Perceptually Discriminating the Highest Priority Alarms Reduces Response Time: A Retrospective Pre-Post Study at Four Hospitals
Human factors(2023)
摘要
Objective Reduce nurse response time for emergency and high-priority alarms by increasing discriminability between emergency and all other alarms and suppressing redundant and likely false high-priority alarms in a secondary alarm notification system (SANS). Background Emergency alarms are the most urgent, requiring immediate action to address a dangerous situation. They are clinician-triggered and have higher positive predictive value (PPV). High-priority alarms are automatically triggered and have lower PPV. Method We performed a retrospective pre-post study, analyzing data 15 months before and 25 months after a SANS redesign was implemented in four hospitals. For emergency alarms, we incorporated digitized human speech to distinguish them from automatically triggered alarms, leaving their onset and escalation pathways unchanged. For automatically triggered alarms, we suppressed some by delaying initial onset and escalation by 20 s. We used linear mixed models to assess the change in response time, Fisher's exact test for the proportion of response times longer than 120 s, and control charts for process stability. Results Response time for emergency alarms decreased at all hospitals (main, from 26.91 s to 22.32 s, p < .001; cardiac, from 127.10 s to 52.43 s, p < .001; cancer, from 18.03 s to 15.39 s, p < .001). Improvements were sustained. Automatically triggered alarms decreased 25.0%. Response time for the three automatically triggered cardiac alarms increased at the four hospitals. Conclusion Auditory sound disambiguation was associated with a sustained reduced nurse response time for emergency alarms, but suppressing some high-priority automatically triggered alarms was not. Application Distinguishing and escalating urgent, actionable alarms with higher PPV improves response time.
更多查看译文
关键词
alarms,automation,clinical,decision support systems,decision-making,health,information technology,medical devices
AI 理解论文
溯源树
样例
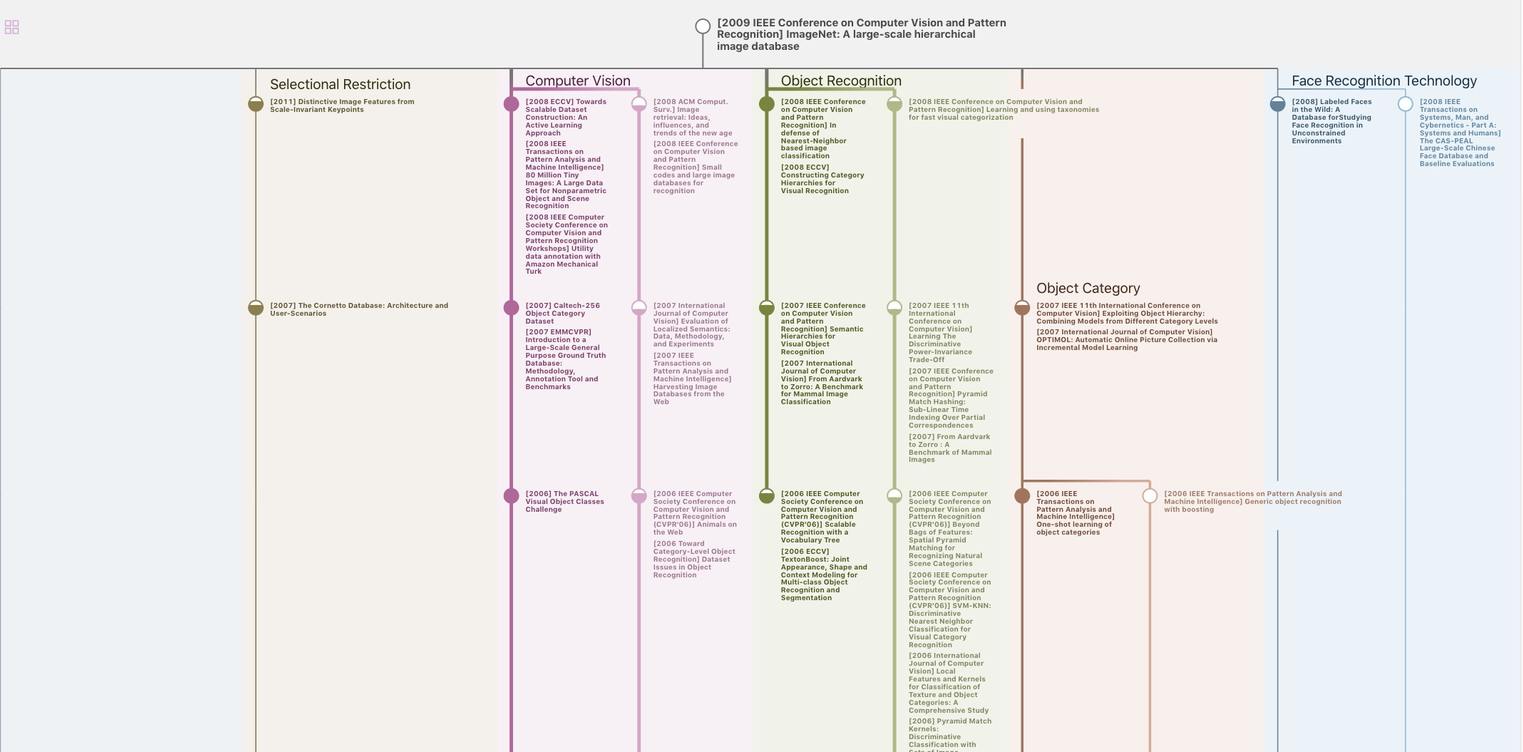
生成溯源树,研究论文发展脉络
Chat Paper
正在生成论文摘要