Short-Term Load Forecasting for Smart HomeAppliances with Sequence to Sequence Learning
ICC 2021 - IEEE International Conference on Communications(2021)
Abstract
Appliance-level load forecasting plays a critical role in residential energy management, besides having significant importance for ancillary services performed by the utilities. In this paper, we propose to use an LSTM-based sequence-to-sequence (seq2seq) learning model that can capture the load profiles of appliances. We use a real dataset collected fromfour residential buildings and compare our proposed schemewith three other techniques, namely VARMA, Dilated One Dimensional Convolutional Neural Network, and an LSTM model.The results show that the proposed LSTM-based seq2seq model outperforms other techniques in terms of prediction error in most cases.
MoreTranslated text
Key words
load forecasting,deep learning
AI Read Science
Must-Reading Tree
Example
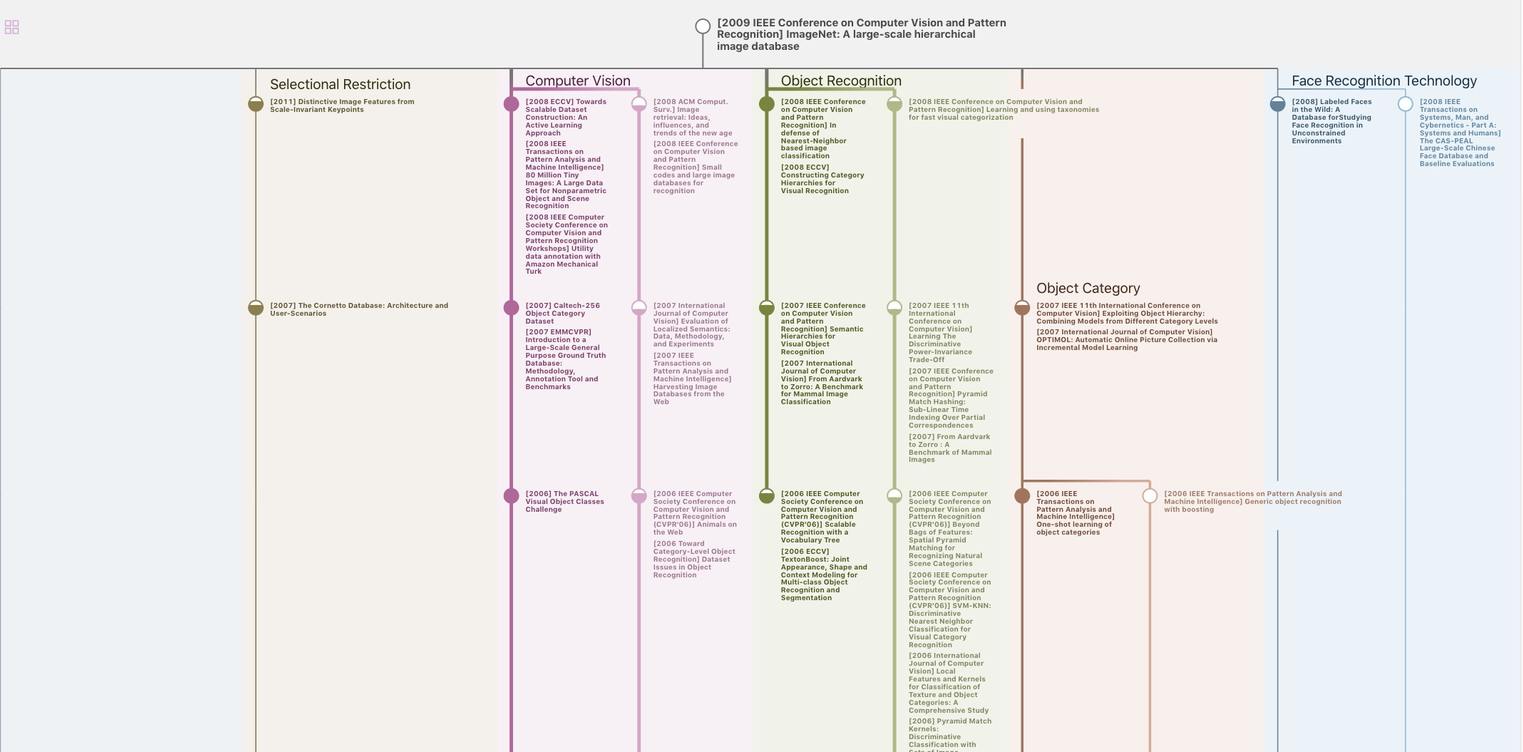
Generate MRT to find the research sequence of this paper
Chat Paper
Summary is being generated by the instructions you defined