In silico prediction of chemical-induced hematotoxicity with machine learning and deep learning methods
MOLECULAR DIVERSITY(2021)
摘要
Chemical-induced hematotoxicity is an important concern in the drug discovery, since it can often be fatal when it happens. It is quite useful for us to give special attention to chemicals which can cause hematotoxicity. In the present study, we focused on in silico prediction of chemical-induced hematotoxicity with machine learning (ML) and deep learning (DL) methods. We collected a large data set contained 632 hematotoxic chemicals and 1525 approved drugs without hematotoxicity. Computational models were built using several different machine learning and deep learning algorithms integrated on the Online Chemical Modeling Environment (OCHEM). Based on the three best individual models, a consensus model was developed. It yielded the prediction accuracy of 0.83 and balanced accuracy of 0.77 on external validation. The consensus model and the best individual model developed with random forest regression and classification algorithm (RFR) and QNPR descriptors were made available at https://ochem.eu/article/135149 , respectively. The relevance of 8 commonly used molecular properties and chemical-induced hematotoxicity was also investigated. Several molecular properties have an obvious differentiating effect on chemical-induced hematotoxicity. Besides, 12 structural alerts responsible for chemical hematotoxicity were identified using frequency analysis of substructures from Klekota–Roth fingerprint. These results should provide meaningful knowledge and useful tools for hematotoxicity evaluation in drug discovery and environmental risk assessment. Graphic abstract
更多查看译文
关键词
Chemical-induced hematotoxicity,Machine learning,Deep learning,Consensus model,Structural alert
AI 理解论文
溯源树
样例
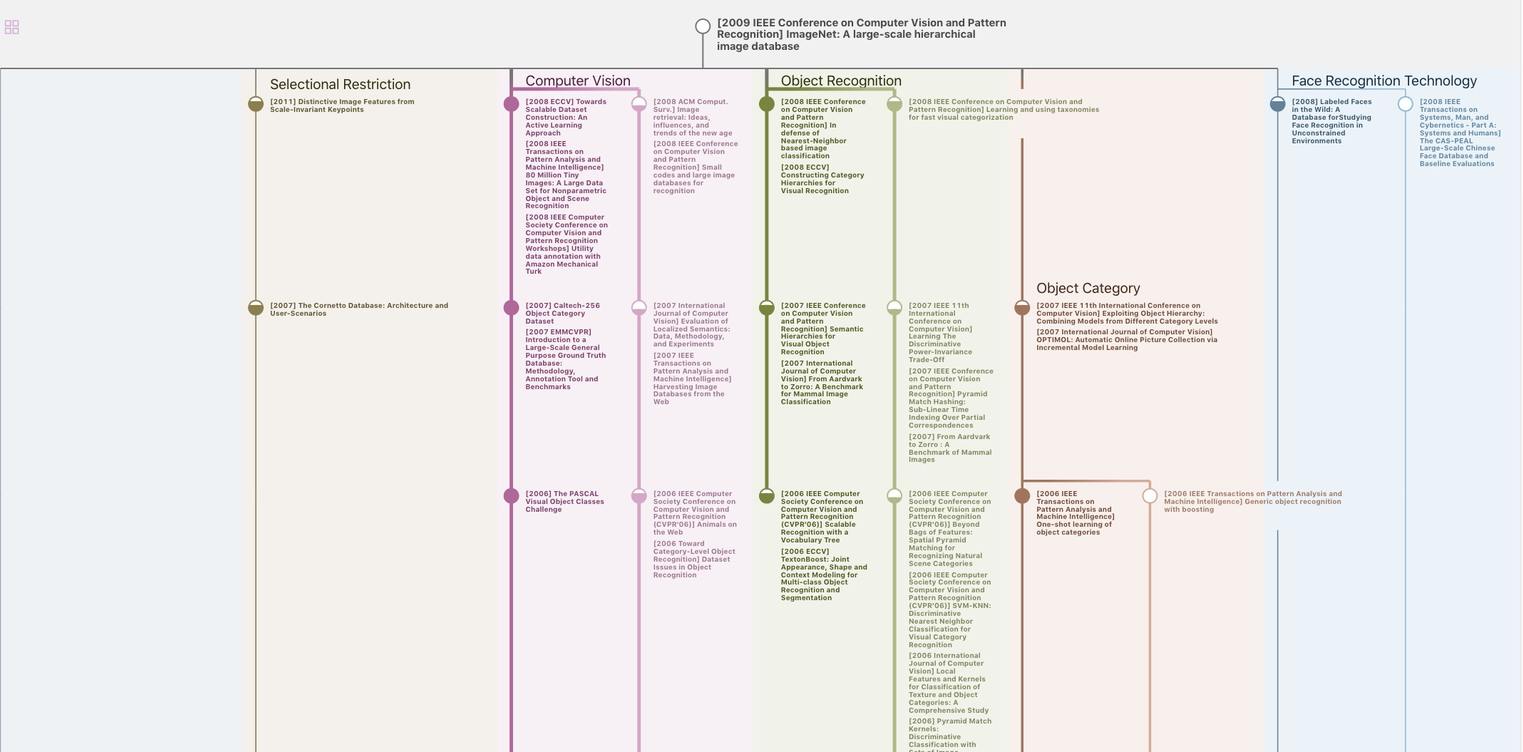
生成溯源树,研究论文发展脉络
Chat Paper
正在生成论文摘要