Neuromorphic scaling advantages for energy-efficient random walk computations
NATURE ELECTRONICS(2022)
摘要
Neuromorphic computing, which aims to replicate the computational structure and architecture of the brain in synthetic hardware, has typically focused on artificial intelligence applications. What is less explored is whether such brain-inspired hardware can provide value beyond cognitive tasks. Here we show that the high degree of parallelism and configurability of spiking neuromorphic architectures makes them well suited to implement random walks via discrete-time Markov chains. These random walks are useful in Monte Carlo methods, which represent a fundamental computational tool for solving a wide range of numerical computing tasks. Using IBM’s TrueNorth and Intel’s Loihi neuromorphic computing platforms, we show that our neuromorphic computing algorithm for generating random walk approximations of diffusion offers advantages in energy-efficient computation compared with conventional approaches. We also show that our neuromorphic computing algorithm can be extended to more sophisticated jump-diffusion processes that are useful in a range of applications, including financial economics, particle physics and machine learning.
更多查看译文
关键词
neuromorphic scaling advantages,walk,energy-efficient
AI 理解论文
溯源树
样例
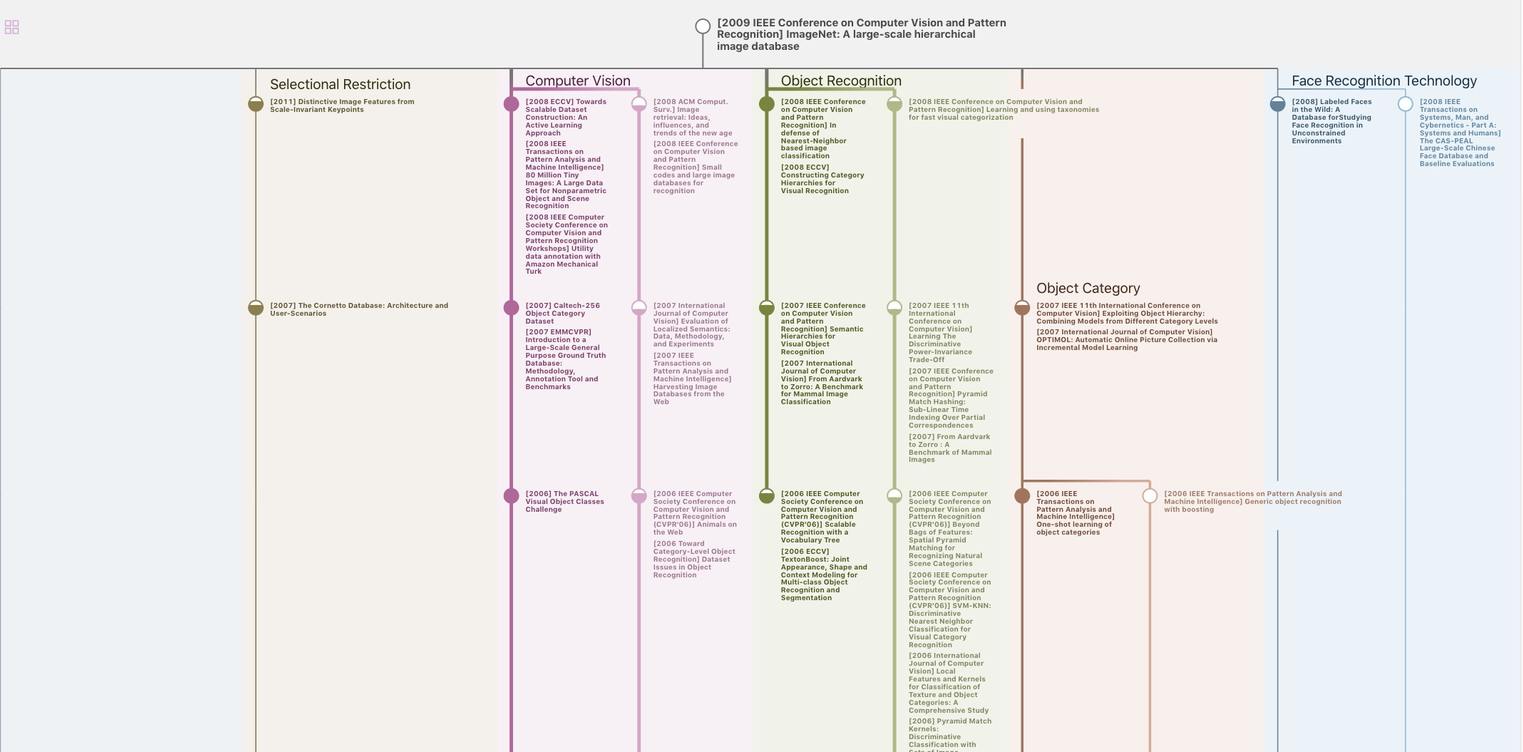
生成溯源树,研究论文发展脉络
Chat Paper
正在生成论文摘要