Multimodal Classification: Current Landscape, Taxonomy and Future Directions
ACM Computing Surveys(2023)
摘要
AbstractMultimodal classification research has been gaining popularity with new datasets in domains such as satellite imagery, biometrics, and medicine. Prior research has shown the benefits of combining data from multiple sources compared to traditional unimodal data that has led to the development of many novel multimodal architectures. However, the lack of consistent terminologies and architectural descriptions makes it difficult to compare different solutions. We address these challenges by proposing a new taxonomy for describing multimodal classification models based on trends found in recent publications. Examples of how this taxonomy could be applied to existing models are presented as well as a checklist to aid in the clear and complete presentation of future models. Many of the most difficult aspects of unimodal classification have not yet been fully addressed for multimodal datasets, including big data, class imbalance, and instance-level difficulty. We also provide a discussion of these challenges and future directions of research.
更多查看译文
关键词
Multimodal learning,neural networks,machine learning,classification
AI 理解论文
溯源树
样例
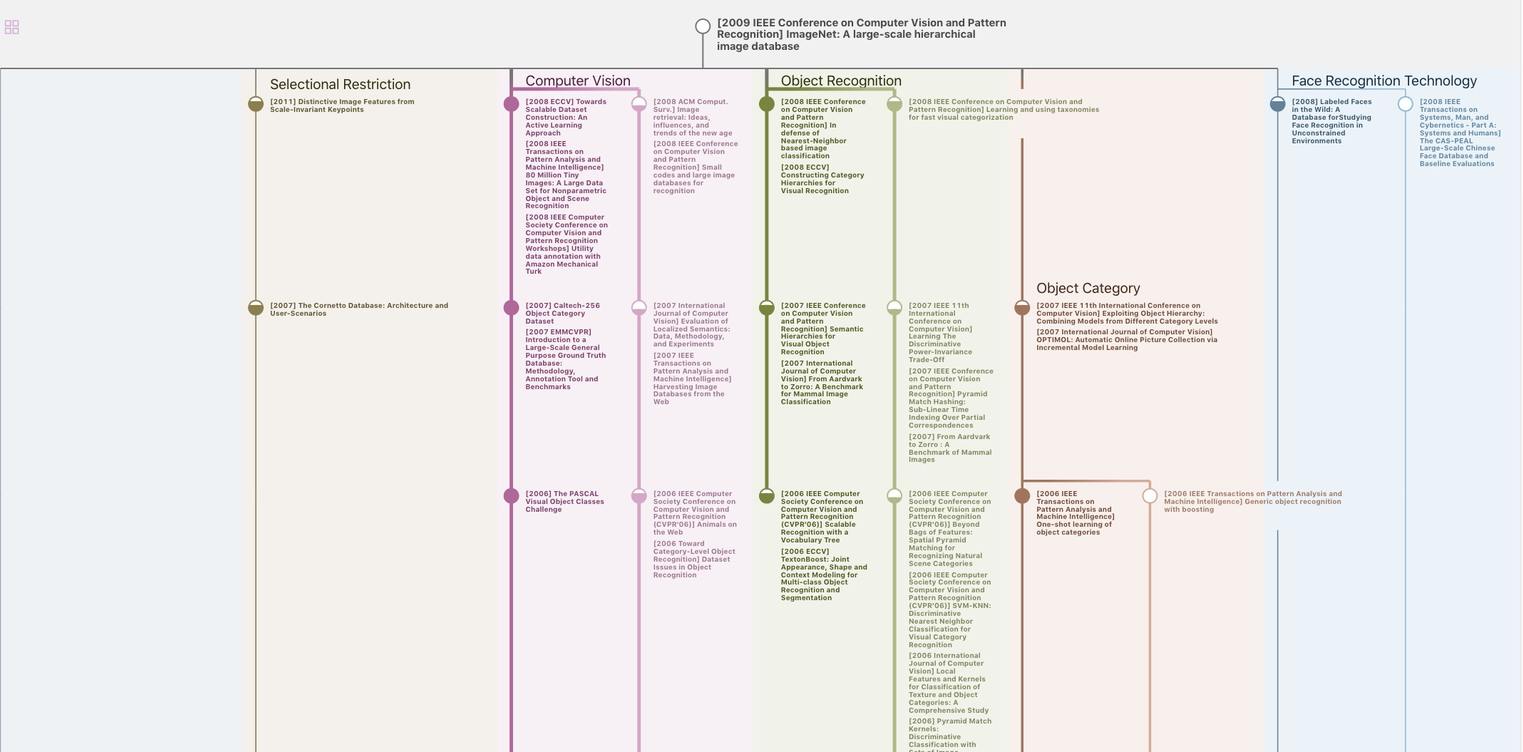
生成溯源树,研究论文发展脉络
Chat Paper
正在生成论文摘要