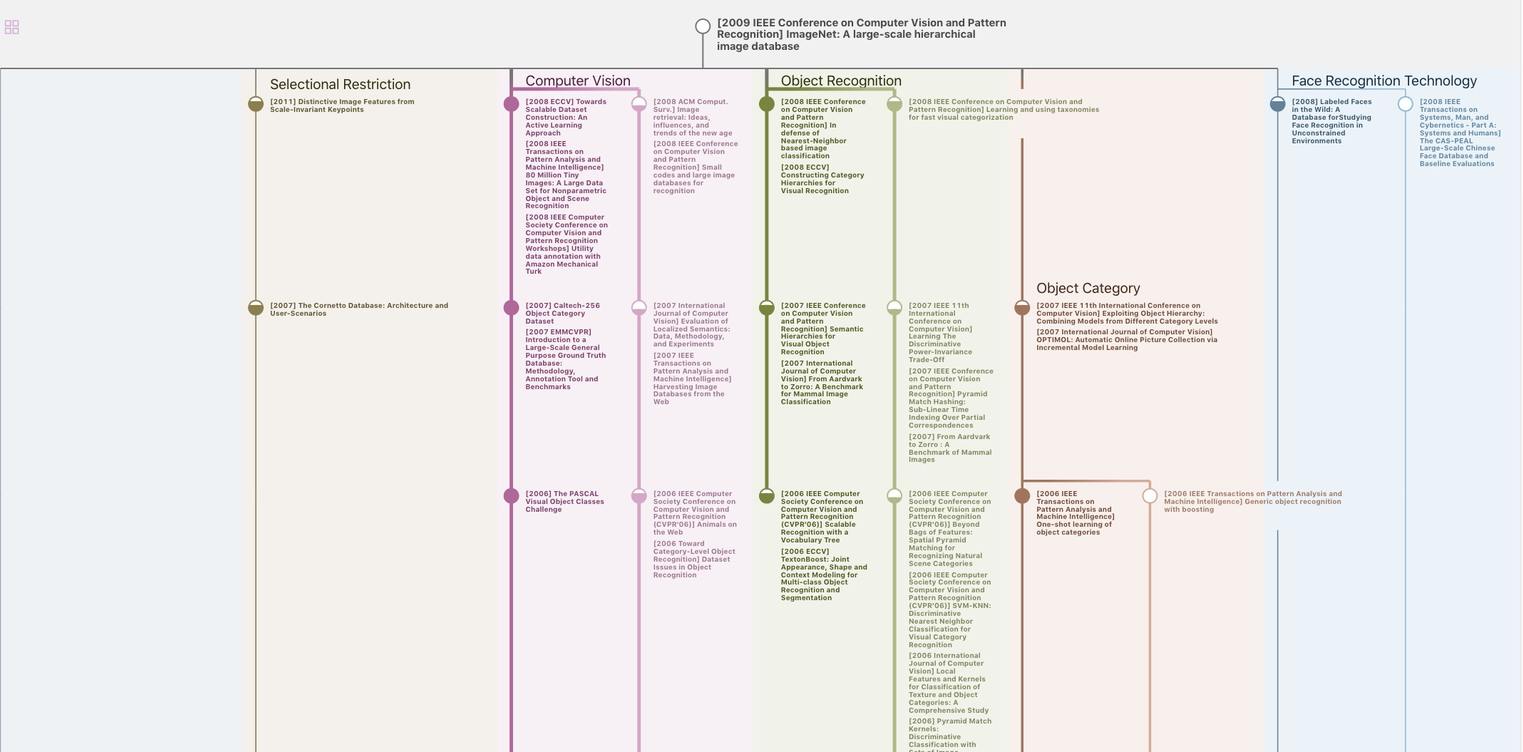
生成溯源树,研究论文发展脉络
Chat Paper
正在生成论文摘要