Leveraging Explainability for Comprehending Referring Expressions in the Real World
arxiv(2021)
摘要
For effective human-robot collaboration, it is crucial for robots to understand requests from users and ask reasonable follow-up questions when there are ambiguities. While comprehending the users' object descriptions in the requests, existing studies have focused on this challenge for limited object categories that can be detected or localized with existing object detection and localization modules. On the other hand, in the wild, it is impossible to limit the object categories that can be encountered during the interaction. To understand described objects and resolve ambiguities in the wild, for the first time, we suggest a method by leveraging explainability. Our method focuses on the active regions of a scene to find the described objects without putting the previous constraints on object categories and natural language instructions. We evaluate our method in varied real-world images and observe that the regions suggested by our method can help resolve ambiguities. When we compare our method with a state-of-the-art baseline, we show that our method performs better in scenes with ambiguous objects which cannot be recognized by existing object detectors.
更多查看译文
关键词
comprehending referring expressions,explainability
AI 理解论文
溯源树
样例
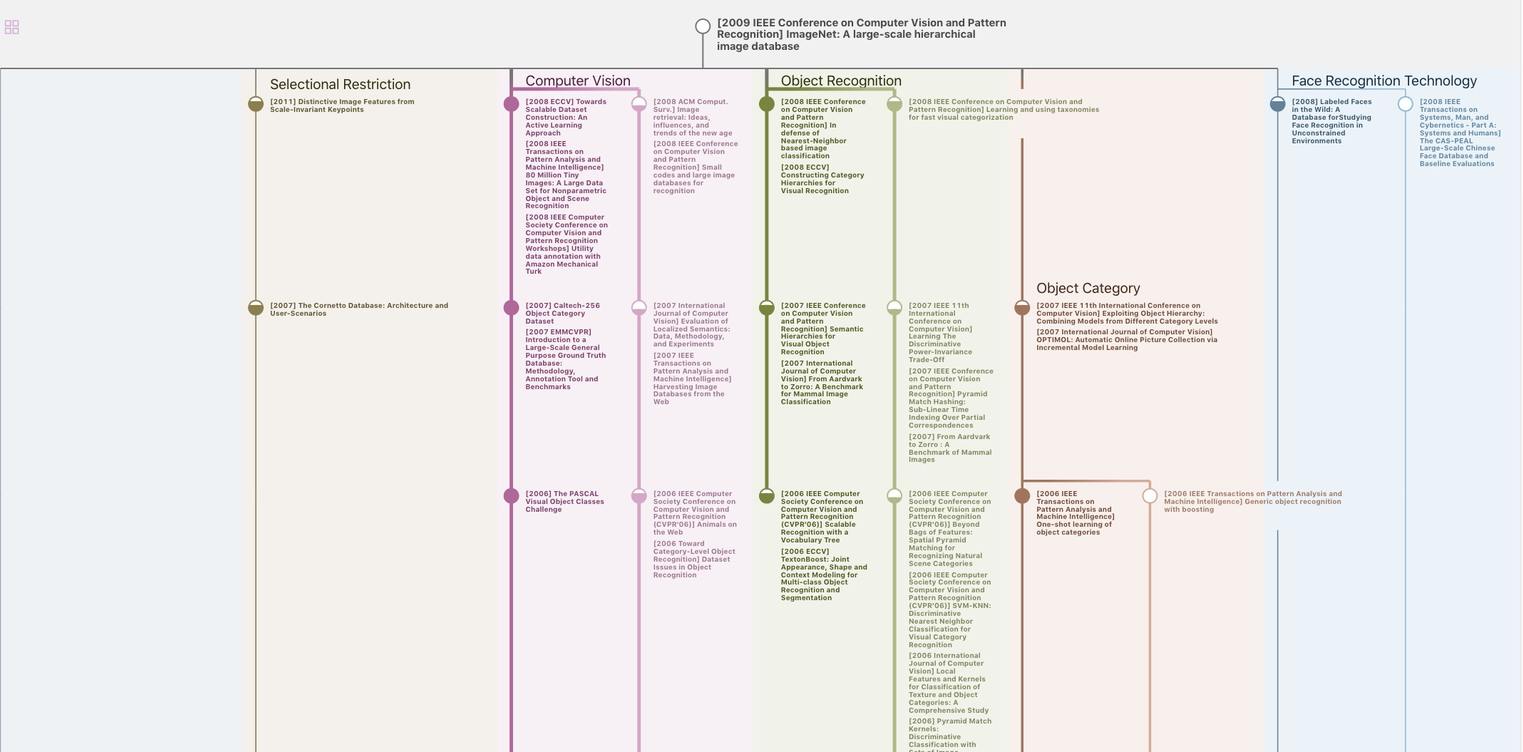
生成溯源树,研究论文发展脉络
Chat Paper
正在生成论文摘要