Stealthy Targeted Data Poisoning Attack On Knowledge Graphs
2021 IEEE 37TH INTERNATIONAL CONFERENCE ON DATA ENGINEERING (ICDE 2021)(2021)
Abstract
A host of different KG embedding techniques have emerged recently and have been empirically shown to be very effective in accurately predicting missing facts in a KG, thus improving its coverage and quality. Unfortunately, embedding techniques can fall prey to adversarial data poisoning attack. In this form of attack, facts may be added to or deleted from a KG, called performing perturbations, that results in the manipulation of the plausibility of target facts in a KG. While recent works confirm this intuition, the attacks considered there ignore the risk of exposure. Intuitively, an attack is of limited value if it is highly likely to be caught, i.e., exposed. To address this, we introduce a notion of the exposure risk and propose a novel problem of attacking a KG by means of perturbations where the goal is to maximize the manipulation of the target fact's plausibility while keeping the risk of exposure under a given budget. We design a deep reinforcement learning-based framework, called RATA, that learns to use low-risk perturbations without compromising on the performance, i.e., manipulation of target fact plausibility. We test the performance of RATA against recently proposed strategies for KG attacks, on two different benchmark datasets and on different kinds of target facts. Our experiments show that RATA achieves state-of-the-art performance even while using a fraction of the risk.
MoreTranslated text
Key words
exposure risk,low-risk perturbations,KG attacks,benchmark datasets,stealthy targeted data poisoning attack,knowledge graphs,embedding techniques,adversarial data poisoning attack,deep reinforcement learning,RATA
AI Read Science
Must-Reading Tree
Example
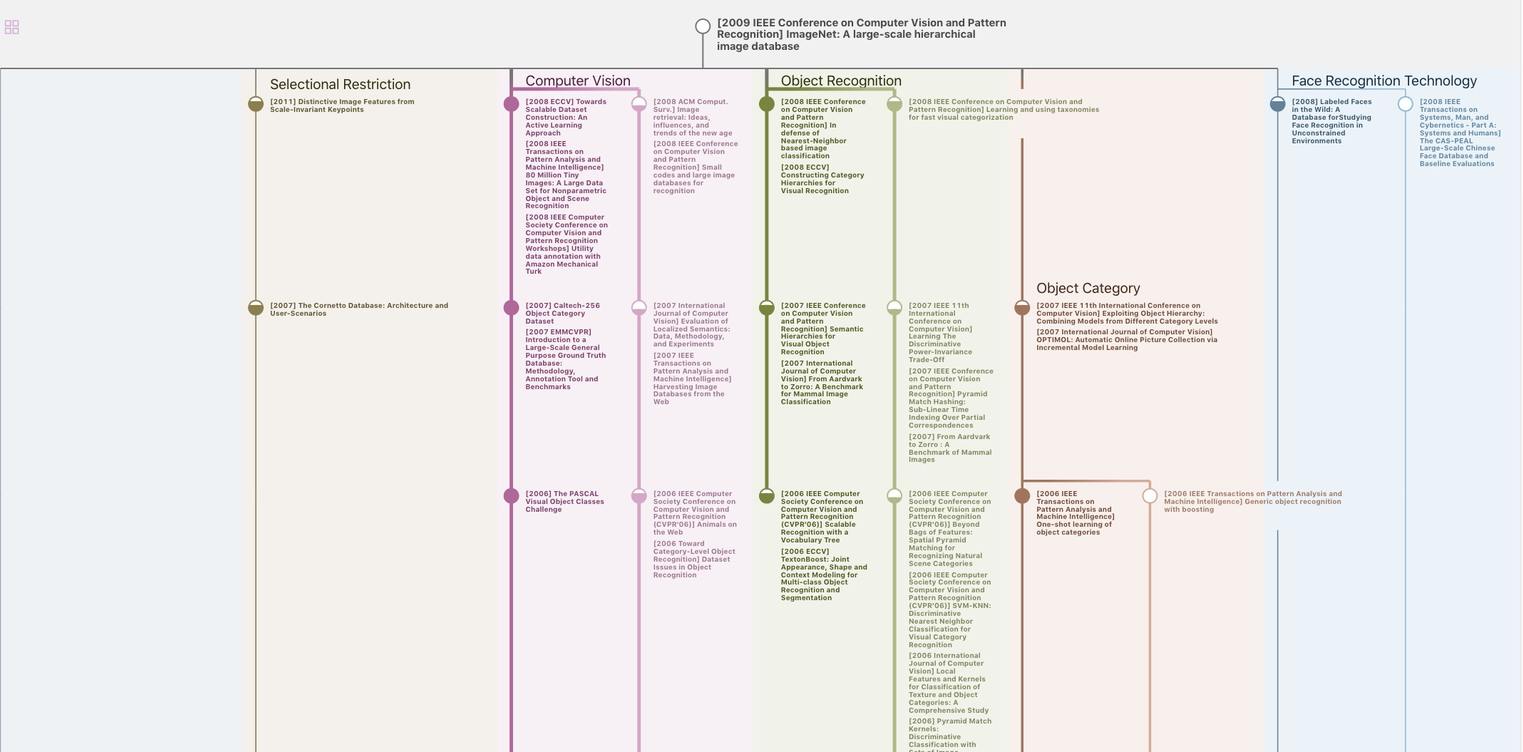
Generate MRT to find the research sequence of this paper
Chat Paper
Summary is being generated by the instructions you defined