A Bag of Tricks for Automated De Novo Design of Molecules with the Desired Properties: Application to EGFR Inhibitor Discovery
ChemRxiv(2021)
摘要
Deep generative neural networks have been used increasingly
in computational chemistry for de novo design of molecules with desired
properties. Many deep learning approaches employ reinforcement
learning for optimizing the target properties of the generated molecules. However,
the success of this approach is often hampered by the problem of sparse rewards as the majority of
the generated molecules are expectedly predicted as inactives. We propose several
technical innovations to address this problem and improve the balance between
exploration and exploitation modes in reinforcement learning. In a
proof-of-concept study, we demonstrate the application of the deep generative
recurrent neural network enhanced by several novel technical tricks to designing
experimentally validated potent inhibitors of the epidermal growth factor
(EGFR). The proposed technical solutions are expected to substantially improve
the success rate of finding novel bioactive compounds for specific biological targets
using generative and reinforcement learning approaches.
更多查看译文
AI 理解论文
溯源树
样例
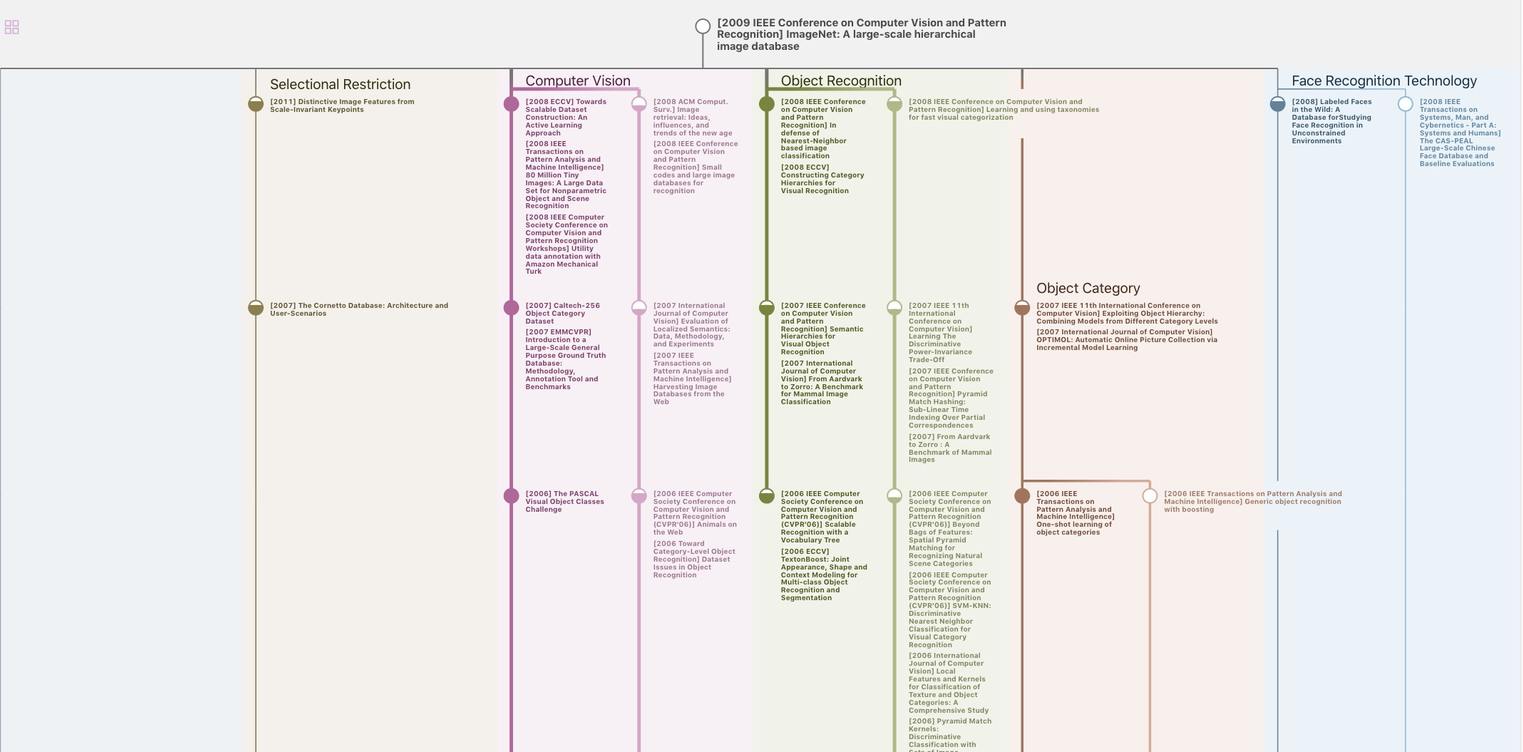
生成溯源树,研究论文发展脉络
Chat Paper
正在生成论文摘要