Lowering post-construction yield assessment uncertainty through better wind plant power curves
WIND ENERGY(2022)
摘要
Many operational analyses of wind power plants require a statistical relationship, which can be called the wind plant power curve, to be developed between wind plant energy production and concurrent atmospheric variables. Currently, a univariate linear regression at monthly resolution is the industry standard for post-construction yield assessments. Here, we evaluate the benefits in augmenting this conventional approach by testing alternative regressions performed with multiple inputs, at a finer time resolution, and using nonlinear machine-learning algorithms. We utilize the National Renewable Energy Laboratory's open-source software package OpenOA to assess wind plant power curves for 10 wind plants. When a univariate generalized additive model at daily or hourly resolution is used, regression uncertainty is reduced, in absolute terms, by up to 1.0% and 1.2% (corresponding to a -59% and -80% relative change), respectively, compared to a univariate linear regression at monthly resolution; also, a more accurate assessment of the mean long-term wind plant production is achieved. Additional input variables also reduce the regression uncertainty: when temperature is added as an input to the conventional monthly linear regression, the operational analysis uncertainty connected to regression is reduced, in absolute terms, by up to 0.5% (-43% relative change) for wind power plants with strong seasonal variability. Adding input variables to the machine-learning model at daily resolution can further reduce regression uncertainty, with up to a -10% relative change. Based on these results, we conclude that a multivariate nonlinear regression at daily or hourly resolution should be recommended for assessing wind plant power curves.
更多查看译文
关键词
machine learning, operational analysis, post constriction yield assessment, uncertainty
AI 理解论文
溯源树
样例
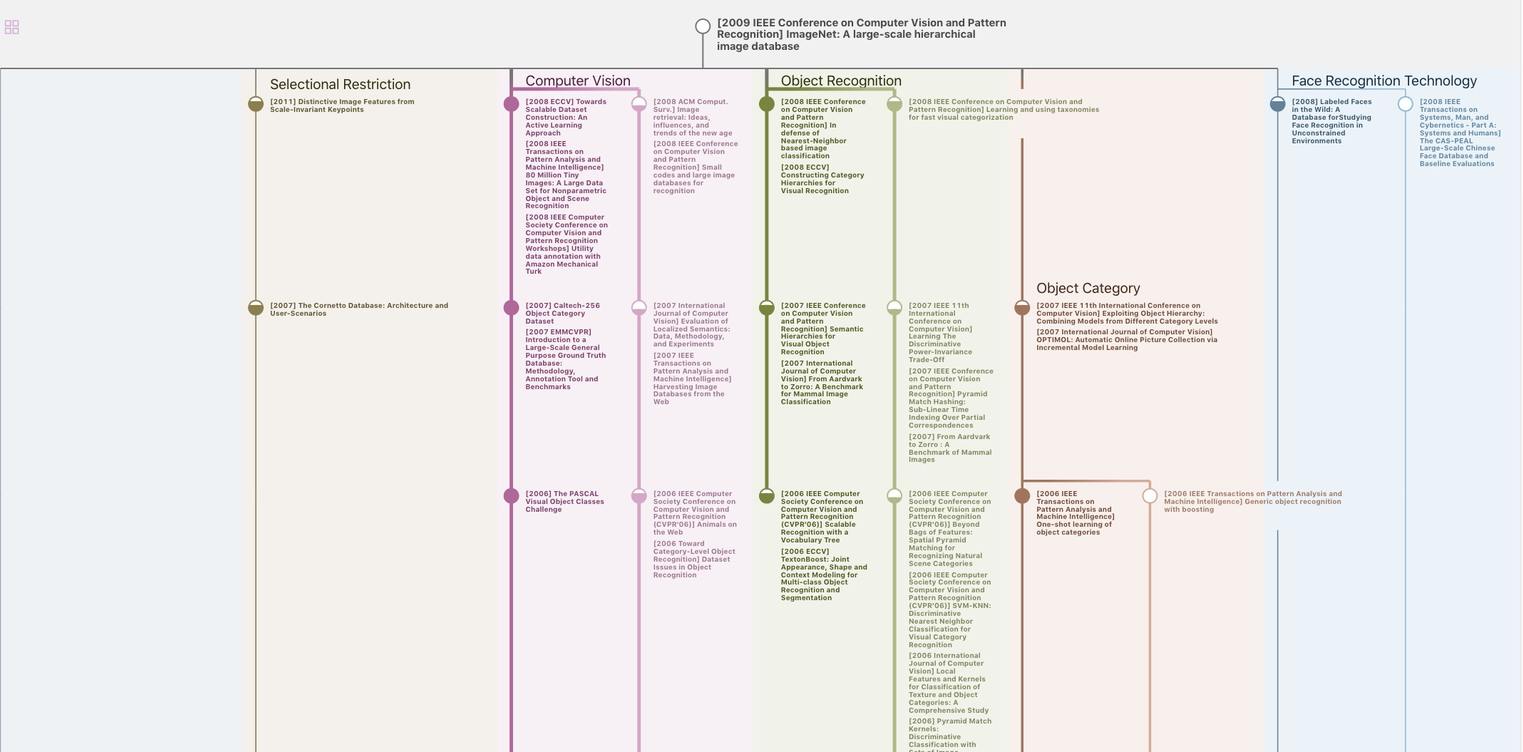
生成溯源树,研究论文发展脉络
Chat Paper
正在生成论文摘要