Domain-Irrelevant Representation Learning for Unsupervised Domain Generalization
arxiv(2021)
摘要
Domain generalization (DG) aims to help models trained on a set of source domains generalize better on unseen target domains. The performances of current DG methods largely rely on sufficient labeled data, which however are usually costly or unavailable. While unlabeled data are far more accessible, we seek to explore how unsupervised learning can help deep models generalizes across domains. Specifically, we study a novel generalization problem called unsupervised domain generalization, which aims to learn generalizable models with unlabeled data. Furthermore, we propose a Domain-Irrelevant Unsupervised Learning (DIUL) method to cope with the significant and misleading heterogeneity within unlabeled data and severe distribution shifts between source and target data. Surprisingly we observe that DIUL can not only counterbalance the scarcity of labeled data but also further strengthen the generalization ability of models when the labeled data are sufficient. As a pretraining approach, DIUL shows superior to ImageNet pretraining protocol even when the available data are unlabeled and of a greatly smaller amount compared to ImageNet. Extensive experiments clearly demonstrate the effectiveness of our method compared with state-of-the-art unsupervised learning counterparts.
更多查看译文
关键词
unsupervised domain-irrelevant generalization,learning
AI 理解论文
溯源树
样例
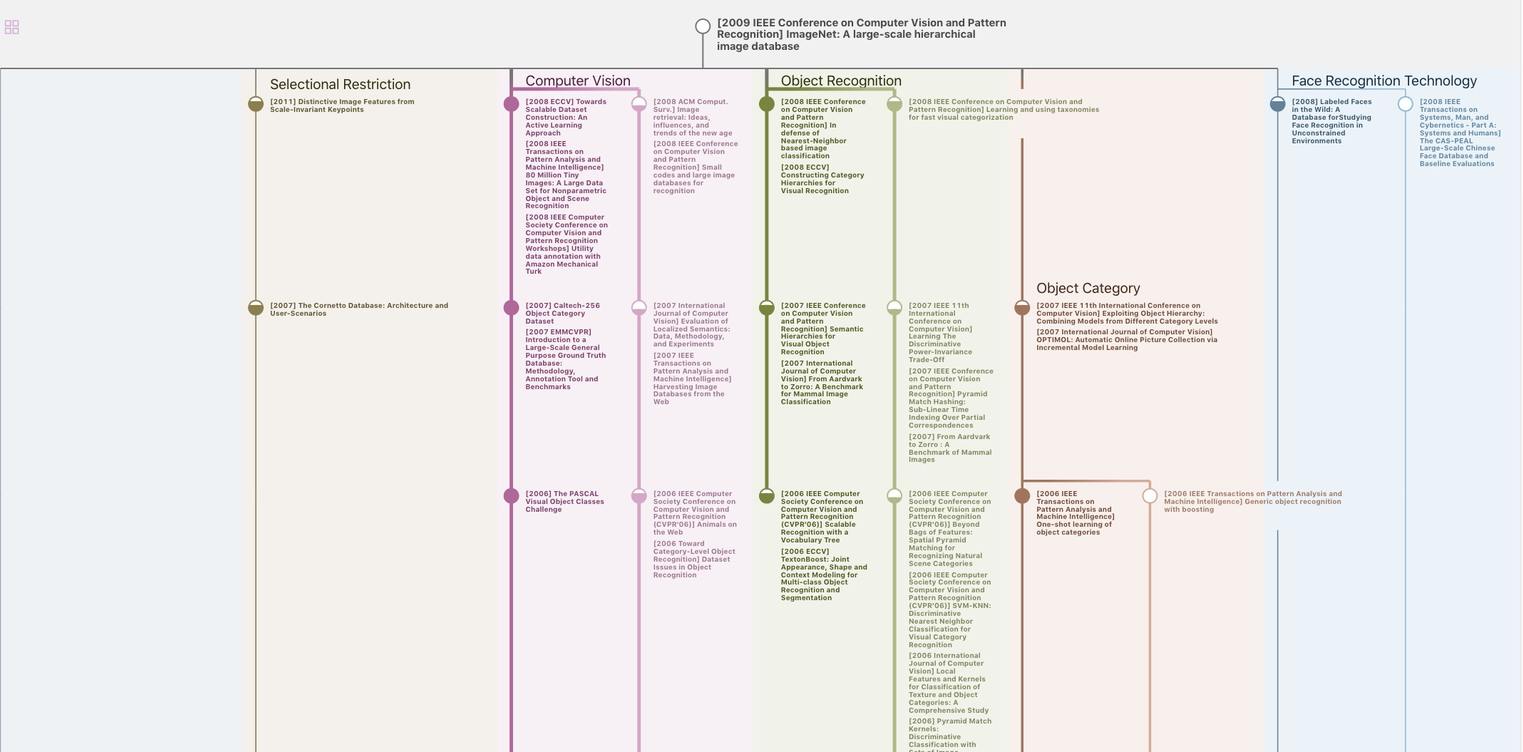
生成溯源树,研究论文发展脉络
Chat Paper
正在生成论文摘要