Improving a neural network model by explanation-guided training for glioma classification based on MRI data
arxiv(2023)
摘要
In recent years, artificial intelligence systems have come to the forefront. These systems, mostly based on deep learning, achieve excellent results in areas such as image processing, natural language processing and speech recognition. Despite the statistically high accuracy of deep learning models, their output is often based on ”black box” decisions. Thus, interpretability methods (Reyes et al. in Radiol Artif Intell 2(3):e190043, 2020) have become a popular way to gain insight into the decision-making process of deep learning models (Miller in Artif Intell 267:1–38, 2019). Explanation of deep learning models is desirable in the medical domain since experts have to justify their judgments to the patients. In this work, we proposed a method for explanation-guided training that uses a layer-wise relevance propagation technique to force the model to focus only on the relevant part of the image. We experimentally verified our method on a convolutional neural network model for low-grade and high-grade glioma classification problems. Our experiments produced promising results in the way where we use interpretation techniques in the training process.
更多查看译文
关键词
Explainable artificial intelligence,Deep neural networks,Medical imaging,Explanation-guided training
AI 理解论文
溯源树
样例
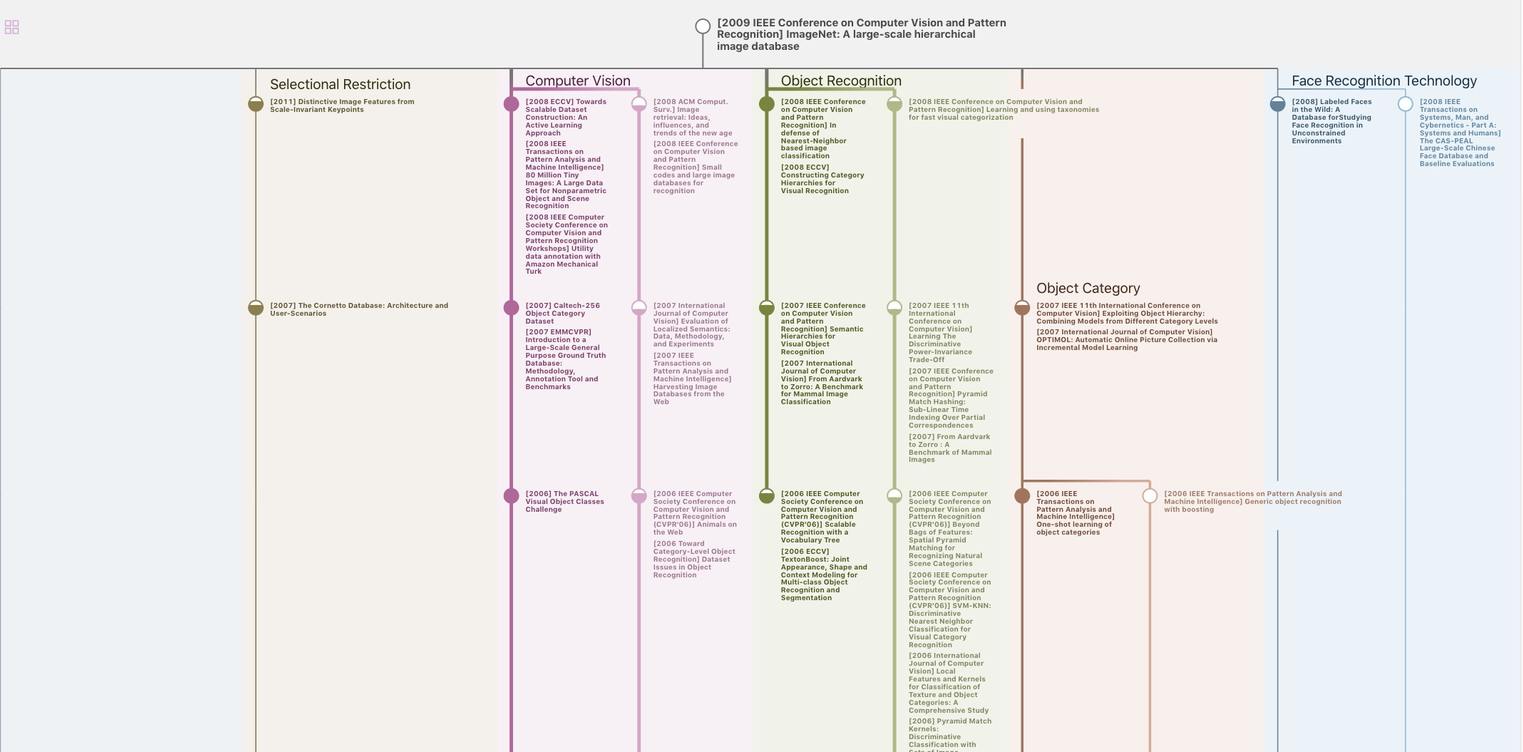
生成溯源树,研究论文发展脉络
Chat Paper
正在生成论文摘要