Improving Protein Function Annotation via Unsupervised Pre-training: Robustness, Efficiency, and Insights
Knowledge Discovery and Data Mining(2021)
摘要
ABSTRACTRecent work demonstrated a large ensemble of convolutional neural networks (CNNs) outperforms industry-standard approaches at annotating protein sequences that are far from the training data. These results highlight the potential of deep learning to significantly advance protein sequence annotation, but this particular system is not a practical tool for many biologists because of the computational burden of making predictions using a large ensemble. In this work, we fine-tune a transformer model that is pre-trained on millions of unlabeled natural protein sequences in order to reduce the system's compute burden at prediction time and improve accuracy. By switching from a CNN to the pre-trained transformer, we lift performance from 73.6% to 90.5% using a single model on a challenging clustering-based train-test split, where the ensemble of 59 CNNs achieved 89.0%. Through extensive stratified analysis of model performance, we provide evidence that the new model's predictions are trustworthy, even in cases known to be challenging for prior methods. Finally, we provide a case study of the biological insight enabled by this approach.
更多查看译文
关键词
deep learning, bert, pfam, protein families, protein function annotation, bioinformatics, neural networks, unsupervised learning
AI 理解论文
溯源树
样例
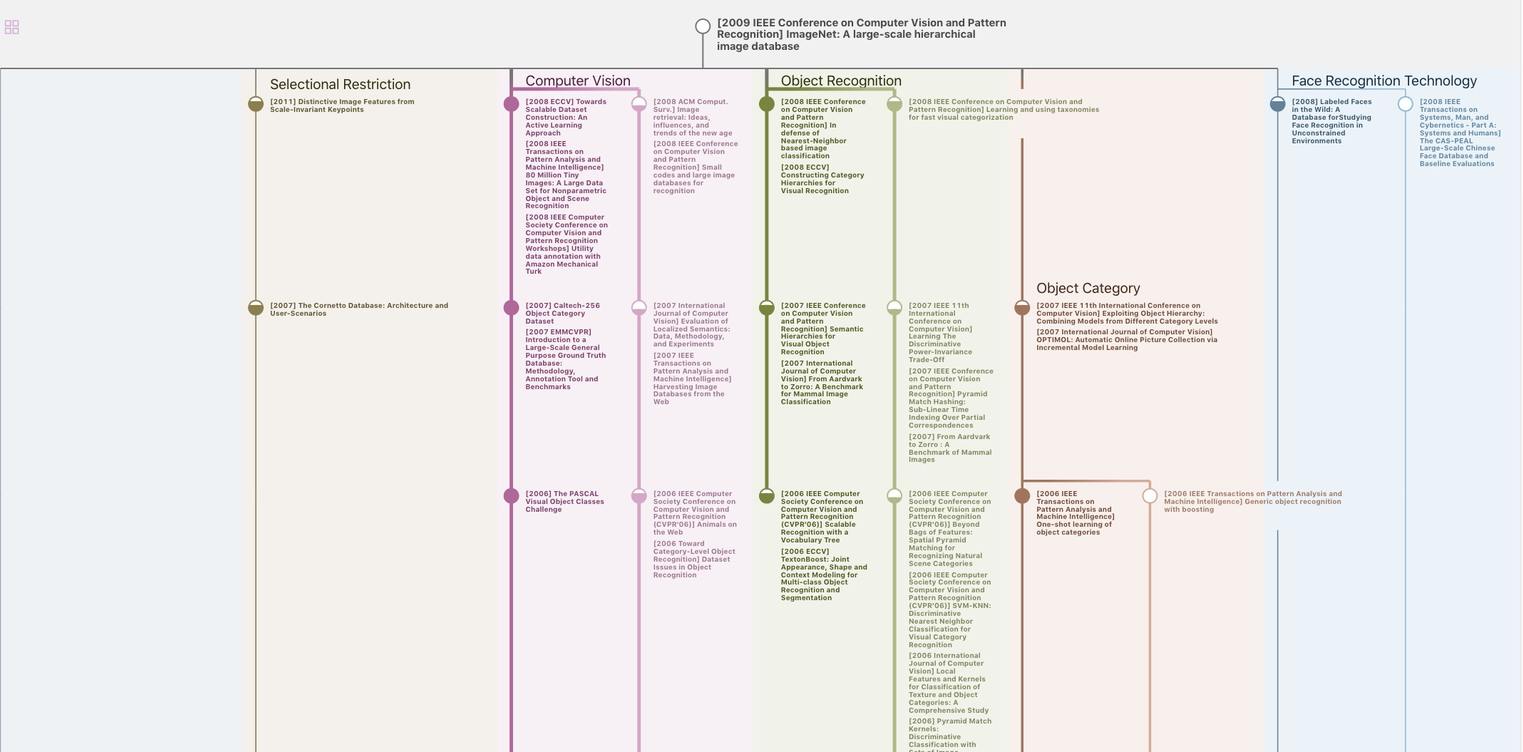
生成溯源树,研究论文发展脉络
Chat Paper
正在生成论文摘要