Physical Equation Discovery Using Physics-Consistent Neural Network (PCNN) Under Incomplete Observability
Knowledge Discovery and Data Mining(2021)
摘要
ABSTRACTDeep neural networks (DNNs) have been extensively applied to various fields, including physical-system monitoring and control. However, the requirement of a high confidence level in physical systems made system operators hard to trust black-box type DNNs. For example, while DNN can perform well at both training data and testing data, but when the physical system changes its operation points at a completely different range, never appeared in the history records, DNN can fail. To open the black box as much as possible, we propose a Physics-Consistent Neural Network (PCNN) for physical systems with the following properties: (1) PCNN can be shrunk to physical equations for sub-areas with full observability, (2) PCNN reduces unobservable areas into some virtual nodes, leading to a reduced network. Thus, for such a network, PCNN can also represent its underlying physical equation via a specifically designed deep-shallow hierarchy, and (3) PCNN is theoretically proved that the shallow NN in the PCNN is convex with respect to physical variables, leading to a set of convex optimizations to seek for the physics-consistent initial guess for the PCNN. We also develop a physical rule-based approach for initial guesses, significantly shortening the searching time for large systems. Comprehensive experiments on diversified systems are implemented to illustrate the outstanding performance of our PCNN.
更多查看译文
关键词
Physical System, Incomplete Observability, Physical Equation Discovery, Deep Neural Network, Convex Optimization
AI 理解论文
溯源树
样例
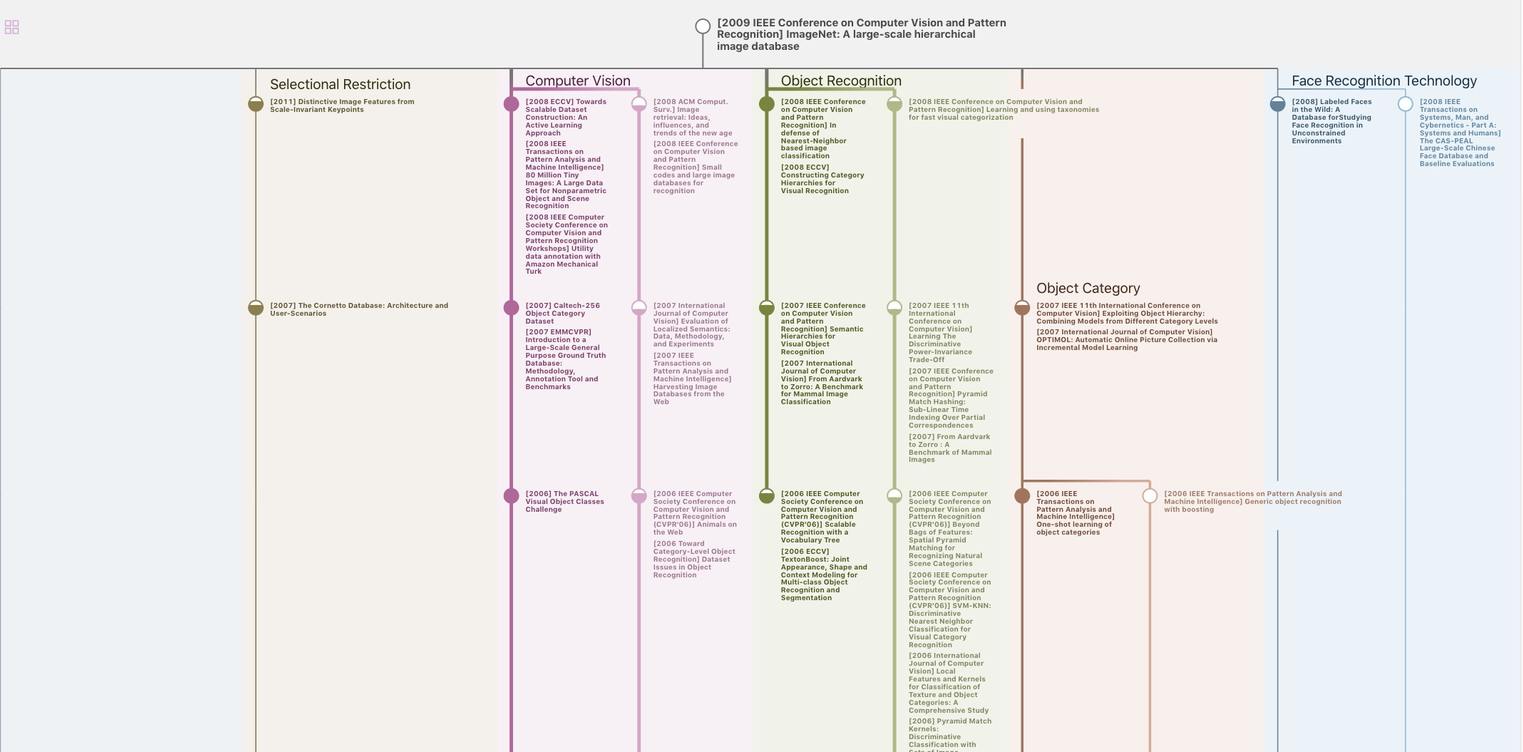
生成溯源树,研究论文发展脉络
Chat Paper
正在生成论文摘要