Analysis of Clustering Algorithms to Clean and Normalize Early Modern European Book Titles.
ICSIM(2021)
摘要
In this paper, we identify the most accurate method of clustering to deduplicate the past centuries book records from multiple libraries for data analysis out of five common algorithms. The presence of duplicate records is a major concern in data analysis. The dataset we studied contains over 5 million records of books published in European languages between 1500 and 1800 in the Machine-Readable Cataloging (MARC) data format from 17,983 libraries in 123 countries. However, each book record was archived by the library owning it. This creates a consistency problem in which the same book was archived in a slightly different way between libraries. Moreover, the change in geography and language over the past centuries also affects data consistency regarding the name of a person and place. Many slightly different names represent the same record. Analyzing such a dataset without proper cleaning will misrepresent the result. Due to the size of the dataset and unknown number of duplicate records with variation, it is impractical to create a lookup table to replace each record. To solve this problem, we use data clustering to deduplicate this dataset. Our work is informed by scholarship on European History and the History of the Book. We find that clustering is an effective method for detecting the slight differences in records caused by the above-mentioned cataloging inconsistencies. Our foundation was experimentation with several candidate clustering methods on a test dataset. The test dataset was prepared by corrupting a clean dataset according to the same characteristics found in the whole dataset. The clean dataset contains roughly 1,000 random records in English, German, French, and Latin with approximately the same language distribution and average record lengths as the whole dataset. Our evaluation reveals that some clustering algorithms can achieve accuracy up to 0.97072. The clustering techniques perform well on the dataset we studied as demonstrated in this paper.
更多查看译文
AI 理解论文
溯源树
样例
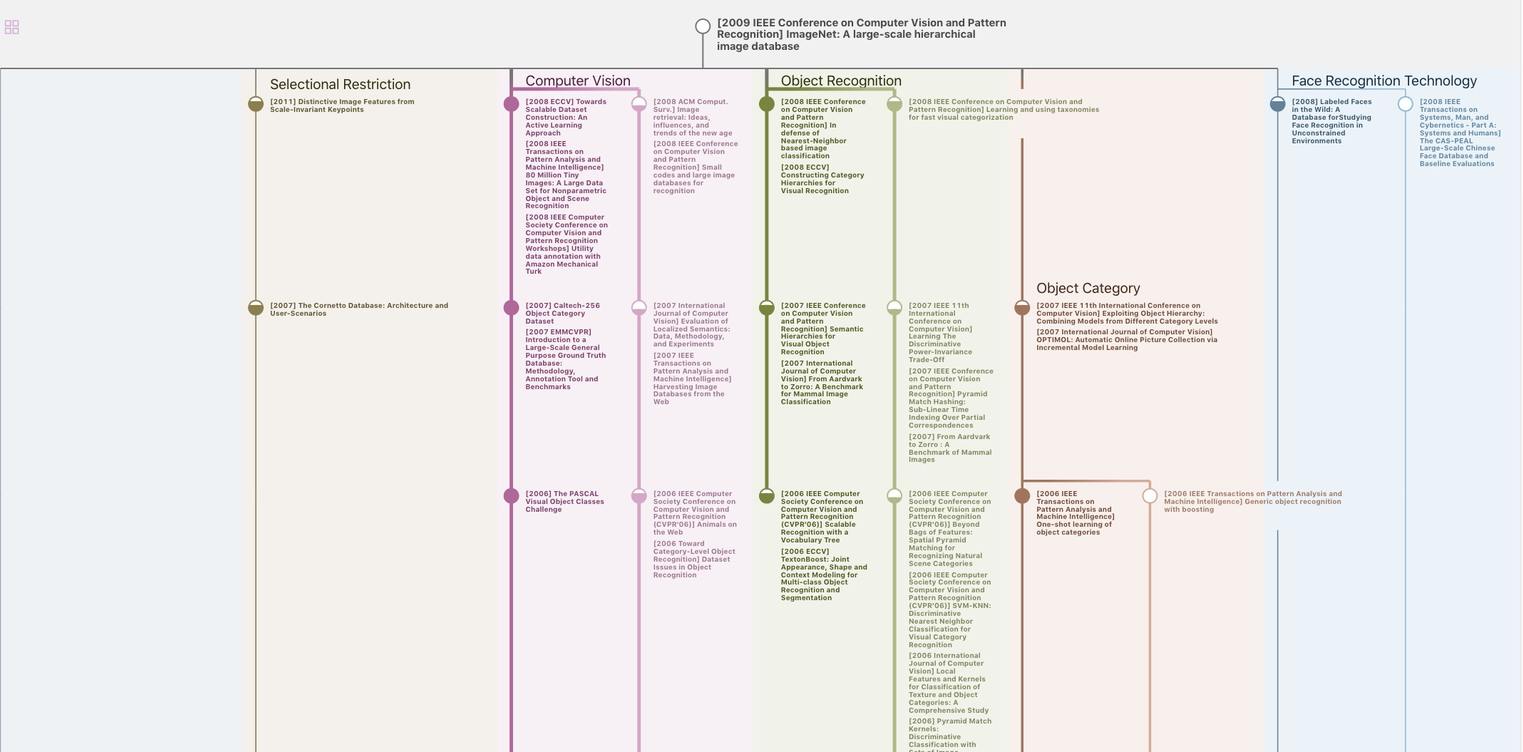
生成溯源树,研究论文发展脉络
Chat Paper
正在生成论文摘要