Coordinated Hyper-Parameter Search for Edge Machine Learning in Beyond-5G Networks.
ICC Workshops(2021)
摘要
Edge intelligence in radio access network (RAN) is an emerging concept wherein machine learning (ML) driven self-organizing network (SON) functions reside in edge nodes or base stations (BSs). This allows to fulfill ultra-low latency, scalability, ultra-high reliability and signaling needs and requirements for beyond-5G applications. However, the resource-intensive hyper-parameter search of edge-distributed ML workloads is a major challenge that undermines the gains of ML driven automated networks. In current edge-ML, resource-constraints force the ML driven SON functions to incline towards inference-only workloads at the edge, whereas their training is done in the central infrastructure in non-collaborative fashion. This requires a redundant transfer of big data for training from the edge to the central location and a hyper-parameter search with high space time complexity, eventually resulting in high signaling costs and delays. This paper aims to address these challenges by presenting the Coordinated HypEr-paramETer seArcH (CHEETAH) framework for edge intelligence. It is a resource-efficient, low time-complexity distributed framework that eliminates the transfer of large training data from the edge to the central location and reduces the time required to find optimal (or near-optimal) hyper-parameters. Using a traffic forecasting use-case based on real network data, we demonstrate that as compared to state-of-the-art, the CHEETAH framework offers an attractive pragmatic solution in terms of predicting the best hyper-parameter configuration while keeping the associated costs low.
更多查看译文
关键词
Edge Machine learning,5G,SON,Hyper-Parameter Search
AI 理解论文
溯源树
样例
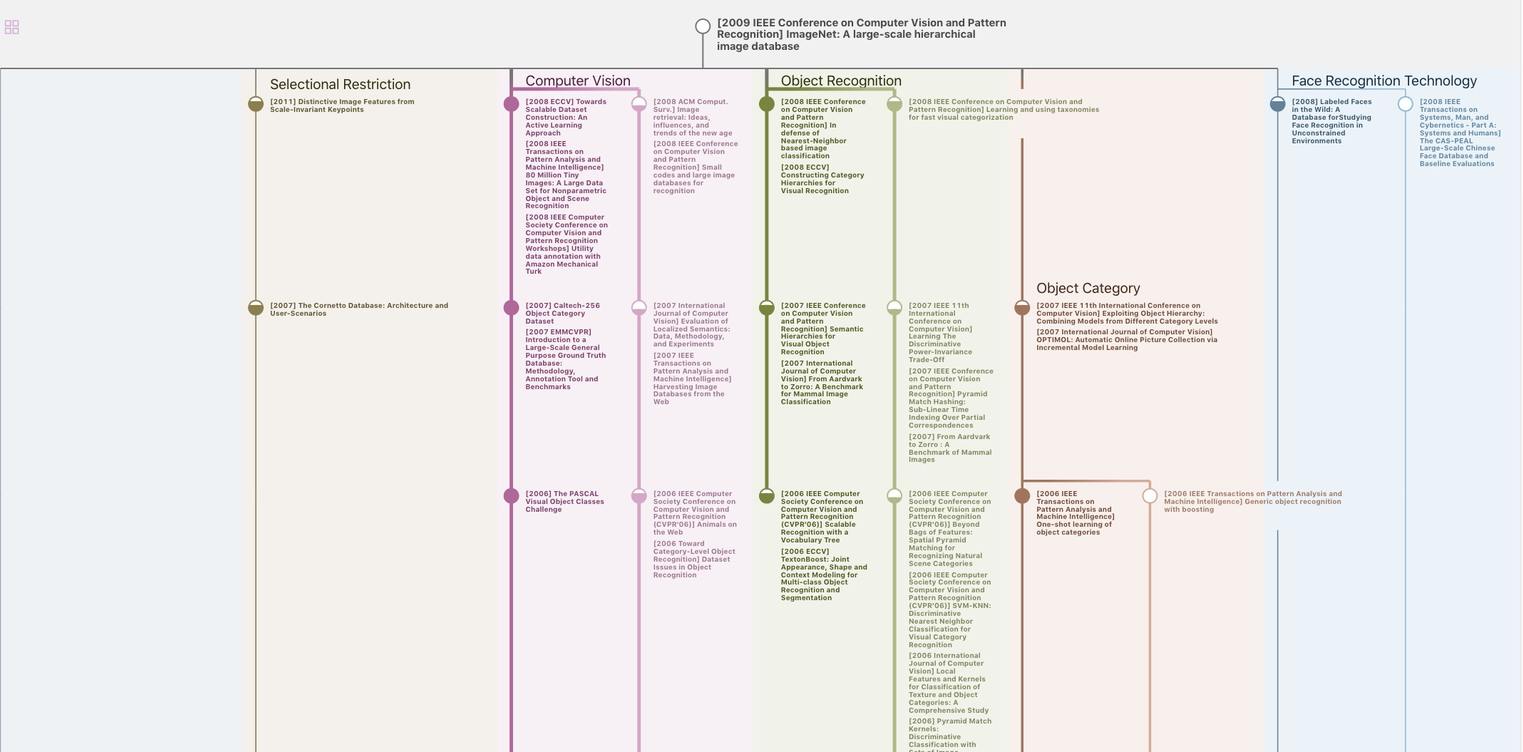
生成溯源树,研究论文发展脉络
Chat Paper
正在生成论文摘要