Multiscale Conditional Relationship Graph Network for Referring Relationships in Images
IEEE Transactions on Cognitive and Developmental Systems(2022)
摘要
Images contain not only individual entities but also abundant visual relationships between entities. Therefore, conditioned on visual relationship triples
${< }subject-relationship-object{>}$
that can be viewed as structured texts, entities (subjects or objects) can be localized in images without ambiguity. However, it is challenging to efficiently model visual relationships since a specific relationship usually has dramatic intraclass visual differences when involved with different entities, quite a number of which are in a small scale. In addition, the subject and the object in a relationship triple may have different best scales, and matching the subject and the object with different appropriate scales may improve prediction. To address these issues, a multiscale conditional relationship graph network (CRGN) is proposed in this article to localize entities based on visual relationships. Specifically, an attention pyramid network is first introduced to generate multiscale attention maps to capture entities with various sizes for entity matching. Then, a CRGN is further designed to aggregate and refine multiscale attention features to localize entities via passing relationship contexts between entity attention maps, which sufficiently utilizes the entity attention maps with the best scales. In order to mitigate the negative effects of intraclass visual differences of relationships, vision-agnostic relationship features are utilized in the proposed CRGN to indirectly model relationship contexts. The experiments demonstrate the superiority of the proposed method compared with the previous powerful frameworks on three challenging benchmark data sets, including CLEVR, Visual Genome, and VRD. The project page can be found in
https://mic.tongji.edu.cn/d9/5c/c9778a186716/page.htm
.
更多查看译文
关键词
Conditional relationship,graph neural network (GNN),multiscale features,referring relationships
AI 理解论文
溯源树
样例
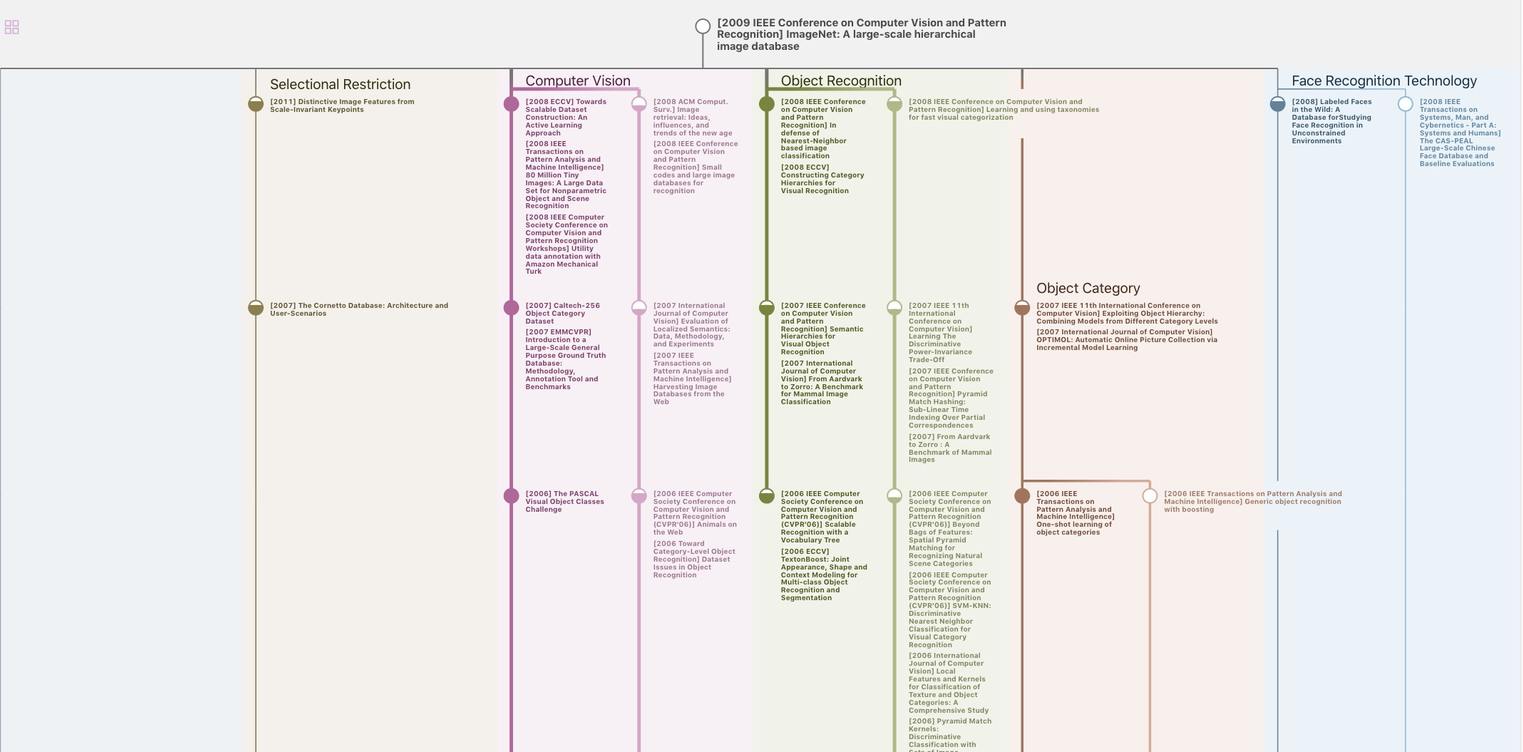
生成溯源树,研究论文发展脉络
Chat Paper
正在生成论文摘要